A Survey on Bias Mitigation in Federated Learning.
2023 IEEE Symposium Series on Computational Intelligence (SSCI)(2023)
摘要
Federated learning (FL) enables collaborative model training while keeping data decentralized. However, system heterogeneity and statistical differences in decentralized data can introduce biases and unfairness. This paper surveys existing bias mitigation techniques in FL across various phases of the training process. We identify sources of bias and present a critical analysis of current fairness-aware FL algorithms, categorizing them as preventive (Pre-processing) or reactive (in-processing and Post-processing) based on when bias mitigation is applied. In addition, this paper reveals open challenges in balancing fairness and efficiency in FL, handling non-independent and identically distributed (non-IID) data, and ensuring privacy. This survey lays out the foundation for developing unbiased and privacy-preserving FL systems without discrimination in the future.
更多查看译文
关键词
Federated Learning,system heterogeneity,Bias mitigation,decentralized data,fairness
AI 理解论文
溯源树
样例
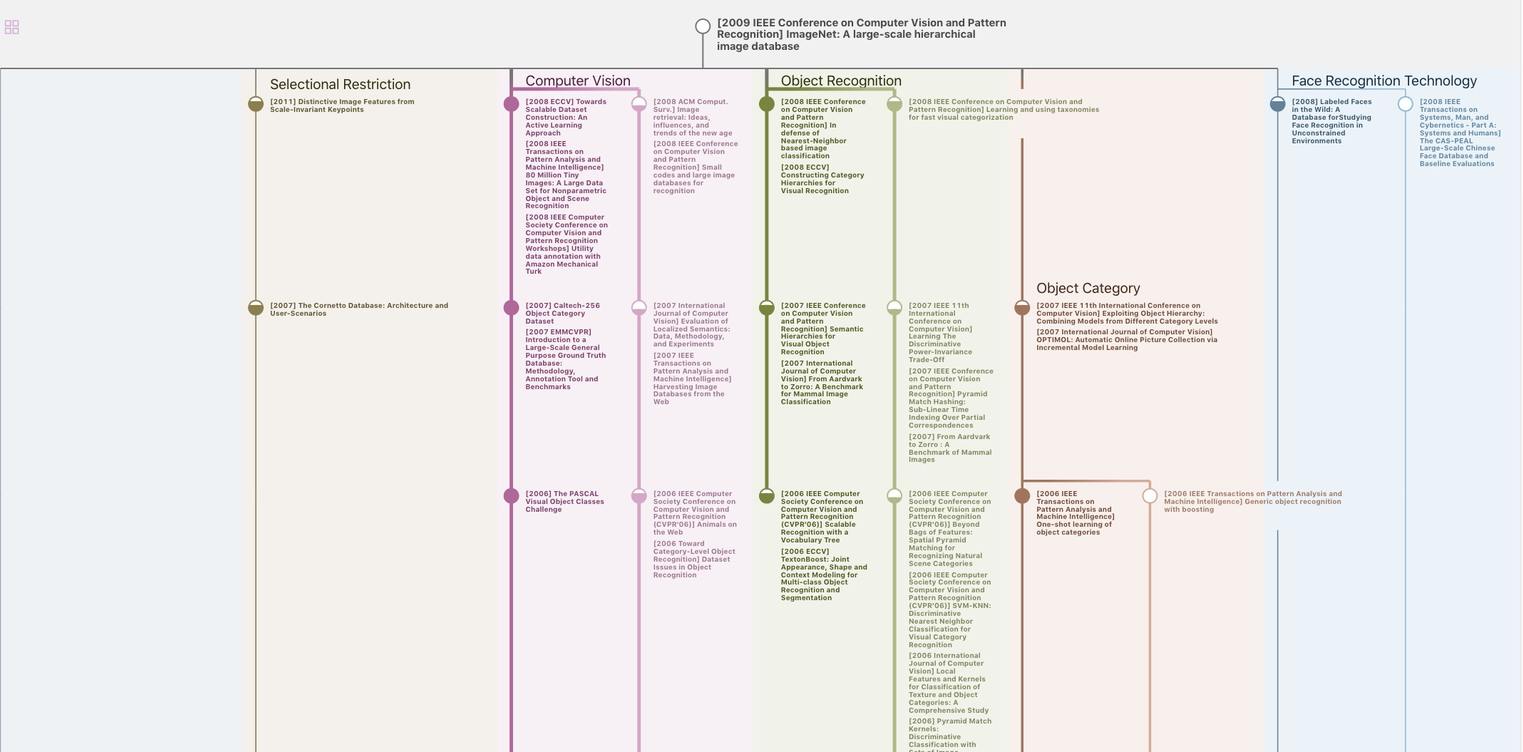
生成溯源树,研究论文发展脉络
Chat Paper
正在生成论文摘要