Deep Learning Approaches for Vessel Estimated Time of Arrival Prediction: A Case Study on the Saint Lawrence River.
2023 14th International Conference on Intelligent Systems: Theories and Applications (SITA)(2023)
摘要
Improving the planning and management of maritime operations is a key element in driving the maritime supply chain. Although technological innovations facilitate the management of maritime operations, they are still affected by disruptions and uncertainties related to the need for more reliable information. One of the most crucial pieces of information for steering the maritime supply chain is the estimated time of arrival (ETA) of vessels at their destination, as accurate ETA predictions can improve the determination of optimal vessel routes, the monitoring of maritime traffic and the planning of port operations.In this context, we propose to leverage three years of historical vessel trip data. The objective of this work is to present an approach based on deep learning models to predict the ETA of vessels in the Saint Lawrence River. The models were trained on different data sources, taking into account various types and characteristics of vessels and their respective itineraries. This study highlights the potential of deep learning-based methods to leverage maritime historical data to predict ETA, providing a valuable solution for managing maritime supply chains.
更多查看译文
关键词
estimated time of arrival,deep learning,Saint Lawrence River,maritime supply chain
AI 理解论文
溯源树
样例
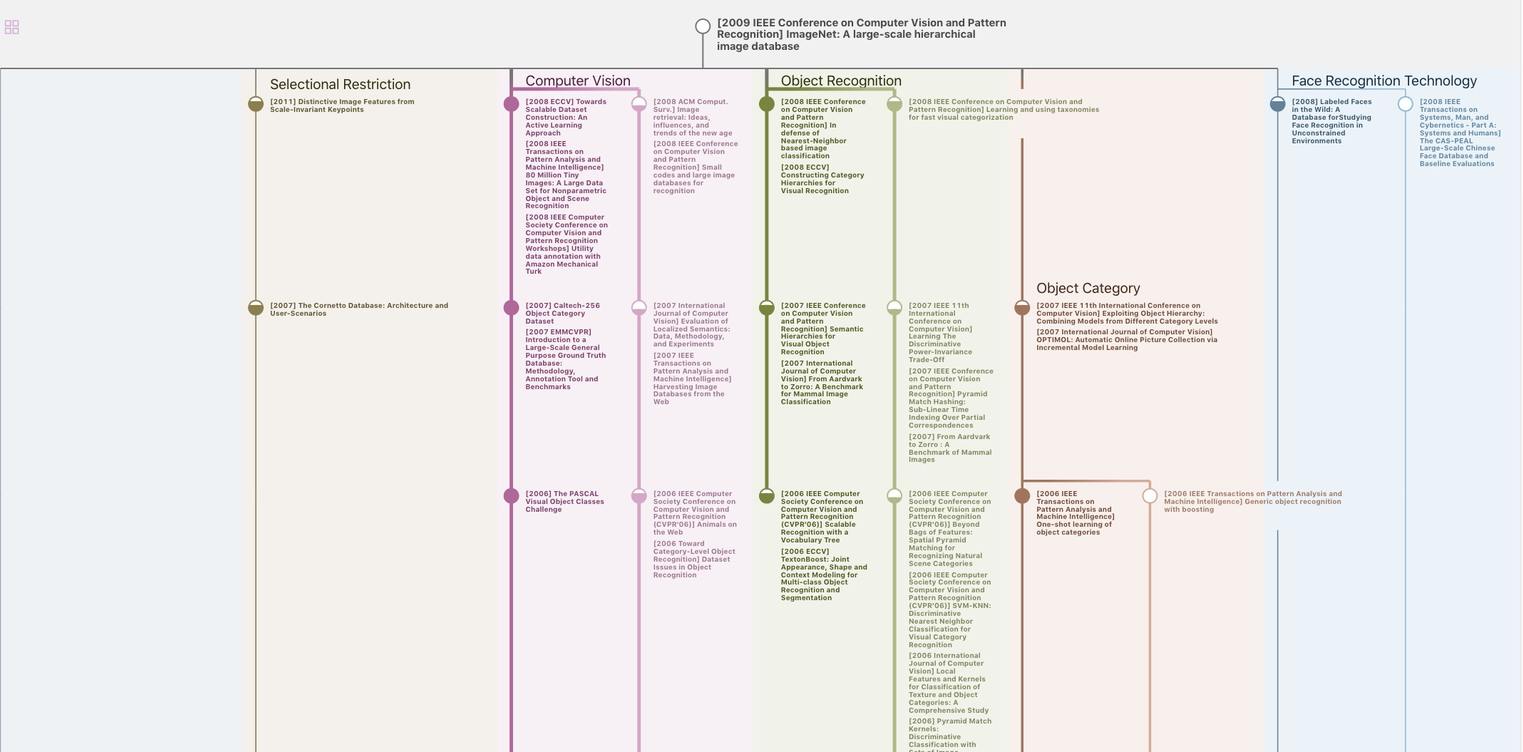
生成溯源树,研究论文发展脉络
Chat Paper
正在生成论文摘要