Parallel Compared-and-Stacked Pyramid Transformer Network for Unsupervised Hyperspectral Change Detection
IEEE TRANSACTIONS ON GEOSCIENCE AND REMOTE SENSING(2024)
摘要
Convolutional neural networks (CNNs) with good feature learning capabilities are widely used in hyperspectral image change detection (HSI-CD) tasks. However, most existing CNN-based HSI-CD methods face two inherent challenges: 1) the lack of available labeled datasets and 2) the limited receptive field that cannot capture the long-distance dependence between the spectral sequences of HSIs. In this article, we propose a parallel compared-and-stacked pyramid transformer network (PCPTNet) for unsupervised HSI-CD, which can model the context information of spectral sequences in the input multi-temporal HSI patch without real labeled data. Specifically, a superpixel-level joint decision-based training samples selection strategy is presented that fully considers the correlation between pixels to improve the reliability of training samples. Then, taking advantage of transformer in context information modeling, PCPTNet is proposed to capture sufficient difference features and stacked features with different scales for CD, which can effectively reduce missed and false detection. The multiscale features containing sufficient low-level detail information and high-level semantic features are fused hierarchically to classify changed and unchanged pixels. Extensive experiments on three real HSI datasets demonstrate that the PCPTNet outperforms other state-of-the-art HSI-CD methods in both visual and quantitative results.
更多查看译文
关键词
Feature extraction,Transformers,Correlation,Training,Task analysis,Deep learning,Context modeling,Hyperspectral image change detection (HSI-CD),joint decision,pyramid,transformer,unsupervised
AI 理解论文
溯源树
样例
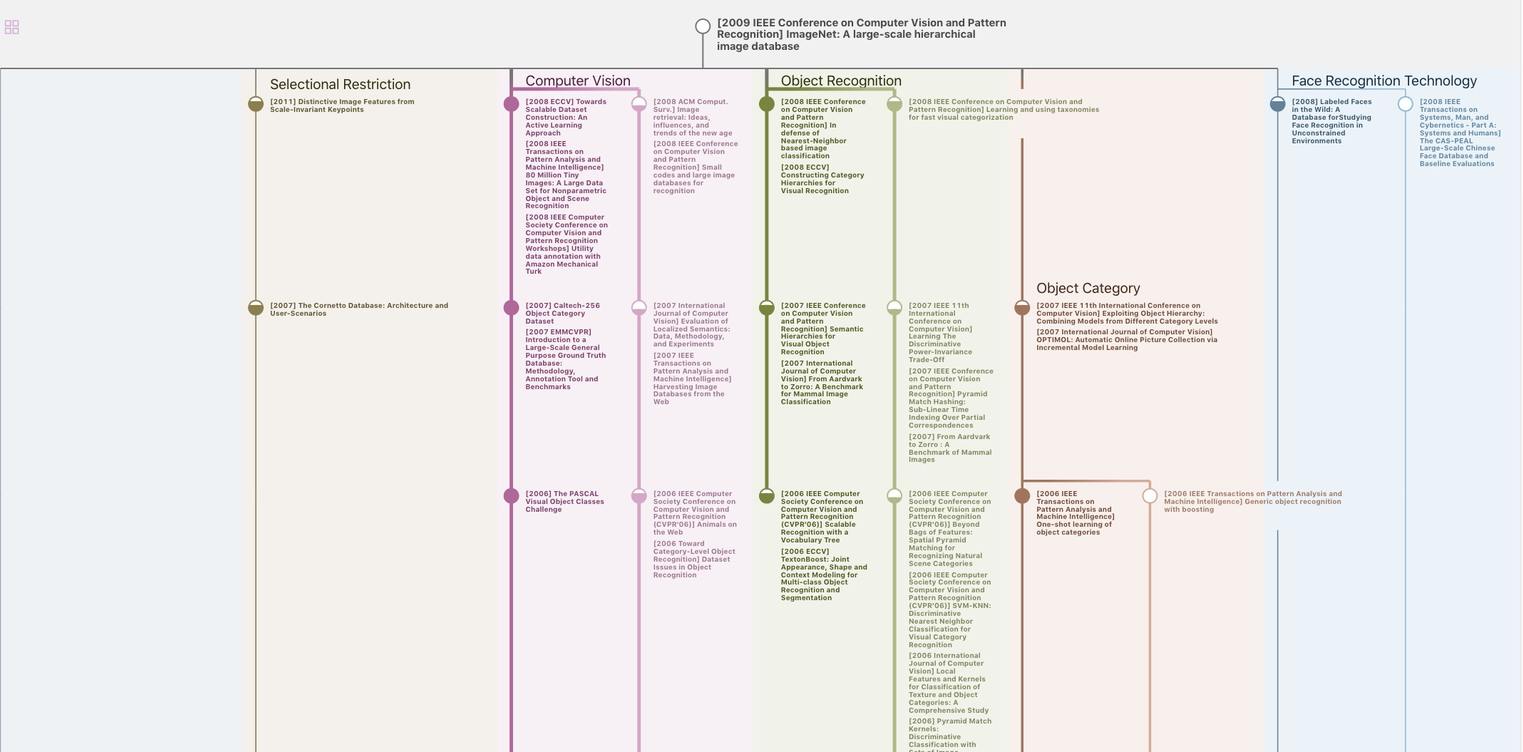
生成溯源树,研究论文发展脉络
Chat Paper
正在生成论文摘要