Exploring the Behavior-Driven Crash Risk Prediction Model: The Role of Onboard Navigation Data in Road Safety
JOURNAL OF ADVANCED TRANSPORTATION(2023)
摘要
Driving behavior has frequently been overlooked in previous road traffic crash research. Hereby, abnormal (extreme) driving behavior data transmitted by the onboard navigation systems were collected for vehicles involved in traffic crashes, including sharp-lane-change, sharp-acceleration, and sudden-braking behaviors. Using these data in conjunction with expressway crash records, multiple classification learners were trained to establish a behavior-driven risk prediction model. To further investigate the influence of driving behavior on crash risk, partial dependence plots (PDPs) were applied. Regression analyses indicate that models have a stronger effect when derivative features such as frequency of specific deviant behavior, speed, and acceleration in the behavior process are included. The behavioral RUSBoost model surpasses other models, achieving an AUC prediction metric of 0.782 and outperforming traditional traffic-flow-driven machine learning models. PDP analysis demonstrates that the sudden-braking behavior is the leading contributory factor of expressway crashes, particularly when the acceleration exceeds 0.5 G. This study confirms the potential of predicting crash risks through augmenting behavior data from navigation software; the findings lay a foundation for countermeasures.
更多查看译文
AI 理解论文
溯源树
样例
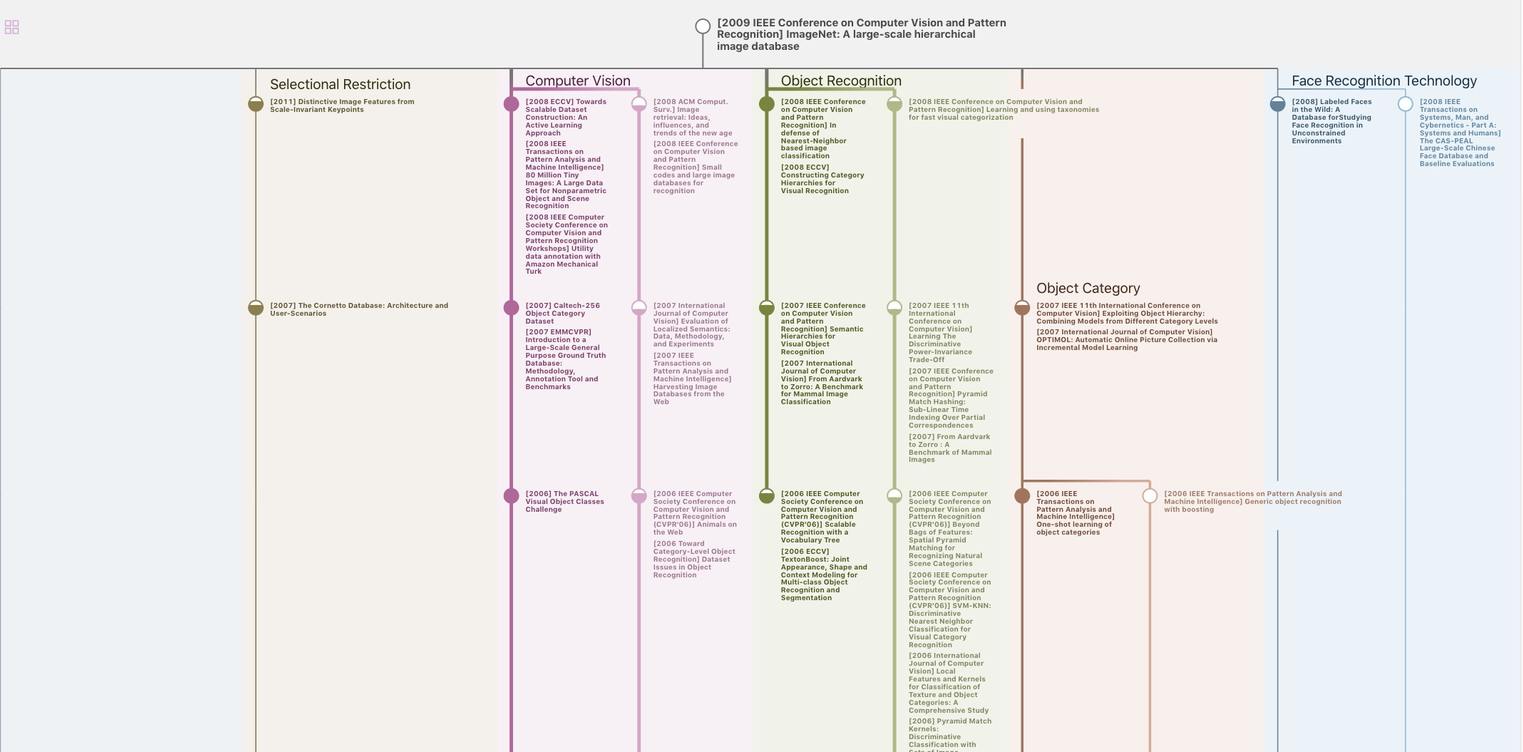
生成溯源树,研究论文发展脉络
Chat Paper
正在生成论文摘要