Semantic perceptive infrared and visible image fusion Transformer
PATTERN RECOGNITION(2024)
摘要
Deep learning based fusion mechanisms have achieved sophisticated performance in the field of image fusion. However, most existing approaches focus on learning global and local features but seldom consider to modeling semantic information, which might result in inadequate source information preservation. In this work, we propose a semantic perceptive infrared and visible image fusion Transformer (SePT). The proposed SePT extracts local feature through convolutional neural network (CNN) based module and learns long-range dependency through Transformer based modules, and meanwhile designs two semantic modeling modules based on Transformer architecture to manage high-level semantic information. One semantic modeling module maps the shallow features of source images into deep semantic, the other learns the deep semantic information in different receptive fields. The final fused results are recovered from the combination of local feature, long-range dependency and semantic feature. Extensive comparison experiments demonstrate the superiority of SePT compare to other advanced fusion approaches.
更多查看译文
关键词
Infrared image,Visible image,Transformer,Long-range dependency,Local feature,Semantic perceptive,Image fusion
AI 理解论文
溯源树
样例
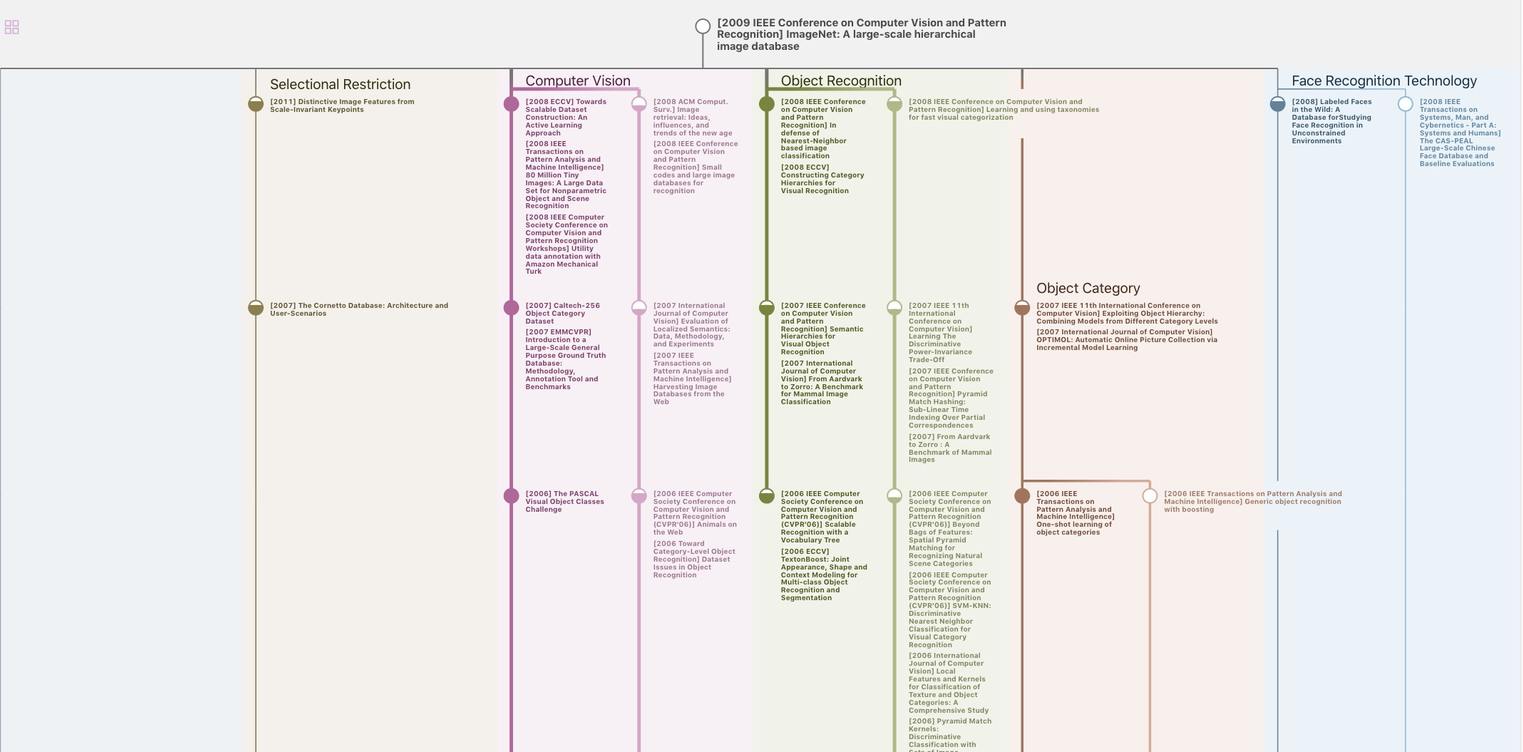
生成溯源树,研究论文发展脉络
Chat Paper
正在生成论文摘要