MSR U-Net: An Improved U-Net Model for Retinal Blood Vessel Segmentation
IEEE ACCESS(2024)
摘要
For the proper diagnosis and treatment of a variety of retinal conditions, retinal blood vessel segmentation is crucial. Delineation of vessels with varying thicknesses is critical for detecting disease symptoms. However, this task is challenging due to inadequate contextual information, complex vessel morphology, and lesion confusion. Many recent works employed several variations of CNNs with U-Net as baseline model for segmenting blood vessels from the fundus images. However, the existing methods still lack in generalizing the vessels well enough, indicating scope for improvement in this challenging problem of vessel segmentation. We introduce a novel Multi-Scale Residual (MSR) U-Net model in this study replacing convolution block and skip connections with an improved Multi-Scale Residual (MSR) convolution block and Bottleneck residual paths (B-Res paths) respectively. Specifically, STARE, DRIVE, and CHASE_DB1 datasets of fundus images are used to validate the proposed segmentation method. Our experimental results consistently showcase better/comparable performances when compared with current approaches, achieving higher area under receiver operator characteristic (AUC), accuracy, and F1 score. in segmenting blood vessels of varying thicknesses, even in scenarios with diverse contextual information, the presence of coexisting lesions, and complex vessel morphologies.
更多查看译文
关键词
Image segmentation,Biomedical imaging,Blood vessels,Retina,Feature extraction,Convolutional neural networks,Lesions,Artificial intelligence,fundus,retina,segmentation,U-Net
AI 理解论文
溯源树
样例
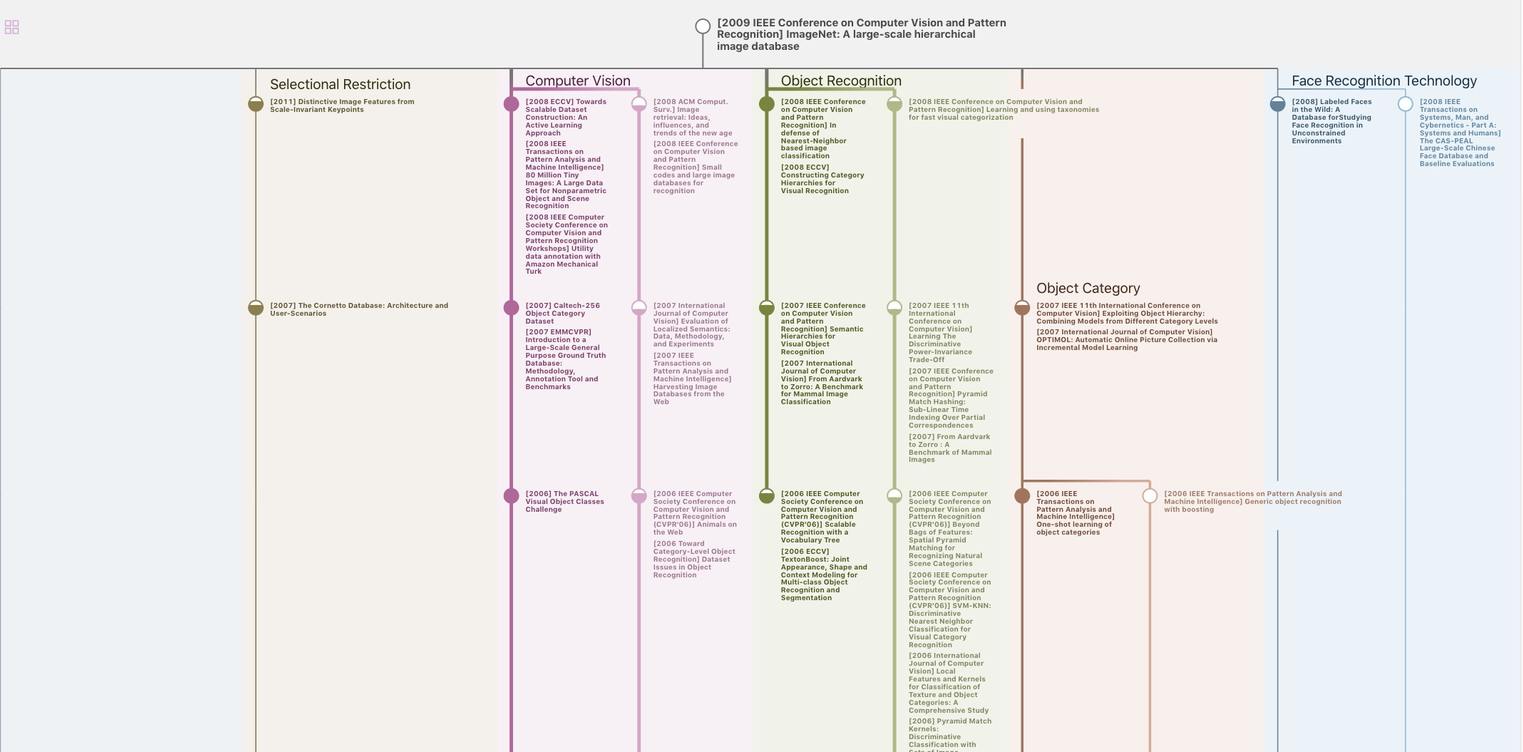
生成溯源树,研究论文发展脉络
Chat Paper
正在生成论文摘要