Ellipse IoU Loss: Better Learning for Rotated Bounding Box Regression
IEEE GEOSCIENCE AND REMOTE SENSING LETTERS(2024)
摘要
Rotated object detection is an important research content in the field of remote-sensing images. However, in the rotated object detection, the inconsistency between the loss function and the final detection metric has become an important factor restricting the improvement of detection accuracy. So, in this letter, an ellipse intersection over union (IoU) loss (EPIoU loss) is proposed to solve these problems. The EPIoU loss uses IoU between the bounding boxes' inscribed ellipses, which is approximate to the original bounding box IoU. This loss function can jointly optimize the prediction box parameters and promote the model to locate the object better. Compared to the complex intersection of rotated rectangles, the intersection calculation of two rotated ellipses is simple. A unified and differentiable process is also designed to calculate EPIoU, which avoids the complexity of the original bounding box IoU calculation. The experiments on DOTA, DIOR, and HRSC datasets verify that the proposed loss function can effectively improve the accuracy of the model.
更多查看译文
关键词
Detectors,Remote sensing,Object detection,Training,Standards,Predictive models,Loss measurement,Loss function,remote-sensing images,rotated object detection
AI 理解论文
溯源树
样例
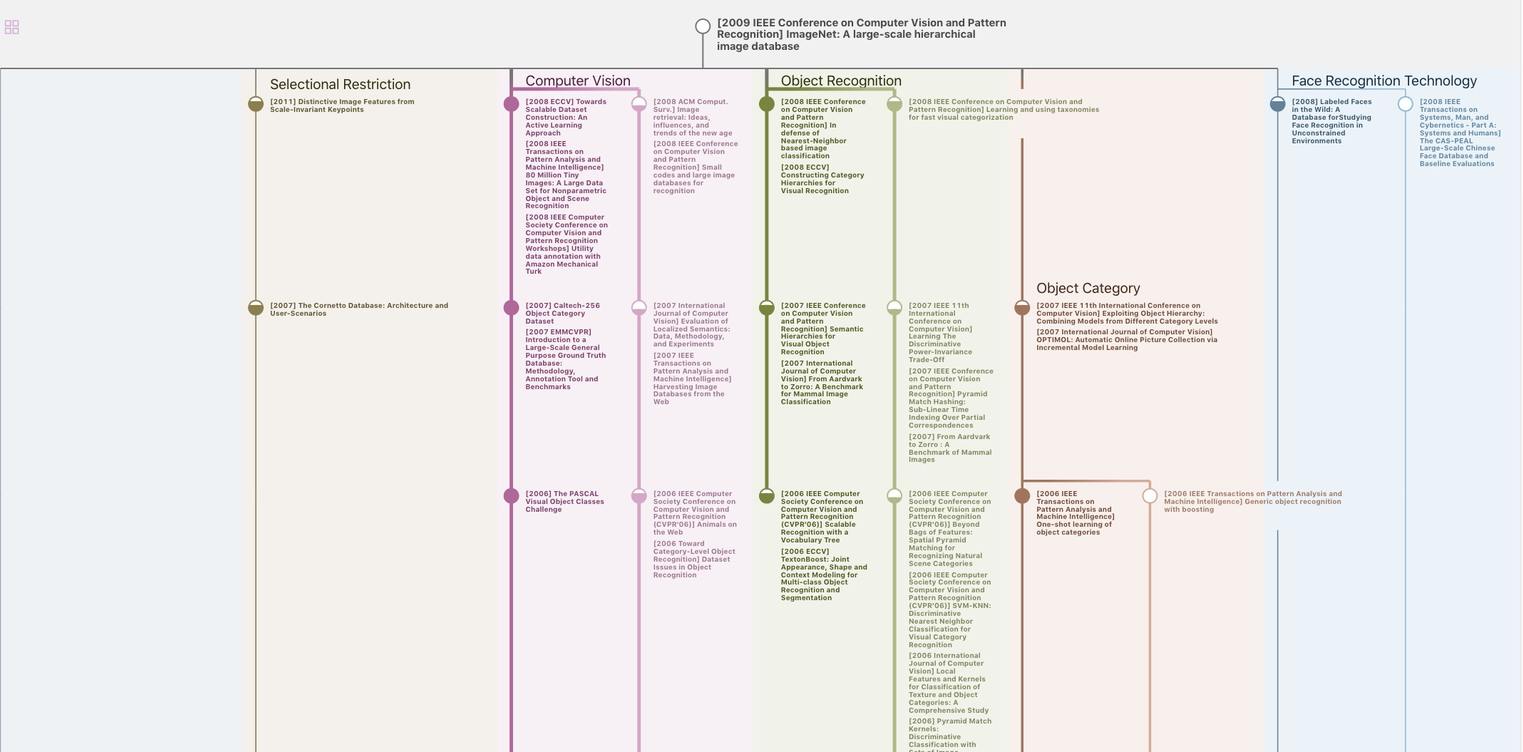
生成溯源树,研究论文发展脉络
Chat Paper
正在生成论文摘要