DFairNAS: A Dataflow Fairness Approach to Training NAS Neural Networks.
2023 16th International Congress on Image and Signal Processing, BioMedical Engineering and Informatics (CISP-BMEI)(2023)
摘要
Neural Architecture Search (NAS) is a technology that finds the best neural architecture from a large number of candidates. Recent researchers have proposed one-shot NAS for reducing search costs. One-shot NAS organizes a search space into a single large-scale network (i.e., supernet) and trains it as an estimator of the neural network candidates (i.e., subnetworks). However, one-shot NAS still faces an unfairness training challenge—the supernet can still have poor estimation performance, as it may be unfairly trained, especially when the search space is irregular. To tackle with this challenge, we propose DFairNAS, a dataflow fairness approach to fairly training supernets in general search spaces. DFairNAS trains supernets while keeping dataflow fairness; it improves the estimation performance of a supernet by organizing its operators into layers and requires all of the dataflows between two adjacent layers to be fairly trained. We evaluate DFairNAS against existing one-shot NAS approaches on the CIFAR datasets. The evaluation results show that DFairNAS outperforms FAIRNAS (a state-of-the-art operator fairness-based NAS approach) by 63.19% and 73.99% in the supernets’ estimation performance in regular and irregular search spaces, respectively; it also outperforms FAIRNAS by 70.3% on F-MATRIC, a novel metric for measuring training fairness. Furthermore, DFairNAS can find architectures with high accuracy without incurring heavy training costs.
更多查看译文
关键词
Neural architecture search,One-shot training,Fairness
AI 理解论文
溯源树
样例
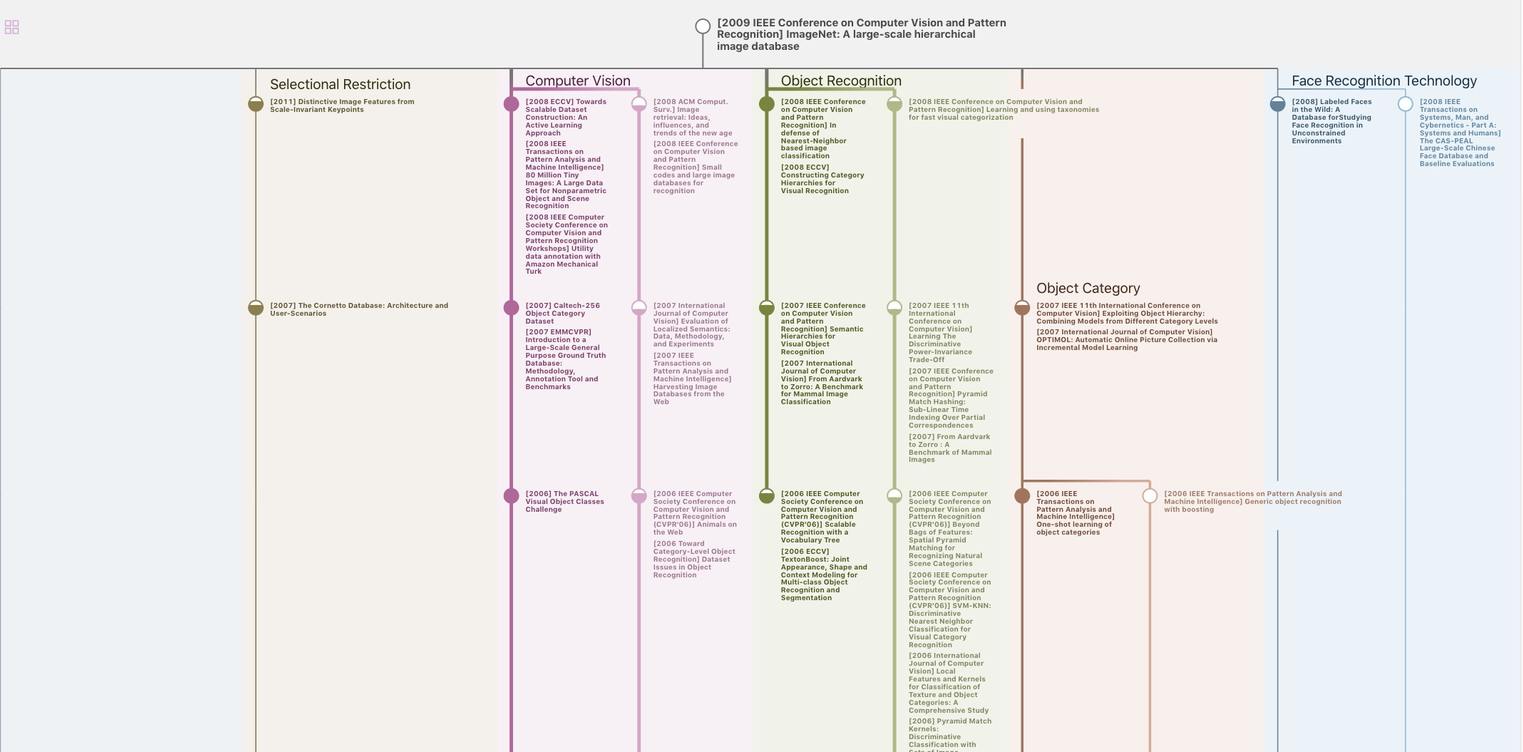
生成溯源树,研究论文发展脉络
Chat Paper
正在生成论文摘要