SNUNet3+: A Full-Scale Connected Siamese Network and a Dataset for Cultivated Land Change Detection in High-Resolution Remote-Sensing Images
IEEE TRANSACTIONS ON GEOSCIENCE AND REMOTE SENSING(2024)
摘要
The decline of cultivated land significantly threatens the food supply. In recent years, remote sensing (RS) change detection emerged as a valuable tool for monitoring nonagriculturalization. However, existing deep learning (DL) methods for change detection suffer from the problem of inadequate utilization of feature information during image feature extraction, leading to noisy or inaccurate change maps. To address these challenges, we propose a Siamese network based on full-scale connected UNet (SNUNet3+), whose encoder extracts features from the original images at various levels. Then, it merges all fine-grained spatial information and coarse-grained semantic information into the decoder through full-scale skip connections to obtain the feature maps with complete information. The spatial and channel squeeze and excitation (scSE) attention mechanism is embedded within the decoder subnetwork to enhance the discriminative power of the features. In addition, a deep supervision module is introduced to improve the feature learning capability of the hidden layers and the quality of features. Moreover, accurate cultivated land change detection remains challenging because of the lack of fine-grained detection datasets. To enable the proposed method to achieve cultivated land change detection, we produced a cultivated land change detection dataset containing 5170 pairs of 256 x 256 bitemporal images with a spatial resolution of 1 m. The effectiveness and performance of the proposed method are evaluated on three high-resolution RS change detection datasets. Extensive experimental results show that the proposed method outperforms other state-of-the-art methods, achieving the highest F1 -score of 72.90%, 90.36%, and 96.64% on the CLCD dataset, LEVIR-CD dataset, and PX-CLCD dataset, respectively.
更多查看译文
关键词
Feature extraction,Remote sensing,Transformers,Convolutional neural networks,Deep learning,Decoding,Task analysis,Change detection,deep learning (DL),nonagriculturalization of cultivated land,remote sensing (RS),UNet3+
AI 理解论文
溯源树
样例
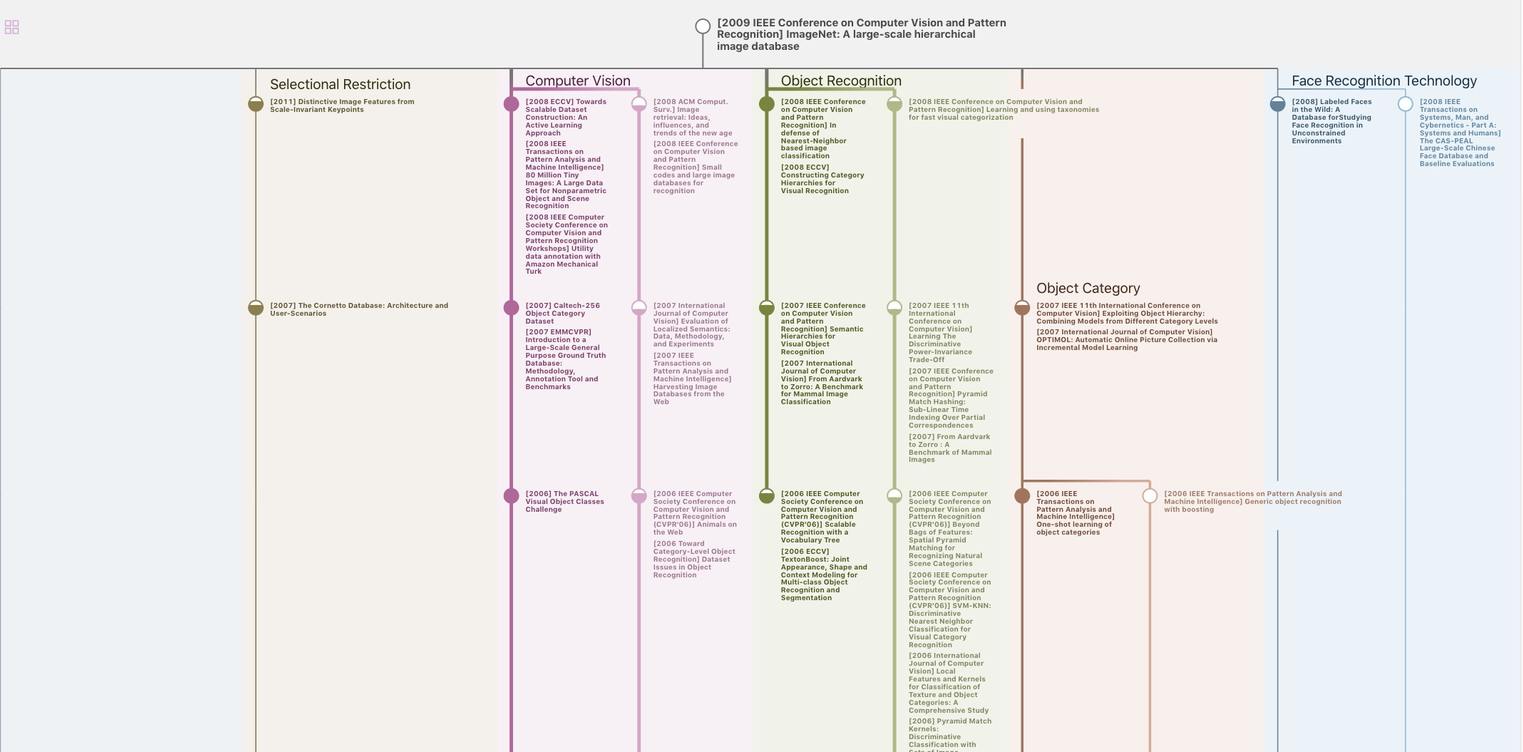
生成溯源树,研究论文发展脉络
Chat Paper
正在生成论文摘要