Tissue-specific RNA methylation prediction from gene expression data using sparse regression models
COMPUTERS IN BIOLOGY AND MEDICINE(2024)
摘要
N6-methyladenosine (m6A) is a highly prevalent and conserved post -transcriptional modification observed in mRNA and long non -coding RNA (lncRNA). Identifying potential m6A sites within RNA sequences is crucial for unraveling the potential influence of the epitranscriptome on biological processes. In this study, we introduce Exp2RM, a novel approach that formulates single -site -based tissue -specific elastic net models for predicting tissue -specific methylation levels utilizing gene expression data. The resulting ensemble model demonstrates robust predictive performance for tissue -specific methylation levels, with an average R -squared value of 0.496 and a median R -squared value of 0.482 across all 22 human tissues. Since methylation distribution varies among tissues, we trained the model to incorporate similar patterns, significantly improves accuracy with the median Rsquared value increasing to 0.728. Additonally, functional analysis reveals Exp2RM's ability to capture coefficient genes in relevant biological processes. This study emphasizes the importance of tissue -specific methylation distribution in enhancing prediction accuracy and provides insights into the functional implications of methylation sites.
更多查看译文
关键词
Epitranscriptome,Tissue-specific methylation status,Human methylome distribution,Methylation level prediction,Elastic net regression
AI 理解论文
溯源树
样例
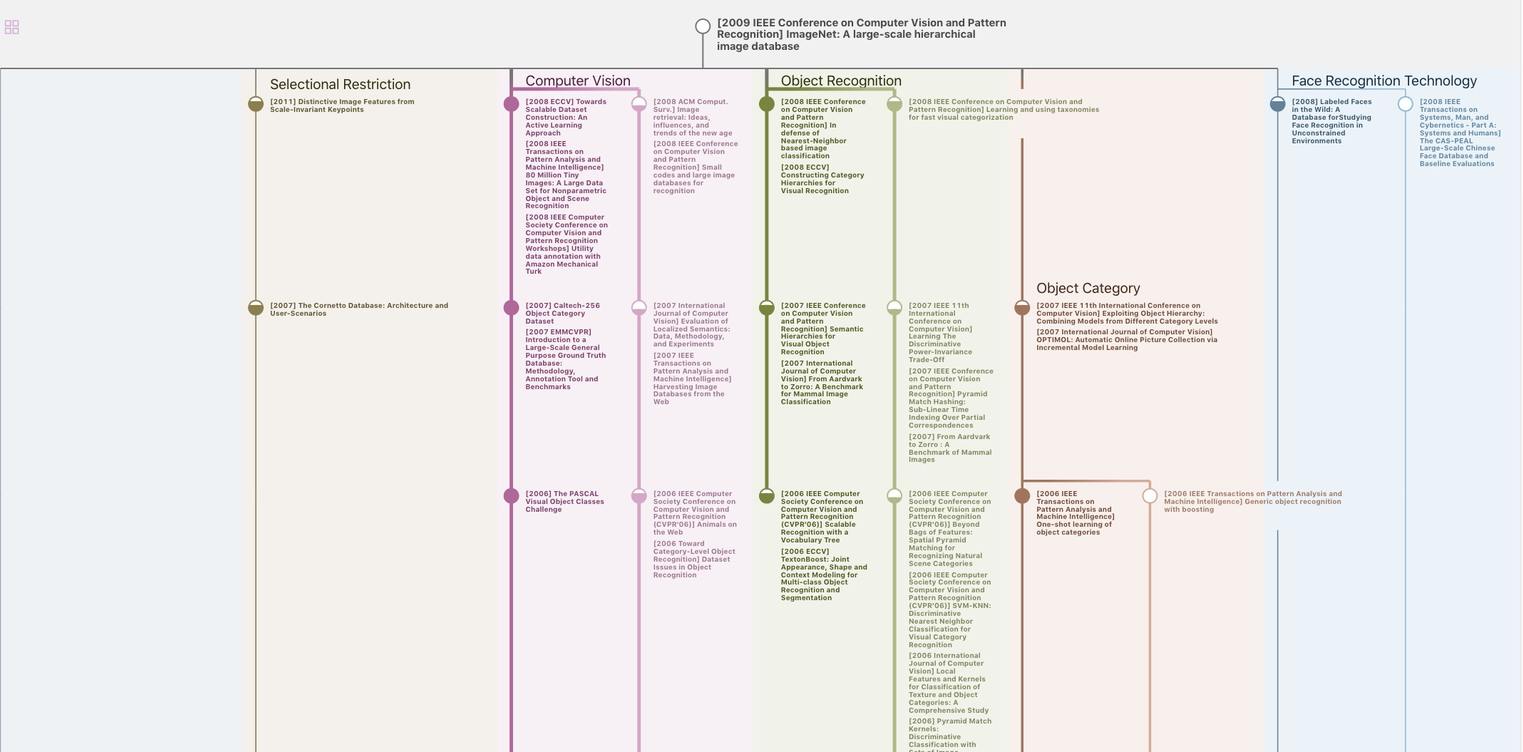
生成溯源树,研究论文发展脉络
Chat Paper
正在生成论文摘要