Talking about diseases; developing a model of patient and public-prioritised disease phenotypes
medrxiv(2023)
摘要
Background Deep phenotyping describes the use of formal and standardised terminologies to create comprehensive phenotypic descriptions of biomedical phenomena. While most often employed to describe patients, phenotype models may also be developed to characterise diseases. These characterisations facilitate secondary analysis, evidence synthesis, and practitioner awareness, thereby guiding patient care. The vast majority of this knowledge is derived from sources that describe an academic understanding of disease, including academic literature and experimental databases. Previous work has revealed a gulf between the priorities, perspectives, and perceptions held by healthcare researchers and providers and the users of clinical services. A comparison between canonical disease descriptions and phenotype models developed from public discussions of disease offers the prospect of discovery of new phenotypes, patient population stratification, and targeted mitigation of symptoms most damaging to patients quality of life.
Methods Using a dataset representing disease and phenotype co-occurrence in social media text, we employ semantic techniques to identify phenotype associations for a set of common and rare diseases, constituting a phenotype model for those diseases that represents the public perspective. We create an integrated resource for biomedical database and literature-derived disease-phenotype associations by aligning data from several previous studies. We then explore differences between the disease-phenotype associations derived from writing in social media with those from the clinical literature and biomedical databases, with a focus on identification of differential themes and novel phenotypes. We also perform an evaluation of associations for several diseases, with specialist clinicians reviewing associations for validity, feasibility, and involvement in clinical care.
Findings We identified 35,782 significant disease-phenotype associations from social media across 311 diseases, of which 304 could be linked to a combined resource of associations derived from academic sources. Social media-derived disease profiles recapitulated those from academic sources (AUC=0.874 (.95=0.858-0.891)). We further identified 26,081 novel phenotype associations that were not contained in the academic sources, of which 15,084 were considered significant. Constitutional symptoms, those holistic manifestations of disease affecting quality of life, were strongly over-represented in the social media phenotype, contributing more associations especially to endocrine, digestive, and reproductive diseases. An expert clinical review found that social media-derived associations were considered similarly well-established to those derived from literature, and were seen significantly more in patient clinical encounters.
Interpretation The phenotype model recovered from social media presents a significantly different perspective than existing resources derived from biomedical databases and literature, providing a large number of associations novel to the latter dataset. We propose that the integration and interrogation of these public perspectives on disease can inform clinical awareness, improve secondary analysis, and bridge understanding across healthcare stakeholders.
### Competing Interest Statement
James Wright is an employee of White Swan, who provided the dataset for this study. Otherwise, the authors declare that they have no competing interests.
### Funding Statement
The authors acknowledge support from the NIHR Birmingham ECMC, NIHR Birmingham SRMRC, Nanocommons H2020-EU (731032), NIHR BBRC, MRC HDR UK (HDRUK/CFC/01), KAUST OSR (URF/1/3790-01-01), and MRC (MR/S003991/1), MAESTRIA (Grant agreement ID 965286), HYPERMARKER (Grant agreement ID 101095480), PARC (Grant Agreement No 101057014) and the MRC Heath Data Research UK (HDRUK/CFC/01) and HDRUK midlands regional community project [QQ2], initiatives funded by UK Research and Innovation, Department of Health and Social Care (England) and the devolved administrations, and leading medical research charities. The views expressed in this publication are those of the authors and not necessarily those of the NHS, the National Institute for Health Research, the Medical Research Council or the Department of Health. PNS acknowledges the support of The Alan Turing Institute.
### Author Declarations
I confirm all relevant ethical guidelines have been followed, and any necessary IRB and/or ethics committee approvals have been obtained.
Yes
The details of the IRB/oversight body that provided approval or exemption for the research described are given below:
Science, Technology, Engineering and Mathematics Committee Ethics board of University of Birmingham gave ethical approval for this work with ERN\_2022-0241 and amendment ERN\_0241-Jun2023.
I confirm that all necessary patient/participant consent has been obtained and the appropriate institutional forms have been archived, and that any patient/participant/sample identifiers included were not known to anyone (e.g., hospital staff, patients or participants themselves) outside the research group so cannot be used to identify individuals.
Yes
I understand that all clinical trials and any other prospective interventional studies must be registered with an ICMJE-approved registry, such as ClinicalTrials.gov. I confirm that any such study reported in the manuscript has been registered and the trial registration ID is provided (note: if posting a prospective study registered retrospectively, please provide a statement in the trial ID field explaining why the study was not registered in advance).
Yes
I have followed all appropriate research reporting guidelines, such as any relevant EQUATOR Network research reporting checklist(s) and other pertinent material, if applicable.
Yes
The result data and intermediate data are available via and .
更多查看译文
AI 理解论文
溯源树
样例
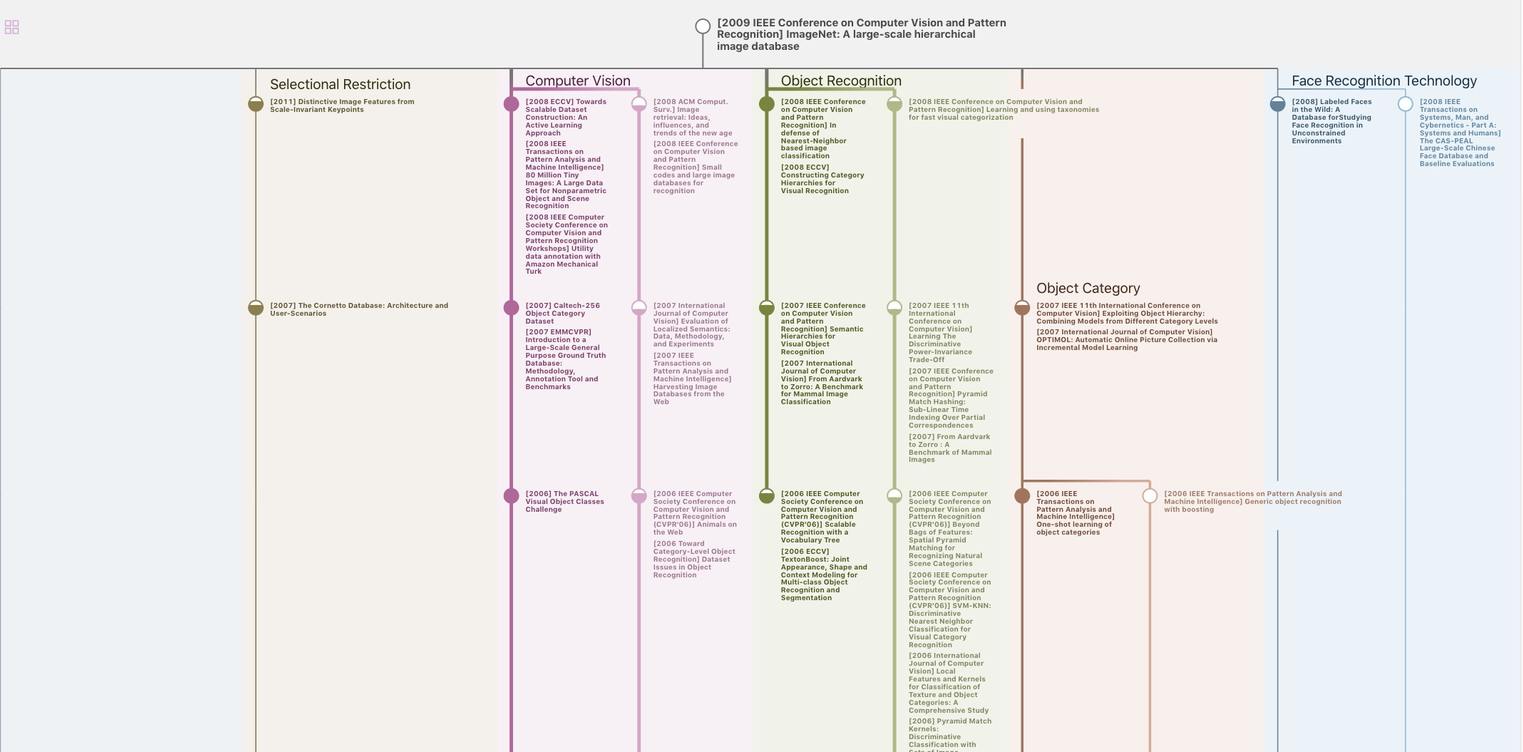
生成溯源树,研究论文发展脉络
Chat Paper
正在生成论文摘要