What Drives Evolution of Self-Driving Automata?
2023 IEEE Symposium Series on Computational Intelligence (SSCI)(2023)
摘要
Self-Driving Automata (SDAs) are variations on finite automata that both read and output symbols. They are versatile and practical when used for the generation of data for a variety of problems. In this study, we examine several questions regarding their operation, using sequence matching as a test problem in the analysis. We present a new mutation operator and four dynamic mutation adjusters. We analyze these, along with crossover, for their ability to solve the problem and their relative ability to improve the population; in all of these, we also examine population diversity over time. We find that using mutation that implements a static quantity of changes outperforms one with dynamic changes. Further, while population diversity does decrease somewhat, evolution is still possible.
更多查看译文
关键词
evolutionary algorithm,self-driving automata,sequence matching,mutation,diversity
AI 理解论文
溯源树
样例
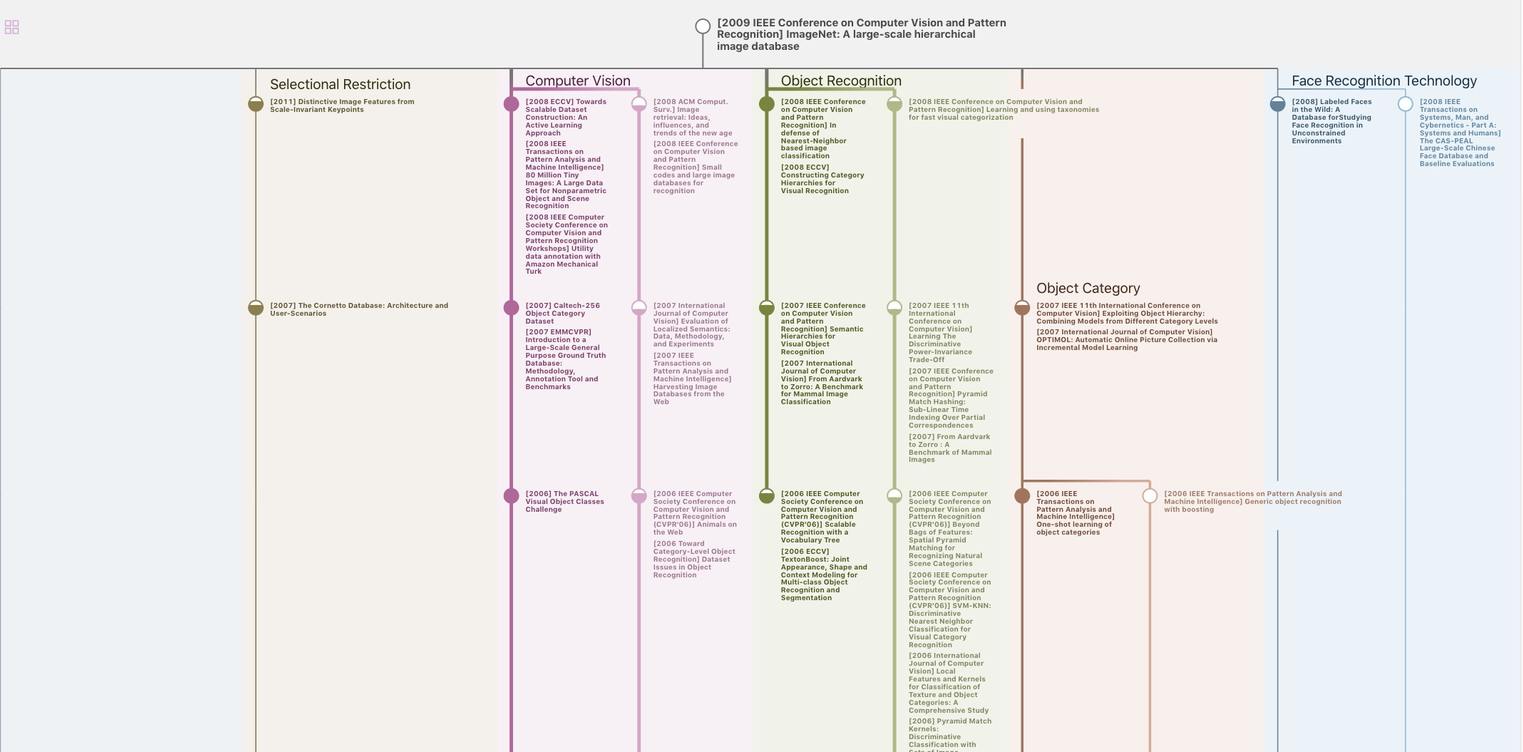
生成溯源树,研究论文发展脉络
Chat Paper
正在生成论文摘要