Outlier Ranking in Large-Scale Public Health Streams
CoRR(2024)
摘要
Disease control experts inspect public health data streams daily for outliers
worth investigating, like those corresponding to data quality issues or disease
outbreaks. However, they can only examine a few of the thousands of
maximally-tied outliers returned by univariate outlier detection methods
applied to large-scale public health data streams. To help experts distinguish
the most important outliers from these thousands of tied outliers, we propose a
new task for algorithms to rank the outputs of any univariate method applied to
each of many streams. Our novel algorithm for this task, which leverages
hierarchical networks and extreme value analysis, performed the best across
traditional outlier detection metrics in a human-expert evaluation using public
health data streams. Most importantly, experts have used our open-source Python
implementation since April 2023 and report identifying outliers worth
investigating 9.1x faster than their prior baseline. Other organizations can
readily adapt this implementation to create rankings from the outputs of their
tailored univariate methods across large-scale streams.
更多查看译文
AI 理解论文
溯源树
样例
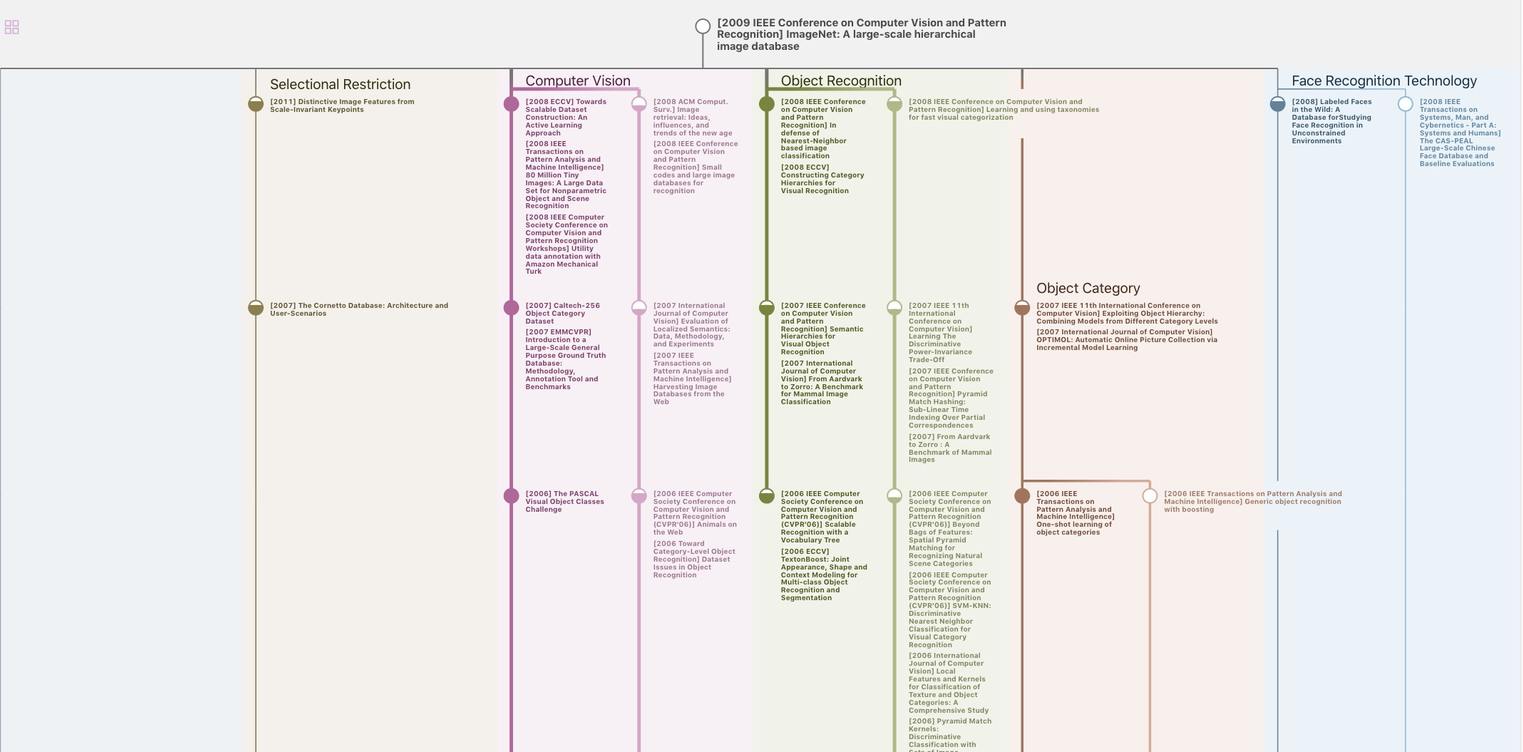
生成溯源树,研究论文发展脉络
Chat Paper
正在生成论文摘要