BLB-GAFS: An Efficient, Multi-Objective Genetic Algorithm Based Feature Selection Method for Intrusion Detection Systems.
2023 IEEE Symposium Series on Computational Intelligence (SSCI)(2023)
摘要
Protecting Internet of Things (IoT) networks from threats is becoming increasingly important as these devices continue to grow in adoption. Modern and unseen attacks that require the analysis of more complex network traffic data for effective identification and mitigation are becoming more prevalent. Traditional machine learning approaches in current intrusion detection systems (IDS) struggle with these volumes of data, prompting exploration into the feature selection space. One class of such feature selection methods is evolutionary algorithms, in which systems mimicking real-life evolution optimize solutions for some problem. In this paper, we propose bag-of-little-bootstraps genetic algorithm feature selection (BLB-GAFS), a novel variant of the genetic algorithm feature selection method that maintains a global search of the solution space while reducing computational cost. This is accomplished with the bag-of-little-bootstraps method for approximating classifier performance. We test the BLB-GAFS technique on three modern intrusion datasets-CCD-INID-V1, detection_of_IoT_botnet_attacks_N_BaIoT, and CIRA-CIC-DoHBrw-2020-that represent updated network patterns and are highly dimensional. We found that the BLB-GAFS method matches or outperforms embedded feature selection methods on the same datasets. Furthermore, the feature sets selected by BLB-GAFS result in significantly improved multiclass precision, recall, and F1-score. Traditionally expensive wrapper feature selection methods like the genetic algorithm can be used on larger datasets through BLB-GAFS, opening the door to other applications with highly dimensional data.
更多查看译文
关键词
feature selection,genetic algorithm,IDS,bag-of-little-bootstraps
AI 理解论文
溯源树
样例
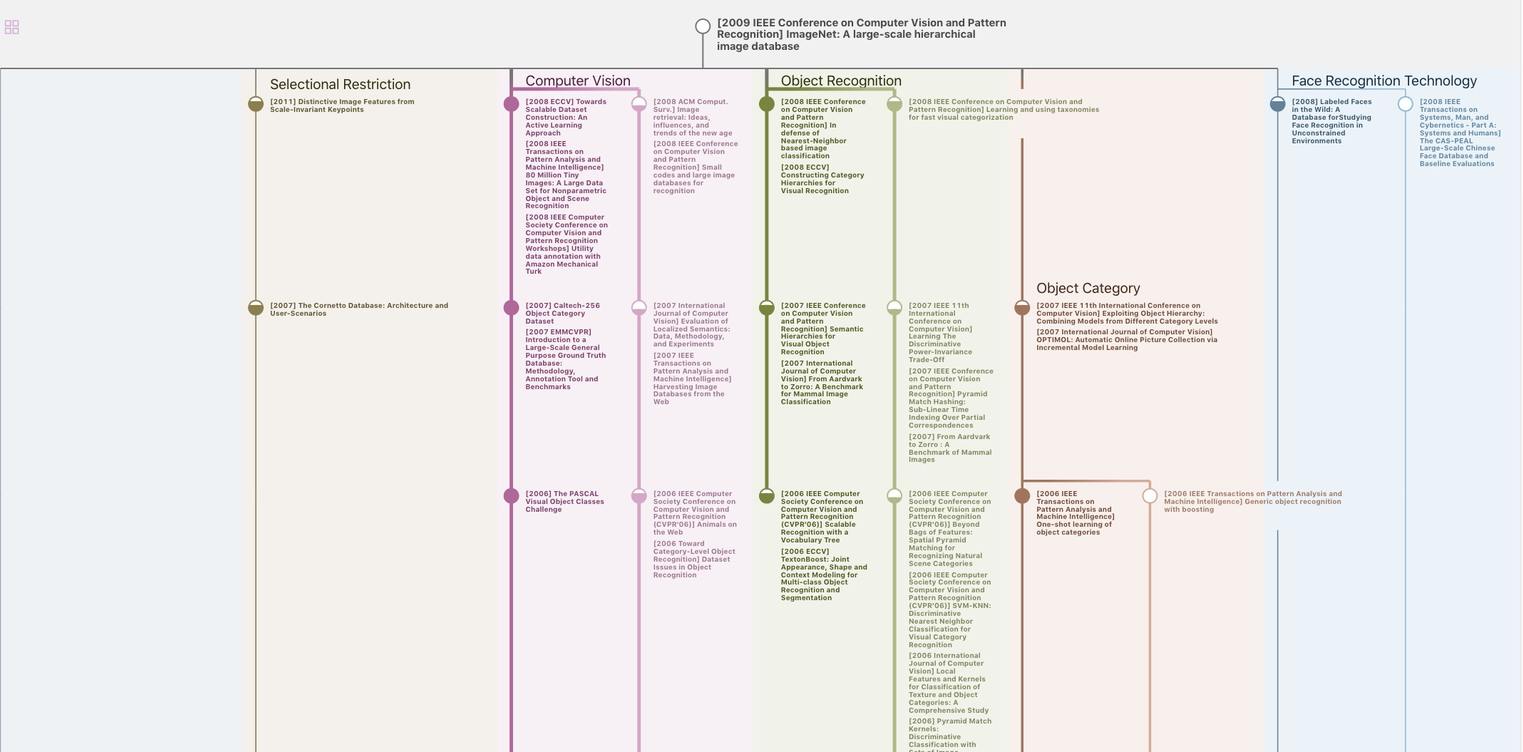
生成溯源树,研究论文发展脉络
Chat Paper
正在生成论文摘要