Inferring biophysical properties of membranes during endocytosis using machine learning
SOFT MATTER(2024)
摘要
Endocytosis is a fundamental cellular process in eukaryotic cells that facilitates the transport of molecules into the cell. With the help of fluorescence microscopy and electron tomography, researchers have accumulated extensive geometric data of membrane shapes during endocytosis. These data contain rich information about the mechanical properties of membranes, which are hard to access via experiments due to the small dimensions of the endocytic patch. In this study, we propose an approach that combines machine learning with the Helfrich theory of membranes to infer the mechanical properties of membranes during endocytosis from a dataset of membrane shapes extracted from electron tomography. Our results demonstrate that machine learning can output solutions that both match the experimental profile and satisfy the membrane shape equations derived from Helfrich theory. The learning results show that during the early stage of endocytosis, the inferred membrane tension is negative, indicating the presence of strong compressive forces at the boundary of the endocytic invagination. Our method presents a generic framework for extracting membrane information from super-resolution imaging. We combine machine learning with the Helfrich theory of membranes to infer the mechanical properties of membranes during endocytosis from a dataset of membrane shapes extracted from electron tomography.
更多查看译文
AI 理解论文
溯源树
样例
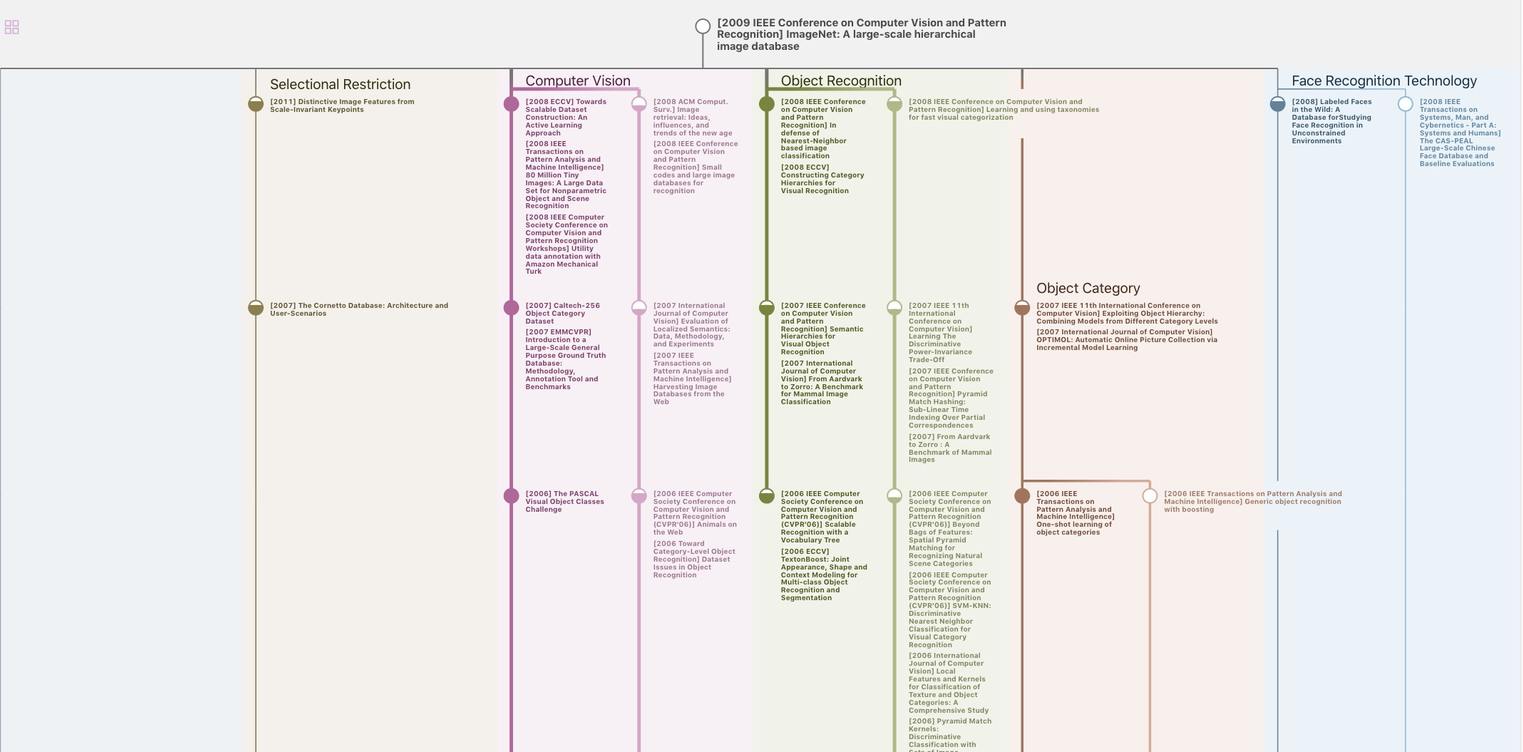
生成溯源树,研究论文发展脉络
Chat Paper
正在生成论文摘要