B2-ViT Net: Broad Vision Transformer Network With Broad Attention for Seizure Prediction
IEEE TRANSACTIONS ON NEURAL SYSTEMS AND REHABILITATION ENGINEERING(2024)
摘要
Seizure prediction are necessary for epileptic patients. The global spatial interactions among channels, and long-range temporal dependencies play a crucial role in seizure onset prediction. In addition, it is necessary to search for seizure prediction features in a vast space to learn new generalized feature representations. Many previous deep learning algorithms have achieved some results in automatic seizure prediction. However, most of them do not consider global spatial interactions among channels and long-range temporal dependencies together, and only learn the feature representation in the deep space. To tackle these issues, in this study, an novel bi-level programming seizure prediction model, B2-ViT Net, is proposed for learning the new generalized spatio-temporal long-range correlation features, which can characterize the global interactions among channels in spatial, and long-range dependencies in temporal required for seizure prediction. In addition, the proposed model can comprehensively learn generalized seizure prediction features in a vast space due to its strong deep and broad feature search capabilities. Sufficient experiments are conducted on two public datasets, CHB-MIT and Kaggle datasets. Compared with other existing methods, our proposed model has shown promising results in automatic seizure prediction tasks, and provides a certain degree of interpretability.
更多查看译文
关键词
Automatic seizure prediction,electroencephalogram (EEG),vision transformer (ViT),multi-head self-attention,broad attention,broad learning system (BLS)
AI 理解论文
溯源树
样例
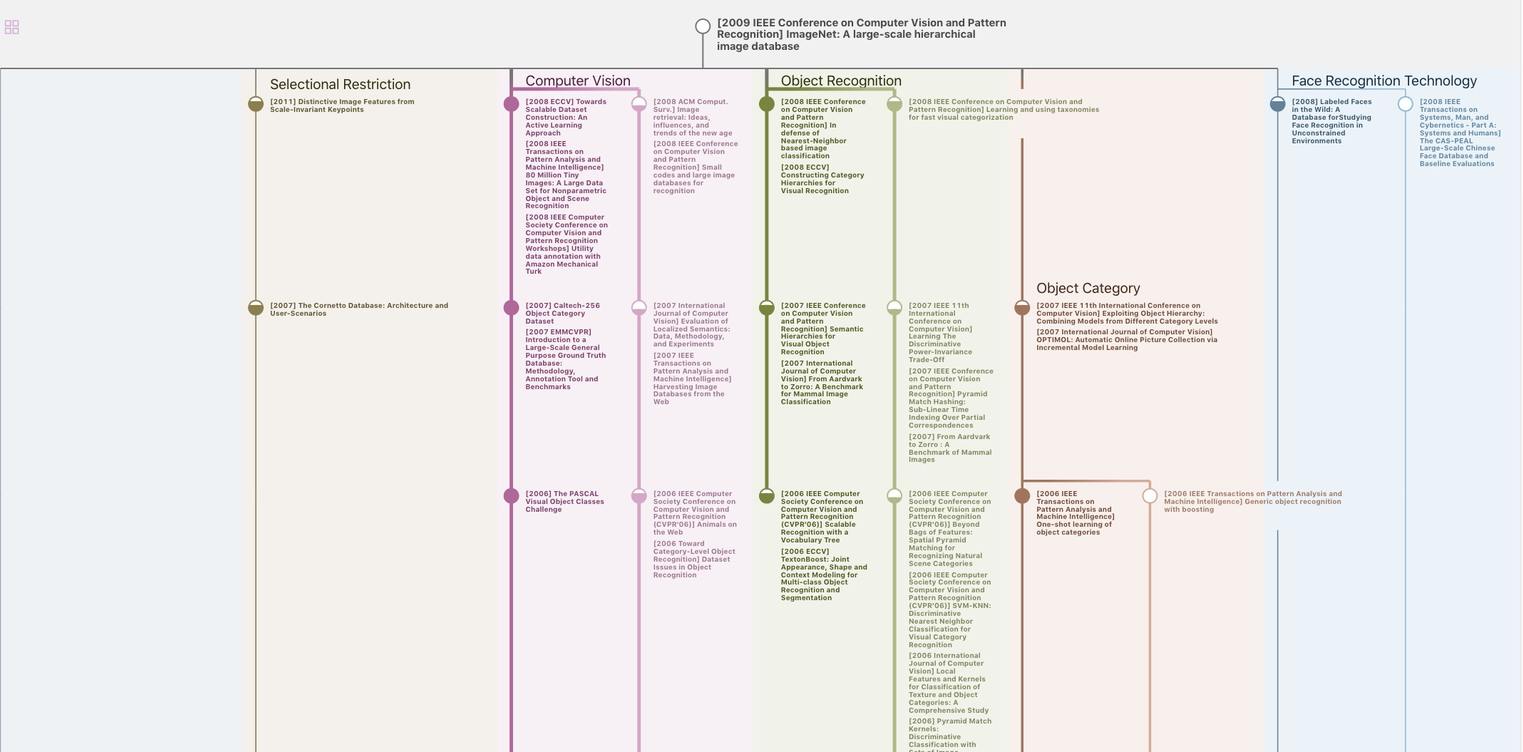
生成溯源树,研究论文发展脉络
Chat Paper
正在生成论文摘要