FDFF-Net: A Full-Scale Difference Feature Fusion Network for Change Detection in High-Resolution Remote Sensing Images
IEEE JOURNAL OF SELECTED TOPICS IN APPLIED EARTH OBSERVATIONS AND REMOTE SENSING(2024)
摘要
Deep-learning techniques have made significant advances in remote sensing change detection task. However, it remains a great challenge to detect the details of changed areas from high-resolution remote sensing images. In this study, we propose a full-scale difference feature fusion network (FDFF-Net) for change detection, which can alleviate pseudochanges and reduce the loss of change details during detection. In the encoding stage, a dense difference fusion module is proposed to effectively mine and fuse the multiple differences for each feature level between bitemporal images, leading to a substantial reduction in missed detection of change areas. Additionally, the different levels of difference features are aggregated through a full-scale skip connection, allowing the network to detect multiple changed objects with various sizes. In the decoding stage, a strip spatial attention module is designed to enhance the perception of the change areas, which improves the ability to detect detailed changes. The experiments on three change detection datasets, CDD, LEVIR-CD, and S2Looking, demonstrate that FDFF-Net outperforms the compared state-of-the-art methods and can detect more complete changes of small objects and clear contours of changed areas.
更多查看译文
关键词
Attention mechanism,change detection,deep learning,difference feature fusion
AI 理解论文
溯源树
样例
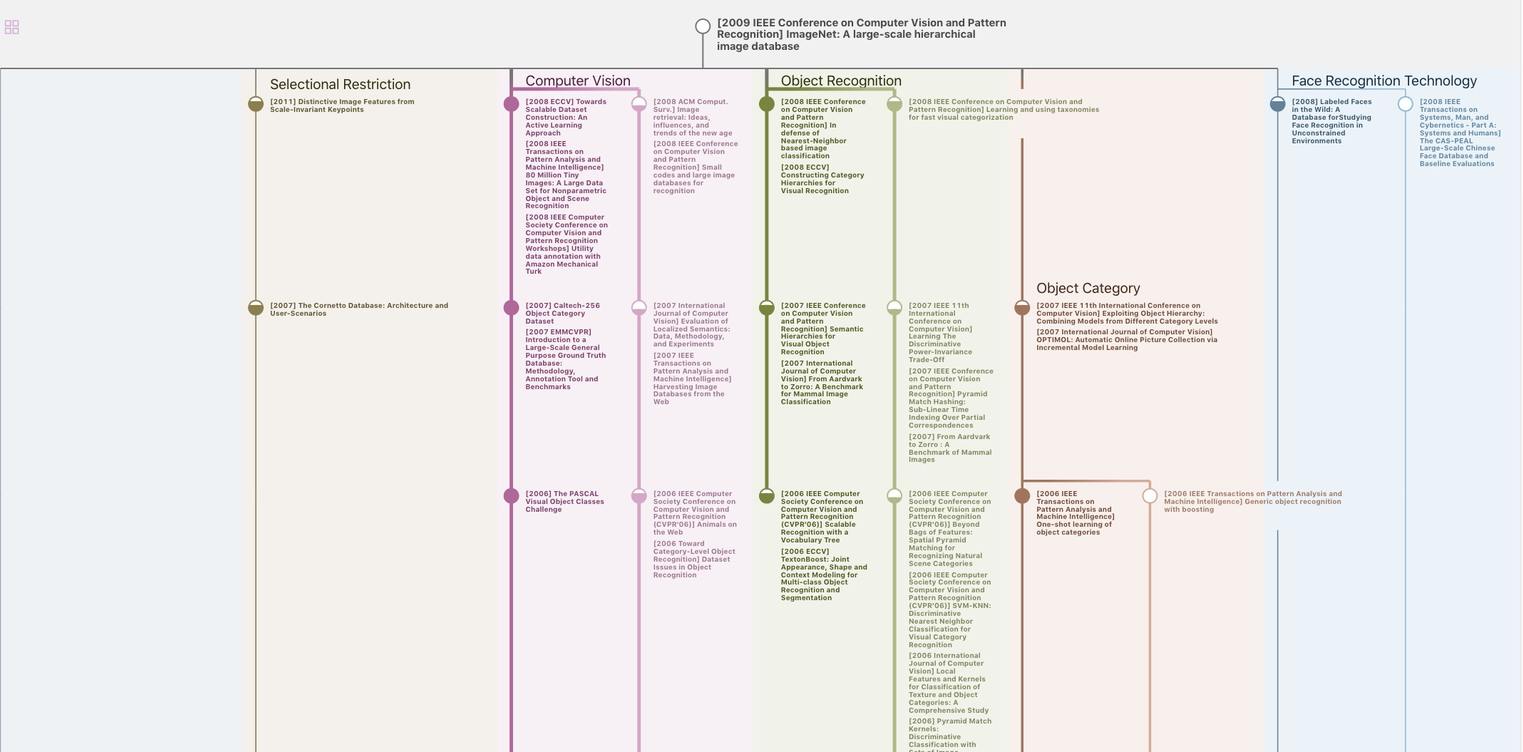
生成溯源树,研究论文发展脉络
Chat Paper
正在生成论文摘要