Multi-label contrastive hashing
Pattern Recognition(2024)
摘要
Joint learning of image representation and hash encoding represents a dominant solution to approximate nearest neighbor search for large-scale image retrieval. Despite significant advances in deep learning to hash in multi-label setting, optimization of semantic similarity-preserving representations with minimal quantization error remains challenging. Motivated by the recent success of contrastive representation learning in various vision tasks, this article introduces a Multi-label Contrastive Hashing (MCH) method for large-scale multi-label image retrieval. We extend the image similarity modeling in existing supervised contrastive loss from binary to multi-level structure, by which multi-level semantic similarity between multi-label images can be well modeled and captured in learning to hash. Despite the appealing properties of contrastive learning, directly adapting it into multi-label hashing is non-trivial, as the quantization loss may restricts the optimization of the multi-level contrastive loss, degrading the multi-level similarity-preserving hashing. To this end, we design a curriculum strategy to adaptively adjust the weight of quantization loss by leveraging the historical quantization deviations during training, such that the multi-level semantic similarity can be well preserved with progressively reduced quantization deviation. We conduct extensive experiments on three benchmark datasets including MirFlickr25k, NUS-WIDE, and IAPRTC-12. The results indicate the effectiveness of our approach, outperforming several state-of-the-art solutions for hashing-based multi-label image retrieval.
更多查看译文
关键词
Multi-label hashing,Multi-level similarity,Supervised contrastive learning,Curriculum learning,Quantization deviation
AI 理解论文
溯源树
样例
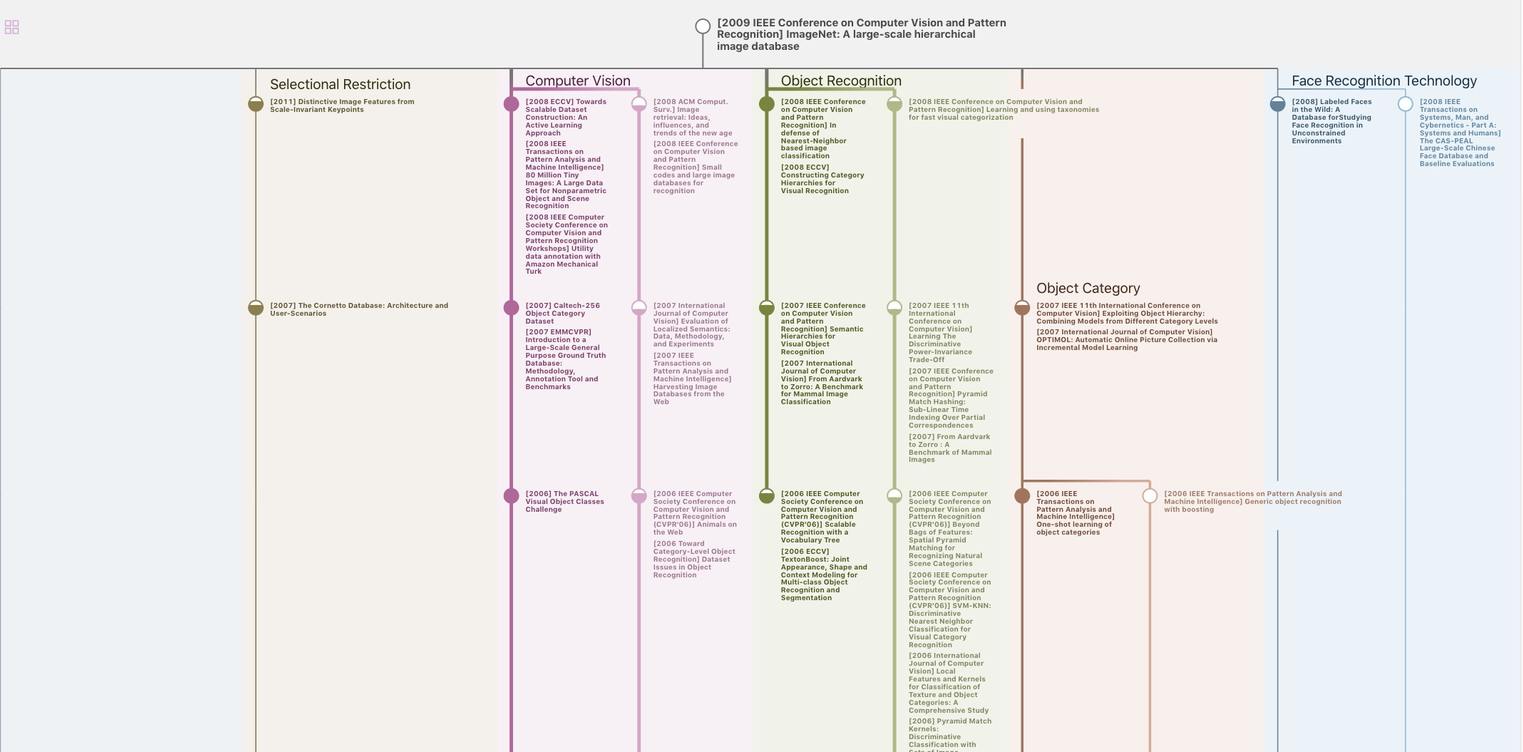
生成溯源树,研究论文发展脉络
Chat Paper
正在生成论文摘要