Evaluation and improvement of temporal robustness and transfer performance of surface soil moisture estimated by machine learning regression algorithms
COMPUTERS AND ELECTRONICS IN AGRICULTURE(2024)
摘要
The accurate monitoring of the spatio-temporal distribution of surface soil moisture (SSM) is crucial to understanding Earth's water cycle. The machine learning regression (MLR) algorithm has brought a new breakthrough to SSM remote sensing estimation due to its powerful nonlinear fitting ability. However, the high-precision estimation of temporal SSM still faces challenges. In this study, we evaluated the temporal robustness and transfer performance of six MLR algorithms for SSM estimation and conducted a universality experiment in agricultural areas with abundant land cover types. First, the factors affecting the estimation accuracy of SSM were analyzed from the perspectives of dynamic variables (backscattering, multi-spectrum, and brightness temperature [TB]) and steady variables (soil texture [ST] and soil roughness [SR]). Results showed that radar incidence angle (RIA) is the key factor for estimating SSM accurately from dual polarization backscattering (dual-pol sigma) data. The introduction of multi-spectrum data can considerably improve the estimation accuracy of SSM and is better than that of TB data. The physical measures (ST) or geometric measures (SR) can be used as auxiliary features for multi-spectrum or TB data, which improve the accuracy of SSM estimated from dual-pol sigma data, with similar improvement effects. Multi-source data can effectively reduce the adverse effects of surface heterogeneity on SSM estimation. Ensemble learning and kernel learning algorithms have similar performance in the case of low-dimensional features and small samples. However, the neural network algorithms can correctly reflect the temporal variation of SSM only in the case of multi-dimensional features and large samples. The optimal accuracy of multi-temporal SSM is estimated by the Gaussian process regression algorithm (RMSE = 0.028 cm(3)/cm(3)). Moreover, to address poor transfer performance of temporal SSM in MLR models (RMSE > 0.060 cm(3)/cm(3)), this study proposed three strategies including the constraint of target domain samples, scattering model, and clustering model. The optimal error (RMSE) of multi-temporal SSM estimated by the three strategies is 0.032, 0.045, and 0.045 cm(3)/cm(3). Overall, the strategies proposed can mitigate the issue of overestimation or underestimation resulting from the inconsistent distribution of SSM and RIAs at various phases. The soil parameters, acting as the medium across different phases, can notably enhance the temporal transfer performance of SSM regression models. This study introduces a novel framework for achieving high estimation accuracy and transfer performance of temporal SSM at a regional scale.
更多查看译文
关键词
Surface soil moisture,Machine learning regression,Temporal robustness,Transfer learning,SMAPVEX16-MB
AI 理解论文
溯源树
样例
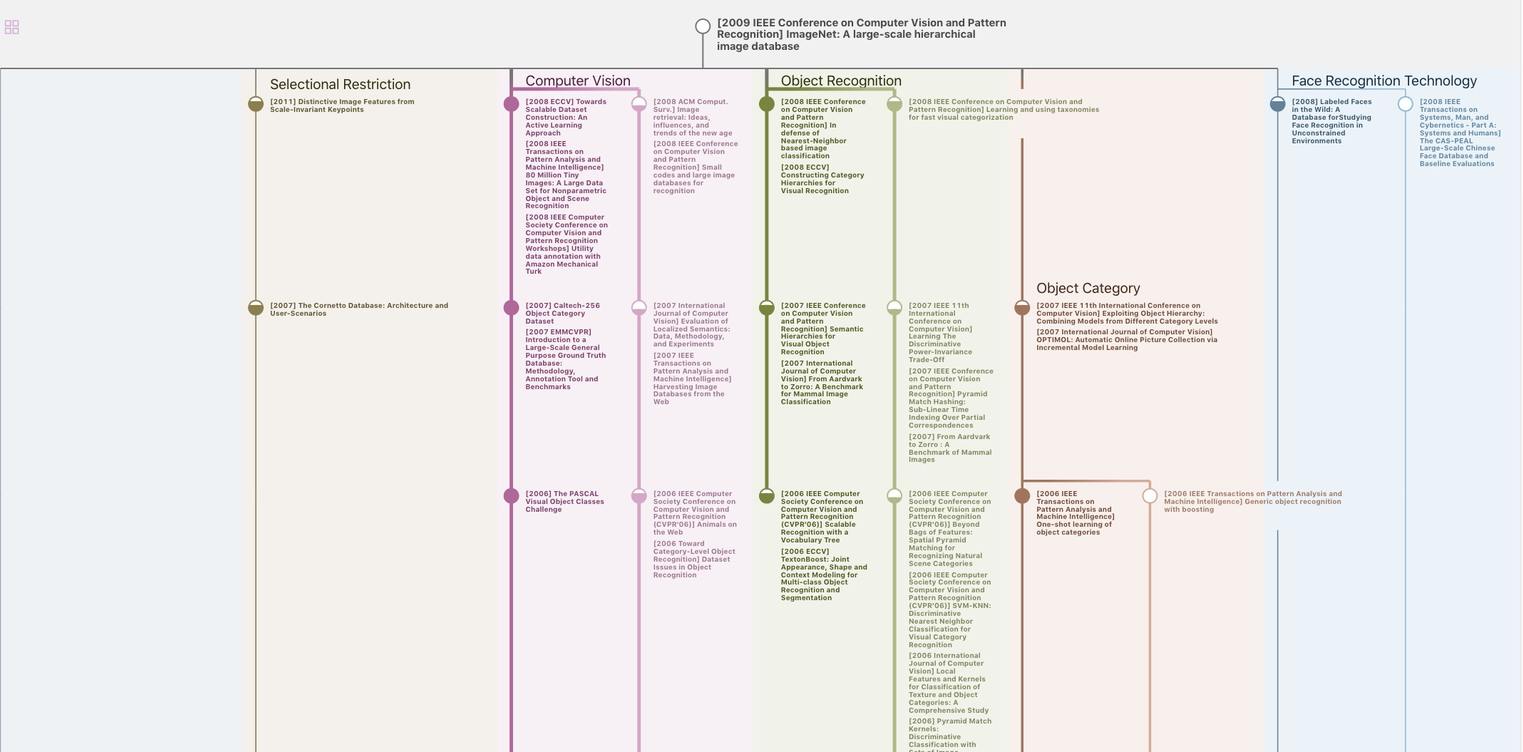
生成溯源树,研究论文发展脉络
Chat Paper
正在生成论文摘要