Low-biased probabilistic multipliers using complementary inaccurate compressors
DIGITAL SIGNAL PROCESSING(2024)
摘要
This paper proposes low -biased probabilistic multipliers and applies the proposed designs to the inference stage of convolutional neural networks (CNNs). Highly inaccurate compressors in the probabilistic multiplier make the error distribution unbalanced and produce significant relative errors. We describe design rules for applying different compressors to the probabilistic multiplier. Besides, the proposed design rules enhance the error characteristics by determining the input values of each compressor, suppressing the significant relative error. Notably, motivated by the design rules, we propose a novel inaccurate 4:2 compressor suitable for our new design. The design rules and proposed 4:2 compressor are adopted in 8 -bit probabilistic multipliers. The error analysis shows that the new designs can enhance error characteristics without increasing hardware costs. Furthermore, the proposed approximate multipliers are applied to the convolutional layers of CNNs. We describe the fine-tuning scheme to reduce the retraining time of approximation -aware training. The experiments show that proposed designs can produce acceptable classification results compared with those using the floating-point and 8 -bit exact multiplications on CIFAR and ImageNet datasets.
更多查看译文
关键词
Approximate computing,Arithmetic unit,Convolutional neural networks,Probabilistic multiplier
AI 理解论文
溯源树
样例
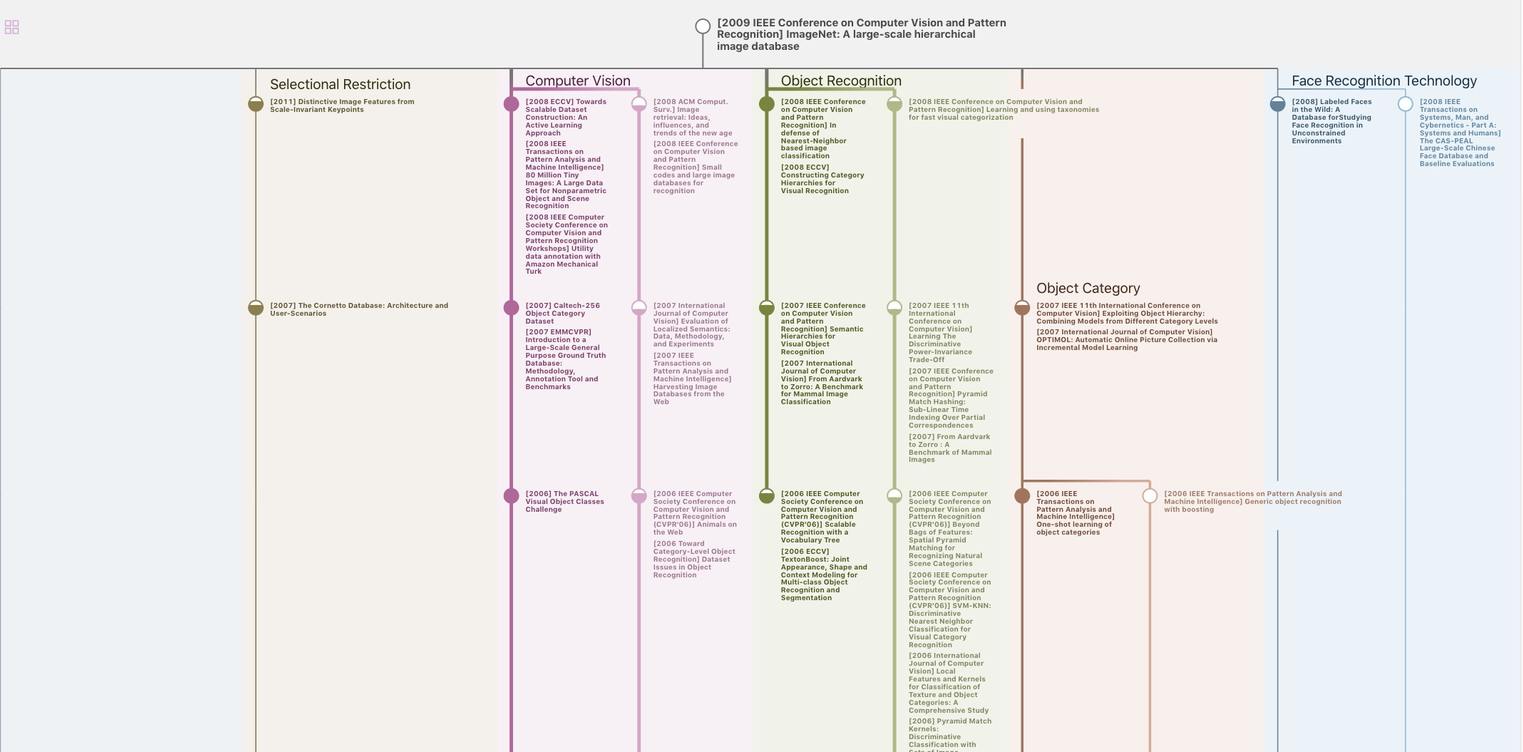
生成溯源树,研究论文发展脉络
Chat Paper
正在生成论文摘要