Patient-Oriented Unsupervised Learning to Unlock Patterns of Multimorbidity Associated with Stroke using Primary Care Electronic Health Records
arxiv(2024)
摘要
Background: Identifying and characterising the longitudinal patterns of
multimorbidity associated with stroke is needed to better understand patients'
needs and inform new models of care.
Methods: We used an unsupervised patient-oriented clustering approach to
analyse primary care electronic health records (EHR) of 30 common long-term
conditions (LTC), in patients with stroke aged over 18, registered in 41
general practices in south London between 2005 and 2021.
Results: Of 849,968 registered patients, 9,847 (1.16
stroke, 46.5
77.0). The median number of LTCs in addition to stroke was 3 (IQR: from 2 to
5). Patients were stratified in eight clusters. These clusters revealed
contrasted patterns of multimorbidity, socio-demographic characteristics (age,
gender and ethnicity) and risk factors. Beside a core of 3 clusters associated
with conventional stroke risk-factors, minor clusters exhibited less common but
recurrent combinations of LTCs including mental health conditions, asthma,
osteoarthritis and sickle cell anaemia. Importantly, complex profiles combining
mental health conditions, infectious diseases and substance dependency emerged.
Conclusion: This patient-oriented approach to EHRs uncovers the heterogeneity
of profiles of multimorbidity and socio-demographic characteristics associated
with stroke. It highlights the importance of conventional stroke risk factors
as well as the association of mental health conditions in complex profiles of
multimorbidity displayed in a significant proportion of patients. These results
address the need for a better understanding of stroke-associated multimorbidity
and complexity to inform more efficient and patient-oriented healthcare models.
更多查看译文
AI 理解论文
溯源树
样例
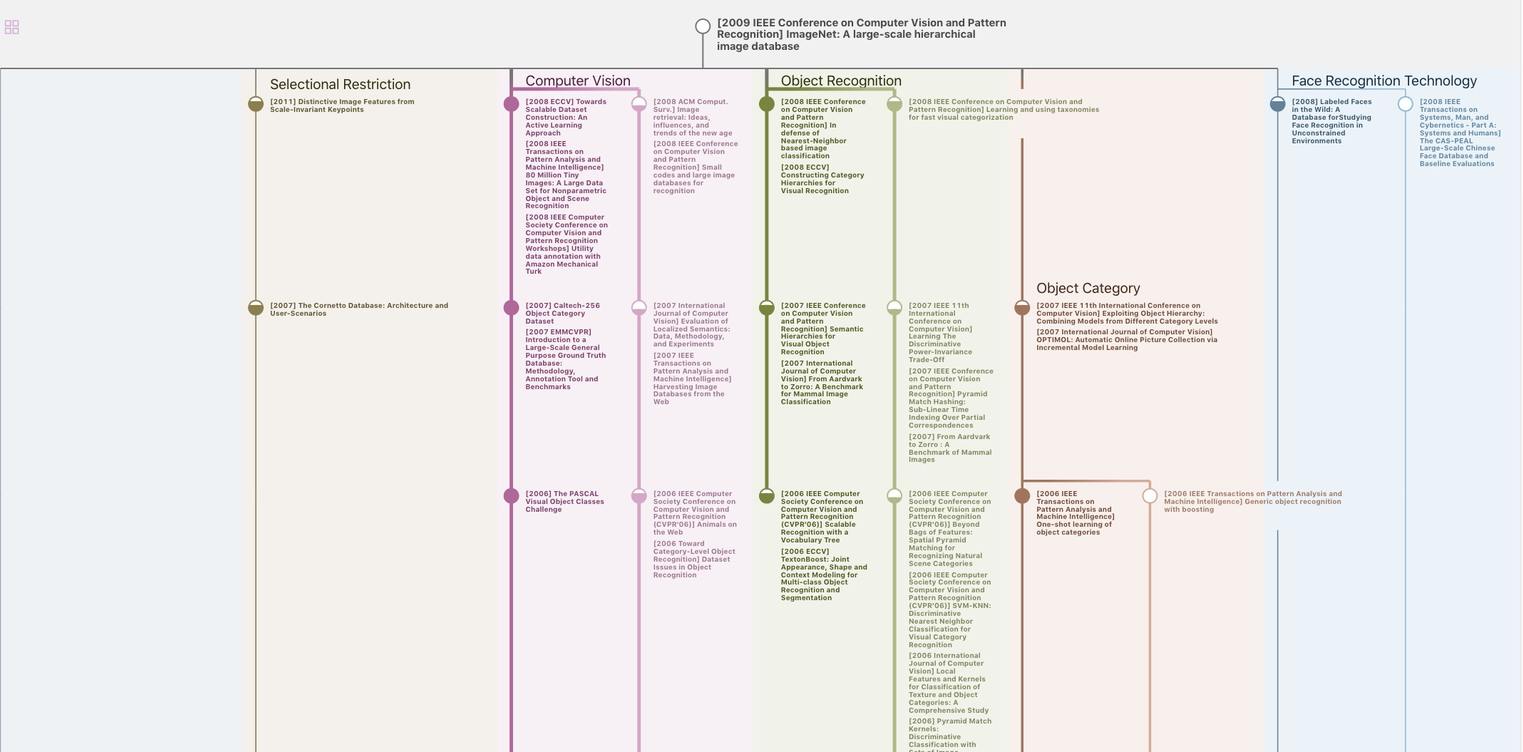
生成溯源树,研究论文发展脉络
Chat Paper
正在生成论文摘要