Integration of Structural Equation Models and Bayesian Networks for Cognitive Load Modeling.
2023 IEEE Symposium Series on Computational Intelligence (SSCI)(2023)
摘要
This study offers a causal probabilistic modeling for inferring the relationship between humans' cognitive load, the physiological signal predictors of such load, and personality traits. We selected a subset of such signals (heart rate, intervals between successive heartbeats, galvanic skin response, and temperature) from the CogLoad dataset using wearable devices. Structural Equation Modeling techniques were employed to select the predictors to identify the level of cognitive load, for which the ground truth was assessed using subjective tests such as HEXACO that determine the personality traits of the human subjects. Bayesian networks were deployed to investigate the causal relationship and model the inference scenarios. The proposed model is intended to contribute to developing a Computational Intelligence tool for monitoring social health in scenarios of future potential crises such as pandemics and mass migration,
更多查看译文
关键词
cognitive load,physiological response,personality traits,emotions,machine reasoning,mental workload,probabilistic inference,Bayesian network,structural equation modelling
AI 理解论文
溯源树
样例
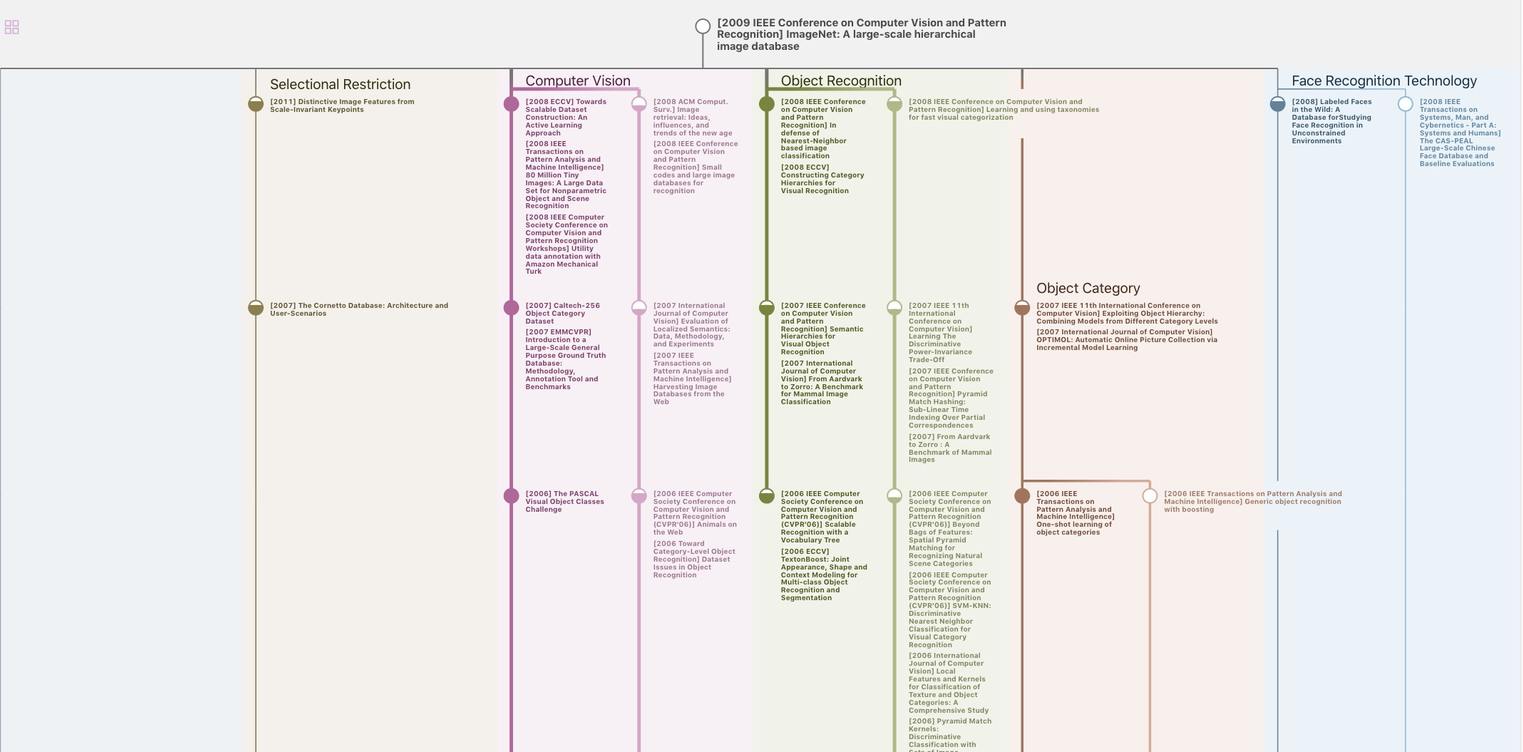
生成溯源树,研究论文发展脉络
Chat Paper
正在生成论文摘要