Convergence Improvement by Parameters Exchange in Asynchronous Decentralized Federated Learning for Non-IID Data.
2023 49th Euromicro Conference on Software Engineering and Advanced Applications (SEAA)(2023)
摘要
Asynchronous decentralized federated learning is a promising distributed machine learning framework from the viewpoint of the server cost saving and communication bottleneck mitigation as well as privacy and security protection. Although several methods have been proposed for asynchronous decentralized federated learning, most existing works have a problem of performance degradation for non-independent and identically distributed (non-IID) data. Even the methods aimed for non-IID data do not achieve high accuracy or fast convergence for highly non-IID data in sparse network topologies. To address this issue, we propose a new parameters exchange approach "Skip" and employ it in combination with an existing approach "Swap" to let the distributed models efficiently learn non-local data. Then, we propose two novel asynchronous decentralized federated learning methods, Greedy-Skip & Swap SGD (GSS SGD) and Topology-aware-Skip & Swap SGD (TSS SGD), by combining Skip and Swap in a topology-agnostic and topology-aware fashion, respectively. Our evaluation demonstrated that our TSS SGD outperforms existing methods for highly non-IID data, in terms of the inference accuracy and convergence speed, regardless of the sparsity of topologies.
更多查看译文
关键词
Asynchronous decentralized federated learning,Non-IID data,Stochastic gradient descent
AI 理解论文
溯源树
样例
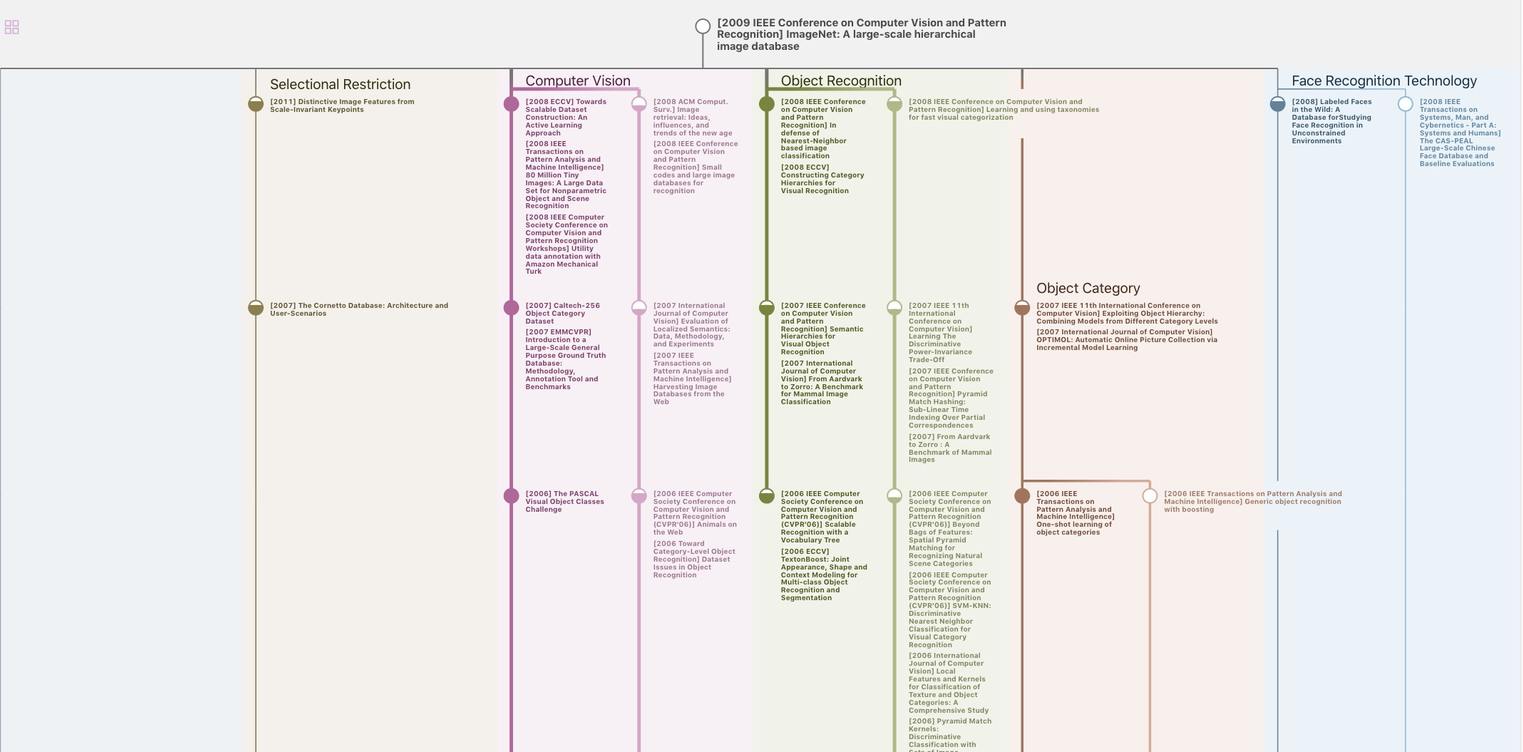
生成溯源树,研究论文发展脉络
Chat Paper
正在生成论文摘要