Multi-modal Learning with Missing Modality in Predicting Axillary Lymph Node Metastasis
2023 IEEE International Conference on Bioinformatics and Biomedicine (BIBM)(2024)
摘要
Multi-modal Learning has attracted widespread attention in medical image
analysis. Using multi-modal data, whole slide images (WSIs) and clinical
information, can improve the performance of deep learning models in the
diagnosis of axillary lymph node metastasis. However, clinical information is
not easy to collect in clinical practice due to privacy concerns, limited
resources, lack of interoperability, etc. Although patient selection can ensure
the training set to have multi-modal data for model development, missing
modality of clinical information can appear during test. This normally leads to
performance degradation, which limits the use of multi-modal models in the
clinic. To alleviate this problem, we propose a bidirectional distillation
framework consisting of a multi-modal branch and a single-modal branch. The
single-modal branch acquires the complete multi-modal knowledge from the
multi-modal branch, while the multi-modal learns the robust features of WSI
from the single-modal. We conduct experiments on a public dataset of Lymph Node
Metastasis in Early Breast Cancer to validate the method. Our approach not only
achieves state-of-the-art performance with an AUC of 0.861 on the test set
without missing data, but also yields an AUC of 0.842 when the rate of missing
modality is 80%. This shows the effectiveness of the approach in dealing with
multi-modal data and missing modality. Such a model has the potential to
improve treatment decision-making for early breast cancer patients who have
axillary lymph node metastatic status.
更多查看译文
关键词
Missing modality,Whole slide image,Clinical data
AI 理解论文
溯源树
样例
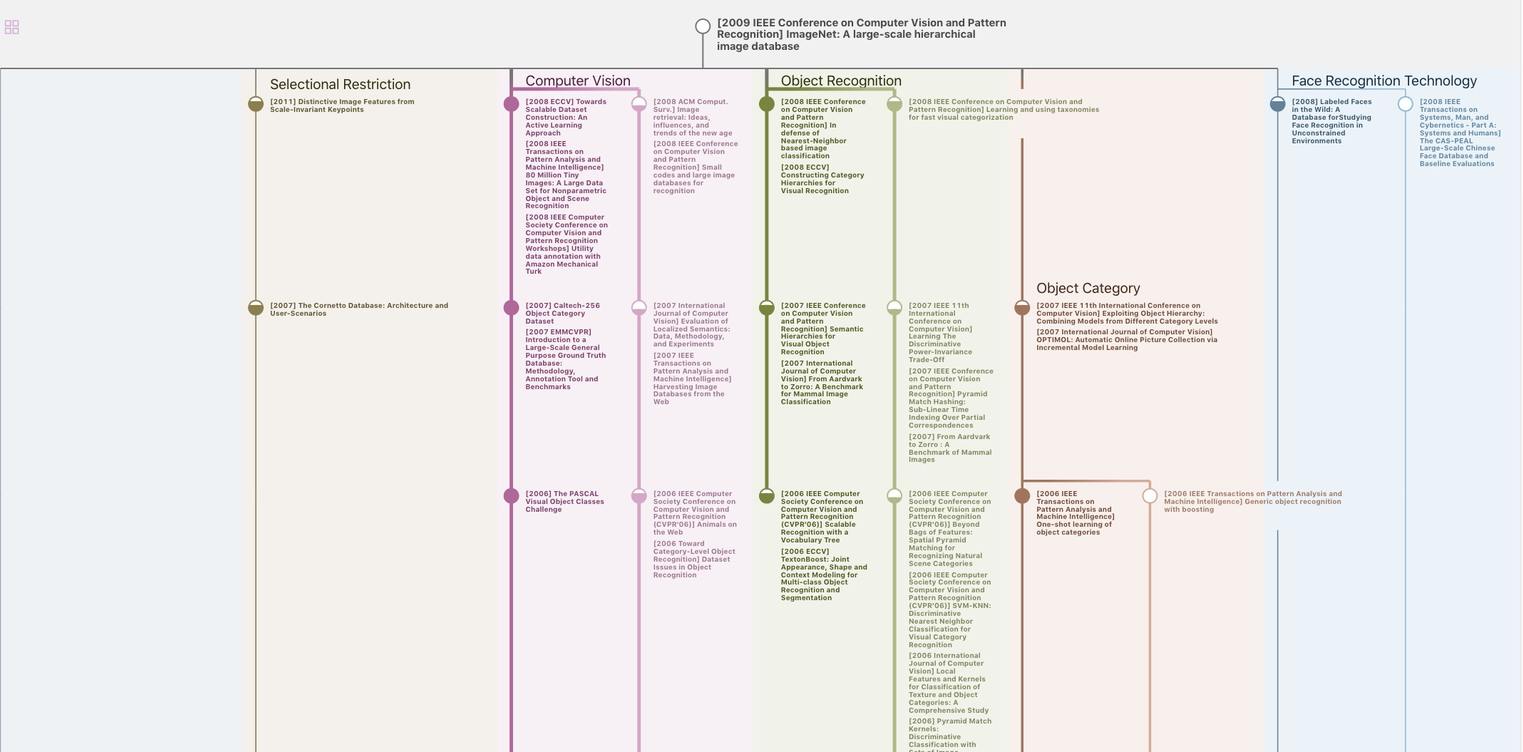
生成溯源树,研究论文发展脉络
Chat Paper
正在生成论文摘要