A Graph Neural Networks-Based Learning Framework With Hyperbolic Embedding for Personalized Tag Recommendation
IEEE ACCESS(2024)
摘要
Learning high-quality representations of users, items, and tags from historical interactive data is crucial for personalized tag recommendation (PTR) systems. Currently, most PTR models are committed to learning representations from first-order interactions without considering the exploitation of high-order interactive relations, which can be beneficial for avoiding sub-optimal learning. Although several PTR models equipped with graph neural networks (GNN) have been proposed to capture higher-order semantic relevance from raw data, they all carry out representation learning in Euclidean space, which can still easily result in sub-optimal learning due to embedding distortion. In order to further improve the quality of representation learning for PTR, the paper proposes a novel PTR model based on a lightweight GNN framework with hyperbolic embedding, namely GHPTR. GHPTR explicitly injects higher-order relevance into entity representation through the message propagation and aggregation mechanism of GNN and leverages hyperbolic embedding to alleviate the embedding distortion problem. Experimental results on real-world datasets have demonstrated the superiority of our model over its Euclidean counterparts and state-of-the-art baselines.
更多查看译文
关键词
Tag recommendation,graph neural networks,hyperbolic geometry,representation learning,embedding
AI 理解论文
溯源树
样例
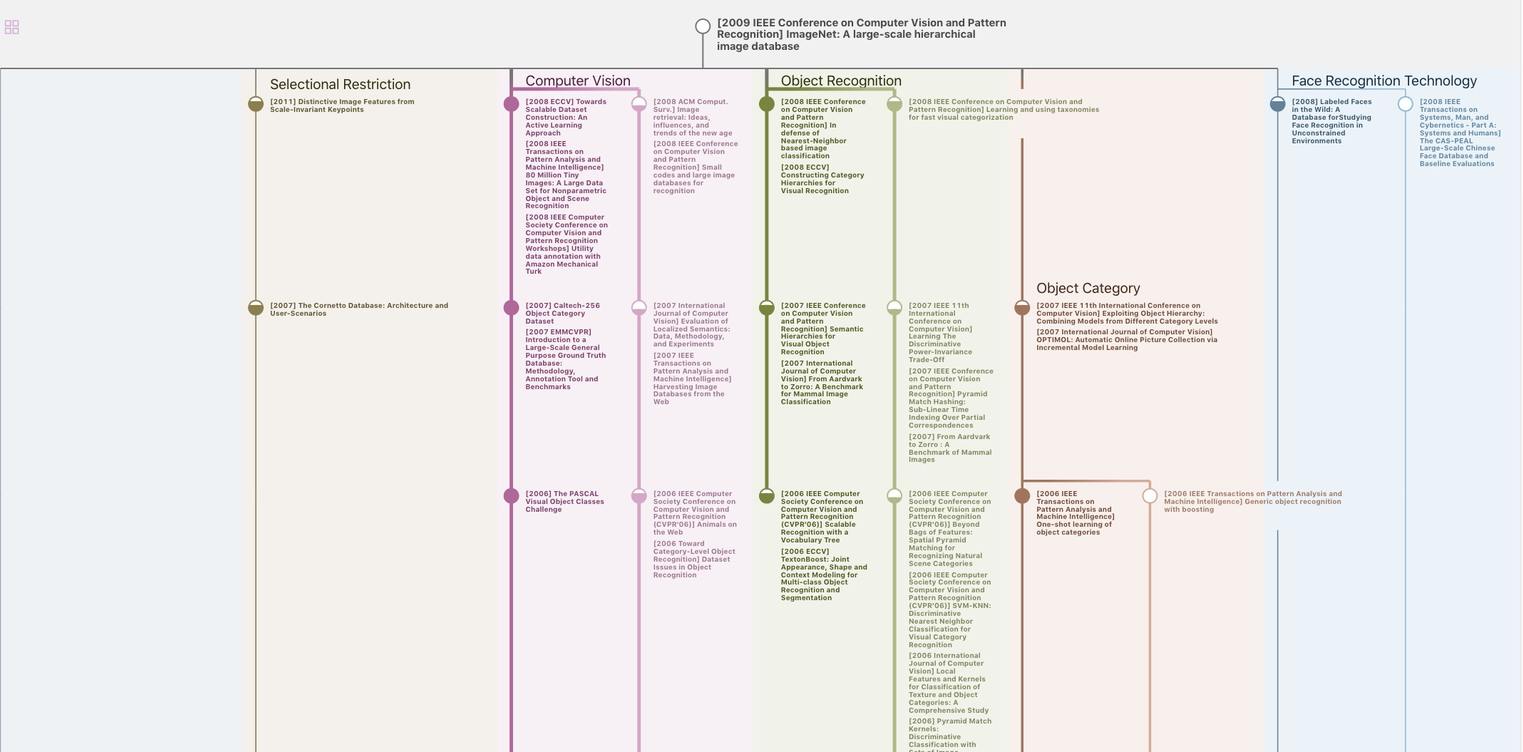
生成溯源树,研究论文发展脉络
Chat Paper
正在生成论文摘要