Fault Identification of Discrete-time unknown Nonlinear Systems: A Two-dimensional Convolutional Neural Network Approach.
2023 IEEE Symposium Series on Computational Intelligence (SSCI)(2023)
摘要
A complex system that is governed by several smaller sub-systems whose coordinated functionality allows it to work properly over time can be challenging to analyze for faults on real time by an observer; moreover, if such failing system could work with no obvious signs of fault over time until it becomes catastrophic and clearly identifiable. Because the variables involved in such system's functionality are usually not easily correlated, the different time-series they might generate can be extremely difficult to analyze by conventional means. Lately, 2-dimensional Convoluted Neural Networks (2D-CNN) have been used to introduce artificial intelligence into diagnosis and fault detection with success; however, the systems that so far have benefited from this are mainly those that deal with images, like medical diagnosis using x-ray images, or autonomous driving using real time pictures, although recently, some resent research on robotic sensor fault and signal analysis have been published using 1-dimensional CNN (1D-CNN) for time-domain signals. This paper proposes a novel 2D-CNN approach to fault identification of an unknown, discrete-time, nonlinear system; by recognizing features that are consistent with a fault in a signal-image of several layers. With such signal-image being an artificial picture created by combining all the system signals in a single high-layered image format that is recognizable by a conventional 2D-CNN. This paper also includes the results of its applicability in a fault identification of a three-phase induction motor in a simulation environment and with measurements of a real motor with injected faults.
更多查看译文
关键词
deep learning,fault identification,fault prediction,applied artificial intelligence. Convolutional neural networks nonlinear systems
AI 理解论文
溯源树
样例
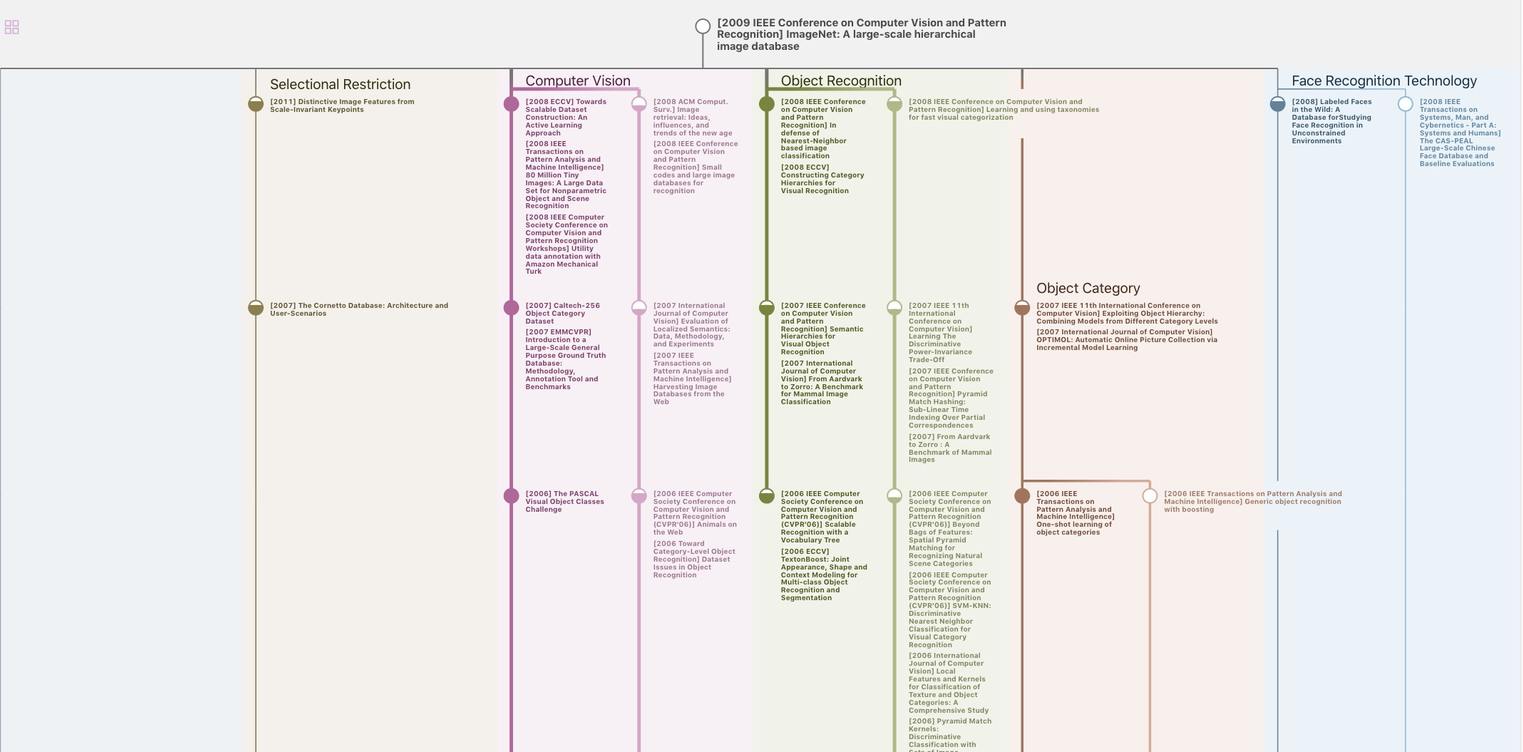
生成溯源树,研究论文发展脉络
Chat Paper
正在生成论文摘要