Exploring Entropy-Based Classification of Time Series Using Visibility Graphs from Chaotic Maps
crossref(2023)
摘要
In this paper, we propose a method for assessing the effectiveness of entropy features using several chaotic mappings. We anlyze fuzzy entropy (FuzzyEn) and neural network entropy (NNetEn) on four discrete mappings: the logistic map, the sine map, the Planck map, and the two-memristor-based map, with a base length time series of 300 elements. FuzzyEn is shown to have improved global efficiency (GEFMCC) in the classification task compared to NNetEn. At the same time, there are local areas of the time series dynamics in which the classification efficiency NNetEn is higher than FuzzyEn. The results of using horizontal visibility graphs (HVG) instead of the raw time series are also shown. GEFMCC decreases after HVG time series transformation, but there are local areas of the time series dynamics in which the classification efficiency increases after including the HVG. The scientific community can use the results to explore the efficiency of entropy-based classification of time series.
更多查看译文
AI 理解论文
溯源树
样例
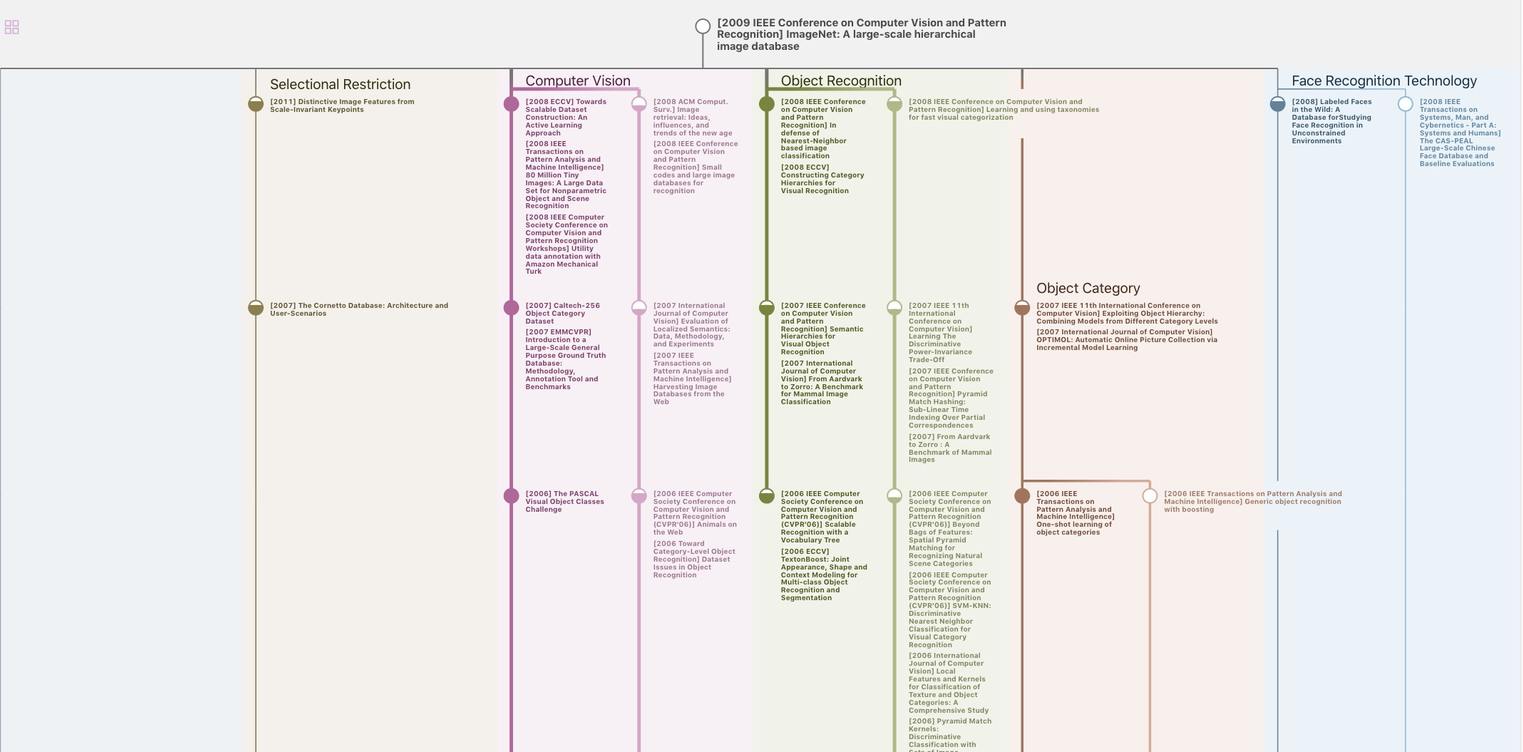
生成溯源树,研究论文发展脉络
Chat Paper
正在生成论文摘要