Forecasting energy power consumption using federated learning in edge computing devices Eduardo de Moraes Sarmento Iran Freitas Ribeiro
INTERNET OF THINGS(2024)
摘要
Several studies in the literature propose using machine learning algorithms to forecast consumers' energy consumption. However, such data is sensitive and has privacy constraints. On the other hand, federated learning is a technique in which the training of machine learning algorithms is performed locally, where the data is generated. In this context, this article presents a hybrid neural network architecture, named CNN-LSTM FED, trained using the public Smart* and The Building Data Genome Project 2 datasets. Additionally, an augmented Smart* dataset was generated using Generative Adversarial Networks (GANs). The performance of CNN-LSTM FED was evaluated by comparing it against the MultiLayer Perceptron (MLP), which serves as a baseline, and against a non-federated version of the CNN-LSTM FED, named CNN-LSTM. Our approach was able to generalize the model even when less than 1% of buildings participated in the modeling process, forecasting with good results the energy consumption of other buildings. Furthermore, the deployment of this architecture in an edge computing device, with limited computational resources for training, is evaluated.
更多查看译文
关键词
Federated learning,Forecasting energy consumption,Privacy requirements,Edge computing,GANs,Dataset augmentation
AI 理解论文
溯源树
样例
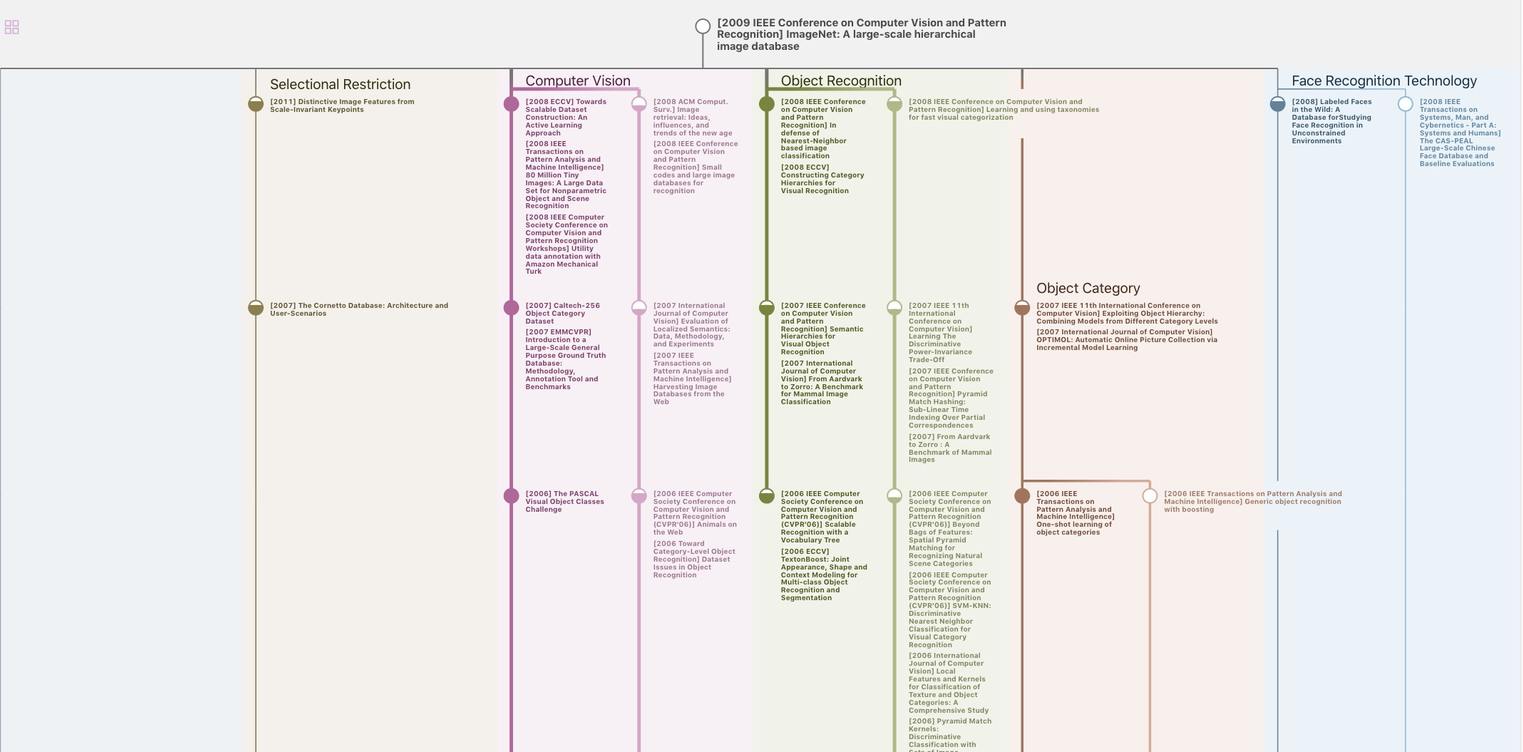
生成溯源树,研究论文发展脉络
Chat Paper
正在生成论文摘要