An Exploration of the accuracy of TOF Automatic Segmentation Algorithm based on U-net model.
2023 16th International Congress on Image and Signal Processing, BioMedical Engineering and Informatics (CISP-BMEI)(2023)
摘要
In order to enhance the reconstruction of Tetralogy of Fallot (TOF) cardiovascular models and facilitate the development of individualized treatment strategies for clinical practitioners, this study employed the modeling outcomes from Materialise
®
-Mimics as a reference point to investigate the modeling effectiveness of the existing automatic segmentation algorithm based on the U-Net model. We selected a single case and performed three-dimensional modeling using both the automatic segmentation algorithm and Mimics. Subsequently, we utilized Mimics to measure morphological parameters, including pulmonary artery diameter and angles, from the models generated by the two aforementioned methods, as well as from the two-dimensional images. The parameters acquired from the two-dimensional plane served as the standard reference. Discrepancies and relative errors between the parameters obtained from the two models and the reference values were calculated, while smaller discrepancies and parameter errors indicated greater model accuracy. Although the models generated through both methods exhibited substantial morphological similarity, the algorithmic models excluded image information at the juncture of the right ventricle and pulmonary artery. Furthermore, the data revealed that the discrepancies and relative errors in the algorithmic model's measurements were higher than those observed in the Mimics-generated models compared to the reference values. Consequently, it is inferred that the modeling efficacy of the automatic segmentation algorithm based on the U-Net model is promising, yet further model training is required to enhance modeling precision.
更多查看译文
关键词
Tetralogy of Fallot,U-Net,Geometry,Construction
AI 理解论文
溯源树
样例
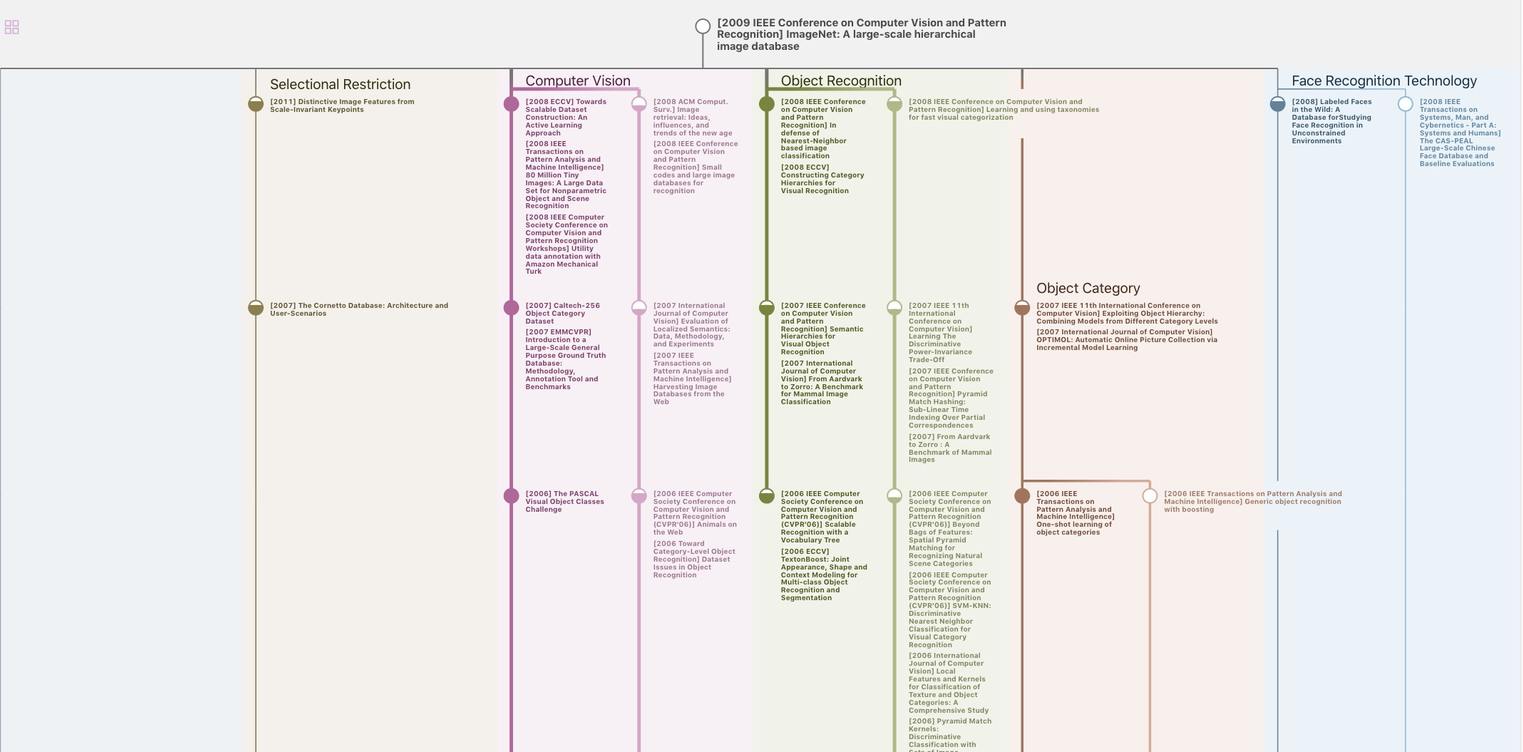
生成溯源树,研究论文发展脉络
Chat Paper
正在生成论文摘要