Adaptive Texture and Spectrum Clue Mining for Generalizable Face Forgery Detection
IEEE TRANSACTIONS ON INFORMATION FORENSICS AND SECURITY(2024)
摘要
Although existing face forgery detection methods achieve satisfactory performance under closed within-dataset scenario where training and testing sets are created by the same manipulation technique, they are vulnerable to samples created by unseen manipulation techniques under cross-dataset scenario. To solve this problem, in this work, we propose a novel adaptive texture and spectral clue mining (ATSC) approach for generalizable face forgery detection. It adaptively adjusts the parameters depended on input images to mine specific intrinsic forgery clues on both spatial and frequency domains. Specifically, ATSC customizes a Texture Clue Mining Module and a Spectrum Clue Selecting Module. The former module exploits instance-aware dynamic convolution on spatial domain to dynamically assemble multiple parallel convolutional kernels based on the learned image-dependent attention maps for effectively capturing subtle texture artifacts on spatial domain. A customized attention loss is also applied to the attention maps as supervision to precisely localize forgery artifacts whilst retain useful background information from suspicious and non-suspicious regions, which drives the module to explore all potential crucial clues and learning robust texture-related forgery feature. Moreover, the latter module applies adaptive frequency filtering mechanism on DCT-based frequency signals, which selects frequency information of interest to capture refined spectrum clues on frequency domain in an input-adaptive manner. Equipped with the above two modules, ATSC can learn more generalizable forgery features for face forgery detection. Extensive experimental results demonstrate the superior generalization ability of the proposed ATSC over various state-of-the-art methods on the challenging benchmarks.
更多查看译文
关键词
Face forgery detection,domain generalization,adaptive learning
AI 理解论文
溯源树
样例
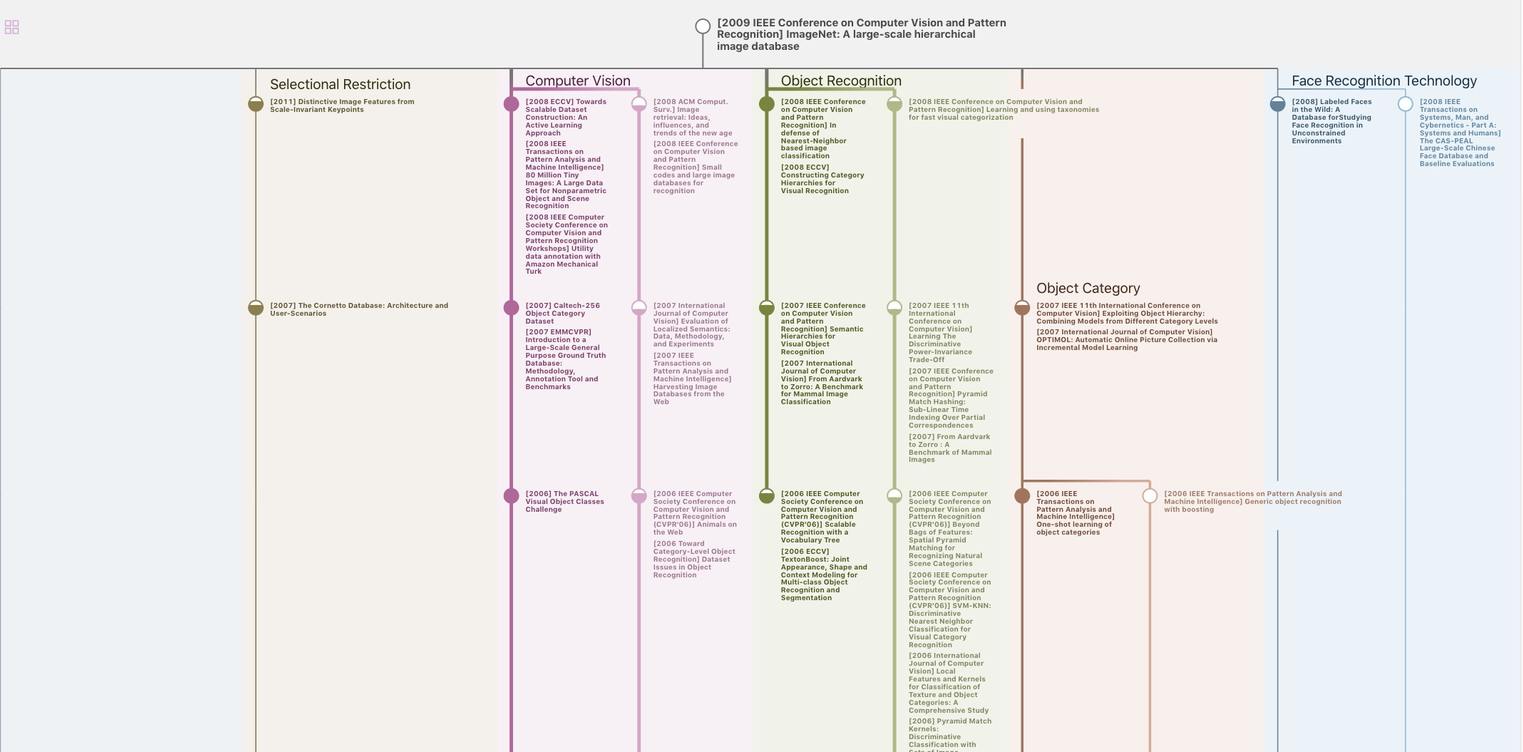
生成溯源树,研究论文发展脉络
Chat Paper
正在生成论文摘要