Enhancing Cascade Quality Prediction Method in Handling Imbalanced Dataset Using Synthetic Minority Over-Sampling Technique
INDUSTRIAL ENGINEERING AND MANAGEMENT SYSTEMS(2023)
摘要
Assessing the production process primarily revolves around quality. When dealing with a basic manufacturing process, quality can be easily anticipated. However, as manufacturing processes grow in complexity, it has been discovered through prior studies that directly predicting the quality of an intricate production system becomes challenging. This is due to the interdependency between each stage of manufacturing, where the outcomes of preceding stages impact subsequent processes. To address this issue, the Cascade Quality Prediction Method (CQPM) was developed. However, as this method employed a classification algorithm, CQPM cannot be directly applied when dealing with datasets that lack target variables for prediction or when the distribution of classes is imbalanced. This study aimed to improve the effectiveness of the CQPM in the context of multistage manufacturing. To achieve this, Hotelling's T2 and the Synthetic Minority Over-sampling Technique (SMOTE) algorithm were incorporated during the data preparation phase, especially when dealing with imbalanced class distributions and missing target variables. The results demonstrated that the inclusion of Hotelling's T2 allowed for the application of classification algorithms. By combining the CQPM approach with a random forest classifier and the SMOTE algorithm, notable improvements were observed in the model's performance. The enhanced data pre-processing techniques led to impressive metric values, including 99.95% accuracy, 93.75% G-Mean, and 96.76% F-Measure. These findings indicate the potential of the proposed model framework to accurately predict quality in multistage manufacturing systems.
更多查看译文
关键词
CQPM,Multistage Manufacturing,Quality Prediction,SMOTE,Hotelling's T2
AI 理解论文
溯源树
样例
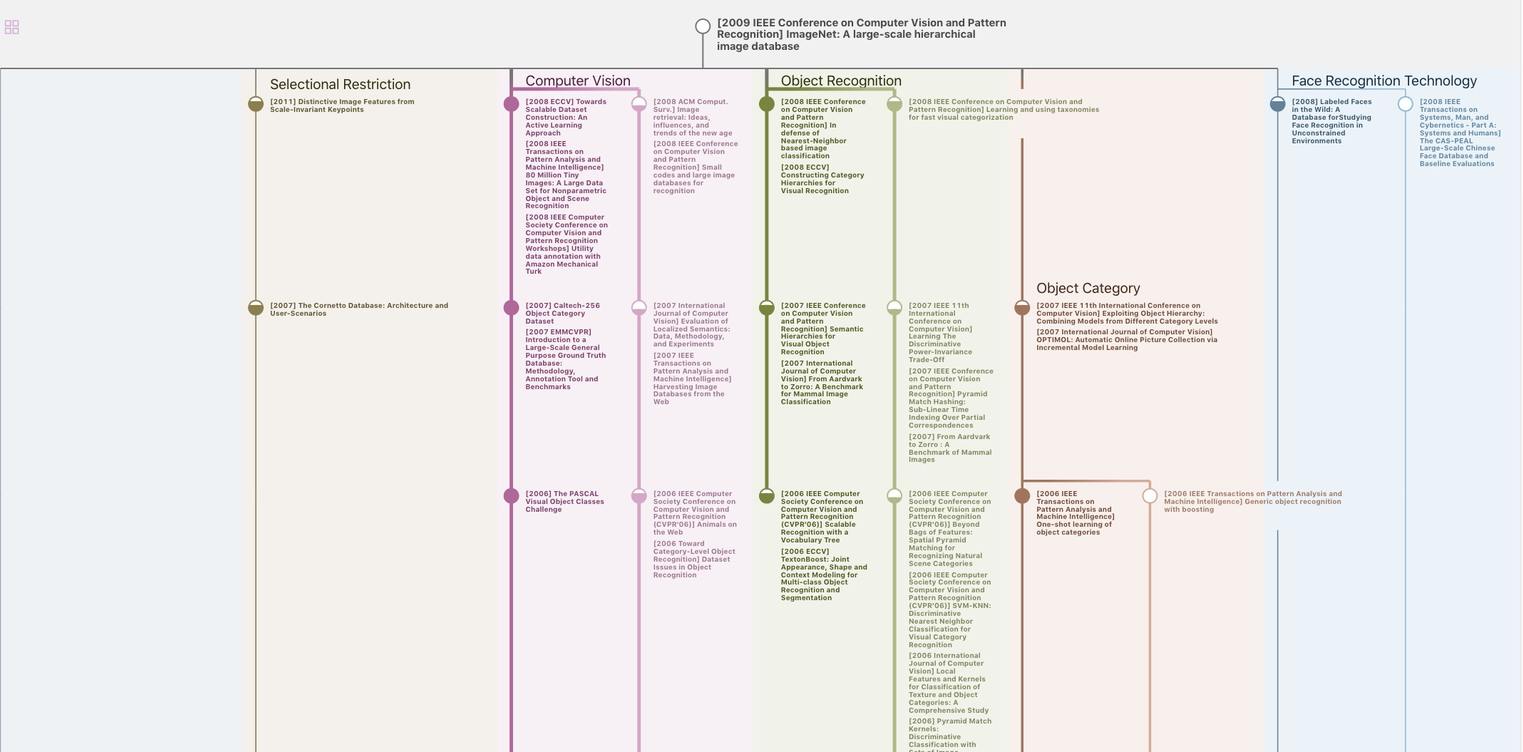
生成溯源树,研究论文发展脉络
Chat Paper
正在生成论文摘要