Comparative Analysis of LSTM and Ensemble LSTM Approaches for Gene Mutation Classification in Cancer
2023 IEEE INTERNATIONAL CONFERENCE ON MACHINE LEARNING AND APPLIED NETWORK TECHNOLOGIES, ICMLANT(2023)
摘要
In this study, we present an in-depth comparison of five different deep learning approaches for the classification of gene mutations based on a dataset provided by the Kaggle competition "Personalized Medicine: Redefining Cancer Treatment." The models compared include a Long Short-Term Memory (LSTM) model, an ensemble of LSTM and Bidirectional LSTM (BiLSTM), an ensemble of LSTM and 1-Dimensional Convolutional Neural Network (1D-CNN), an ensemble of LSTM and Gated Recurrent Unit (GRU), and a multi-ensemble model combining LSTM, BiLSTM, 1D-CNN, and GRU. These models were evaluated on several metrics including accuracy, precision, recall, F1 score, and mean squared error (MSE) for both the training and validation sets. Among all the models, the LSTM + 1D-CNN ensemble model demonstrated superior performance on the validation set while also being the most time-efficient model to train. These results contribute to the growing body of research in the field of personalized medicine and highlight the efficacy of deep learning ensemble models in the classification of gene mutations, which could play a vital role in future cancer treatment strategies.
更多查看译文
关键词
Gene Mutation Classification,Text Classification,Long Short-Term Memory
AI 理解论文
溯源树
样例
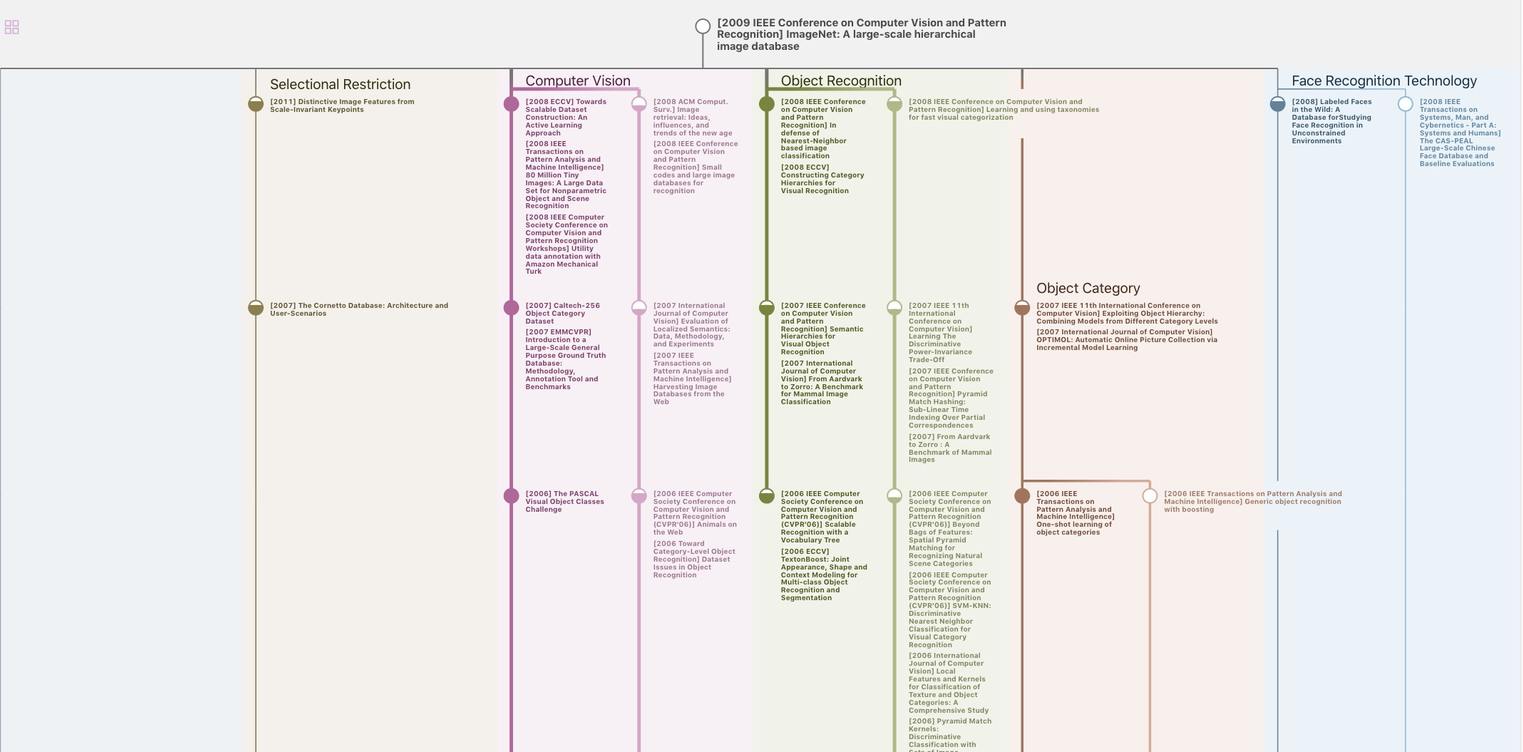
生成溯源树,研究论文发展脉络
Chat Paper
正在生成论文摘要