On the Choice of Unique Identifiers for Predicting Pareto-Optimal Solutions Using Machine Learning.
2023 IEEE Symposium Series on Computational Intelligence (SSCI)(2023)
摘要
Incomplete or sparse non-dominated fronts are unavoidable in multi-objective optimization due to complexity of problems, morphology of Pareto optimal fronts, and stochasticity involved in evolutionary optimization algorithms. It is pragmatic to develop methods that can alleviate some of these issues after the optimization run is complete, without the need for re-optimization or additional solution evaluations. Previously developed methods demonstrated that it is possible to predict Pareto-optimal solutions from pseudo-weight vectors using Gaussian Process Regression (GPR) models. We extend the GPR-based method to predict new Pareto-optimal solutions using reference vectors as unique identifiers and demonstrate that like the pseudo-weight vectors, reference vectors can also used instead in learning the association between identifiers and corresponding variable vectors. Results on many test problems indicate that the choice of a suitable identifier makes a large impact on the decision-making process, particularly for visualizing the newly created non-dominated (ND) solutions. In this study, we discuss the advantages and disadvantages of using pseudo-weights and reference vectors as unique identifiers for ND solutions, paving the way to devise further identifiers for predicting new Pareto-optimal solutions.
更多查看译文
关键词
machine-learning,multi-objective optimization,optimization,evolutionary algorithm,multi-criterion decision making
AI 理解论文
溯源树
样例
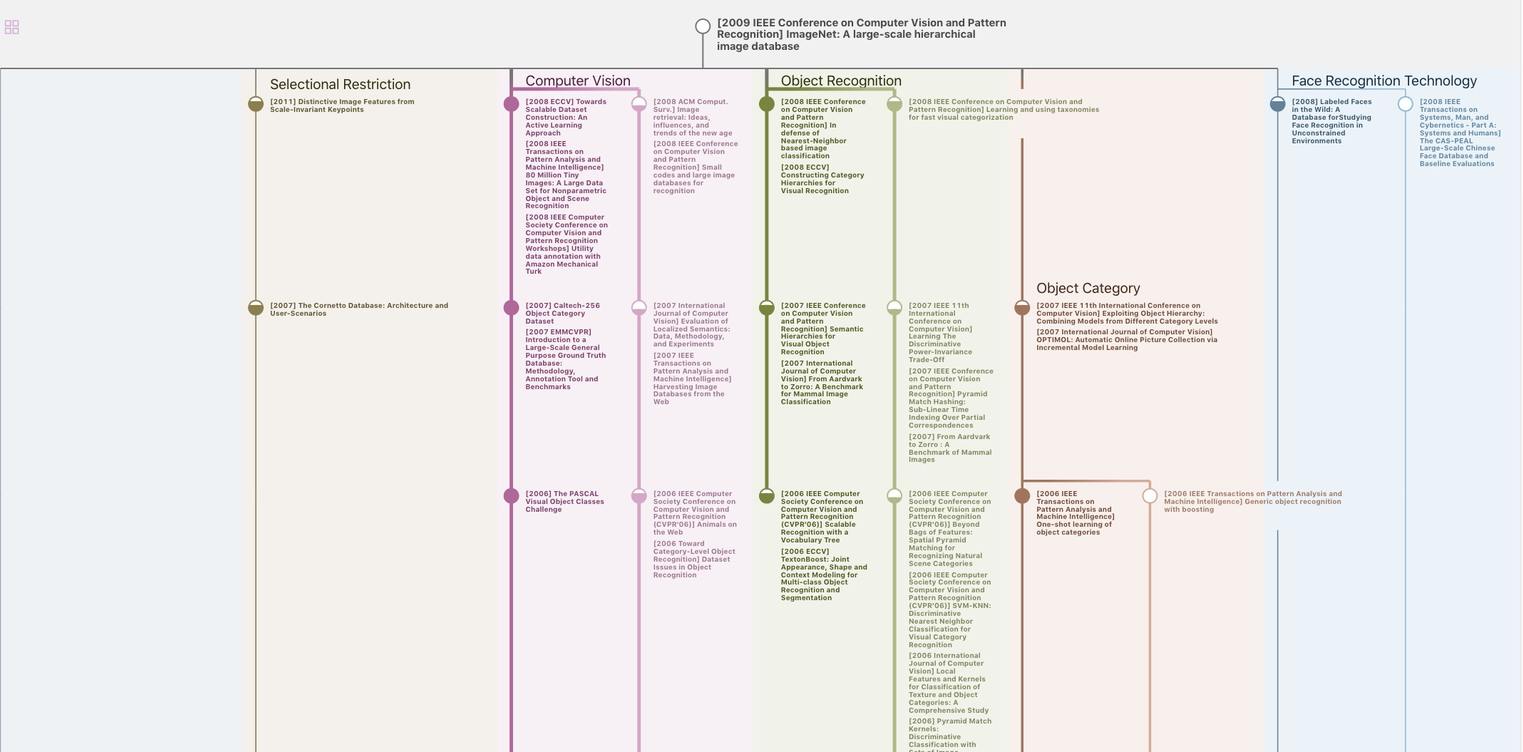
生成溯源树,研究论文发展脉络
Chat Paper
正在生成论文摘要