Dual-Stream Complex-Valued Convolutional Network for Authentic Dehazed Image Quality Assessment
IEEE TRANSACTIONS ON IMAGE PROCESSING(2024)
摘要
Effectively evaluating the perceptual quality of dehazed images remains an under-explored research issue. In this paper, we propose a no-reference complex-valued convolutional neural network (CV-CNN) model to conduct automatic dehazed image quality evaluation. Specifically, a novel CV-CNN is employed that exploits the advantages of complex-valued representations, achieving better generalization capability on perceptual feature learning than real-valued ones. To learn more discriminative features to analyze the perceptual quality of dehazed images, we design a dual-stream CV-CNN architecture. The dual-stream model comprises a distortion-sensitive stream that operates on the dehazed RGB image, and a haze-aware stream on a novel dark channel difference image. The distortion-sensitive stream accounts for perceptual distortion artifacts, while the haze-aware stream addresses the possible presence of residual haze. Experimental results on three publicly available dehazed image quality assessment (DQA) databases demonstrate the effectiveness and generalization of our proposed CV-CNN DQA model as compared to state-of-the-art no-reference image quality assessment algorithms.
更多查看译文
关键词
Streaming media,Image quality,Feature extraction,Transforms,Computational modeling,Task analysis,Convolutional neural networks,Complex-valued convolutional neural network,dual stream network,dehazed image quality assessment,dark channel difference,dual-tree complex wavelet transform
AI 理解论文
溯源树
样例
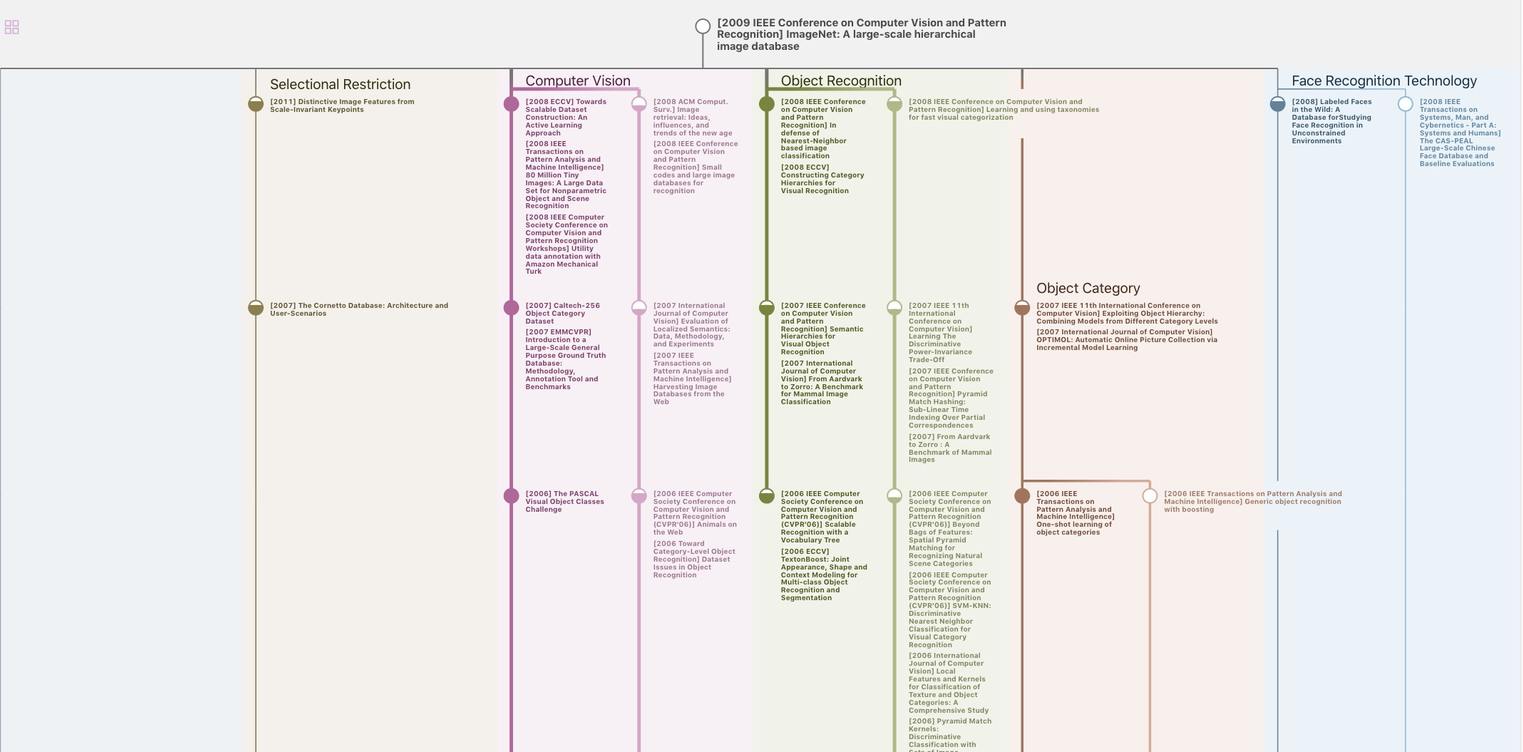
生成溯源树,研究论文发展脉络
Chat Paper
正在生成论文摘要