Transfer Learning Techniques for the Lithium-Ion Battery State of Charge Estimation
IEEE ACCESS(2024)
摘要
State of Charge (SOC) estimation is vital for battery management systems (BMS), impacting battery efficiency and lifespan. Accurate SOC estimation is challenging due to battery complexity and limited data for training Machine Learning based models. Transfer learning (TL) leverages pre-trained models, reducing training time and improving generalization in SOC estimation. In this paper, 8 different transfer learning techniques are examined, which were applied in four different models (LSTM, GRU, BiLSTM, and BiGRU) for SOC estimation. These transfer learning techniques have been applied to three datasets for re-training the models and results have been compared with the same models defined by Bayesian Hyperparameter Optimization. The TL4 and TL5 techniques consistently stood out as among the most efficient in both accuracy and computational time.
更多查看译文
关键词
Transfer learning,lithium-ion battery,machine learning,state of charge
AI 理解论文
溯源树
样例
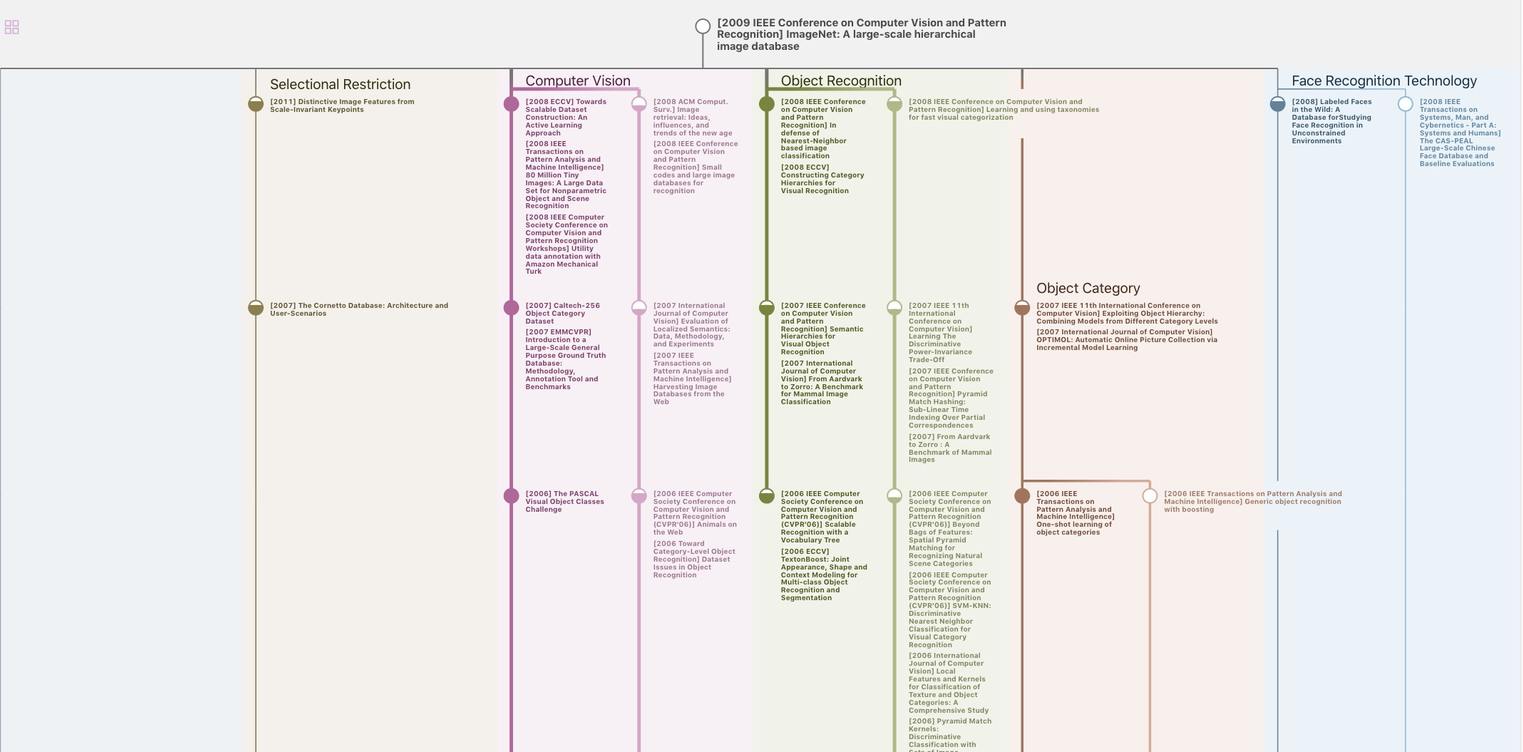
生成溯源树,研究论文发展脉络
Chat Paper
正在生成论文摘要