Non-destructive detection of fusarium head blight in wheat kernels and flour using visible near-infrared and mid-infrared spectroscopy
CHEMOMETRICS AND INTELLIGENT LABORATORY SYSTEMS(2024)
摘要
Fusarium head blight (FHB) is one of the most severe fungal diseases that reduces yield of cereal crops and degrades kernel quality with mycotoxins, which are harmful to human and animal health. The majority of FHB identification at post-harvest stage is through lab-based analysis, whilst effective it is a time consuming, expensive, and laborious process. Hence, a non-destructive, rapid, accurate, and robust method is required for FHB detection at post-harvest. This study explores the potential of visible near-infrared (vis-NIR) in the wavelength range from 400 to 1700 nm and the mid-infrared (MIR) in the wavenumber range from 4000 to 650 cm-1 to predict FHB infection of wheat kernels and flour. A total of 143 ear samples (93 infected, and 50 healthy) were collected from an inoculated trial covering several winter wheat varieties. The collected spectral data was analysed with two different machine learning algorithms, namely, random forest (RF) and linear discriminant analysis (LDA). Both models produced a higher test accuracy of 96.6 % and 100 %, respectively, for the flour samples than that (e.g., 93.1 %) for the kernels, using the MIR spectroscopy. Recursive feature elimination (RFE) demonstrated notable improvements in accuracy of the vis-NIR for the kernels, with LDA model providing 100 % classification accuracy. While RFE failed to improve the accuracy of MIR-LDA models. The results highlight the effectiveness of vis-NIR and MIR spectroscopy with RFE and machine learning for classifying FHB in wheat kernel and flour samples.
更多查看译文
关键词
Fusarium head blight,Visible near -infrared,Mid -infrared,Spectroscopy,Disease detection
AI 理解论文
溯源树
样例
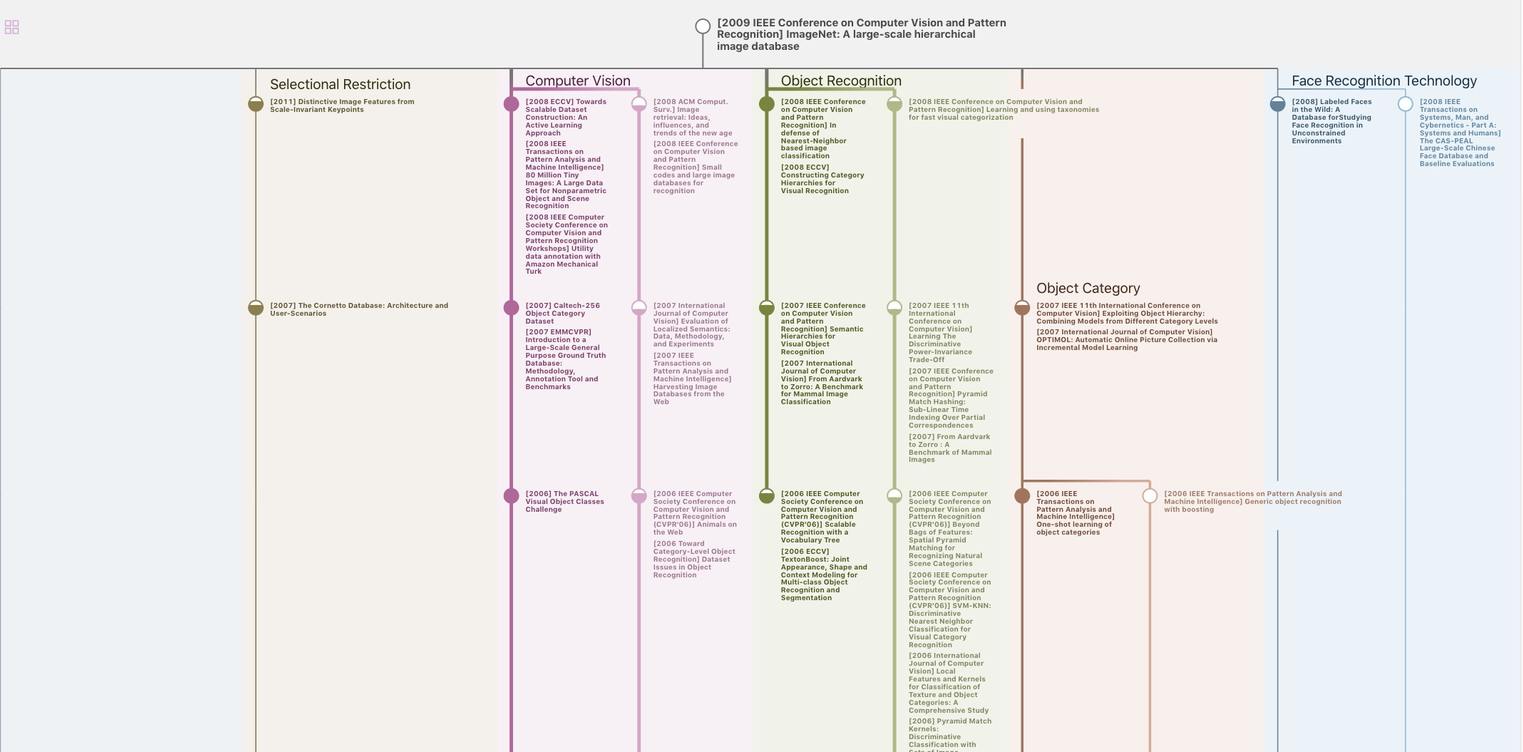
生成溯源树,研究论文发展脉络
Chat Paper
正在生成论文摘要