Multi-Dataset Human Activity Recognition: Leveraging Fusion for Enhanced Performance
2023 IEEE 20th International Conference on Smart Communities: Improving Quality of Life using AI, Robotics and IoT (HONET)(2023)
摘要
This paper explores the domain of Human Activity Recognition (HAR) utilizing sensor-based data and machine learning models. Deeply, the paper research two data fusion methods with the goal of building efficient model for activity recognition problem. two dataset have combined for the purpose of classification. We address a common challenge in HAR research. such as, Using different smartphone models and sensor configurations. To tackle this challenge, we conducts experiments on two datasets, KU-HAR and UCI HAR, and employs data fusion techniques at both feature and decision levels. Four popular machine learning classifiers-Decision Trees, Random Forest, Gradient Boosting, and XGBoost-are evaluated using these fusion methods. The results show promising performance in classifying fundamental human activities. XGBoost demonstrated the highest accuracy, surpassing all other models with approximately 96.83%. In comparison to XGBoost's accuracy, Gradient Boosting achieved an accuracy of about 95.71 %, demonstrating strong performance. Random Forest, while slightly behind, still delivered an acceptable accuracy of around 94.28 %, making it a viable choice for Human Activity Recognition tasks. decision tree has the lowest performance with 85.69 % In terms of decision-level fusion accuracy, Stacking outperformed the other methods with an accuracy of approximately 96.70%. Majority Voting also demonstrated strong performance, closely following with an accuracy of about 95.96%. However, Bagging fell behind the rest, achieving an accuracy of approximately 93.65 %.
更多查看译文
关键词
Feature Fusion,Time-Domain features,Sensors,Accelerometer,Gyroscope
AI 理解论文
溯源树
样例
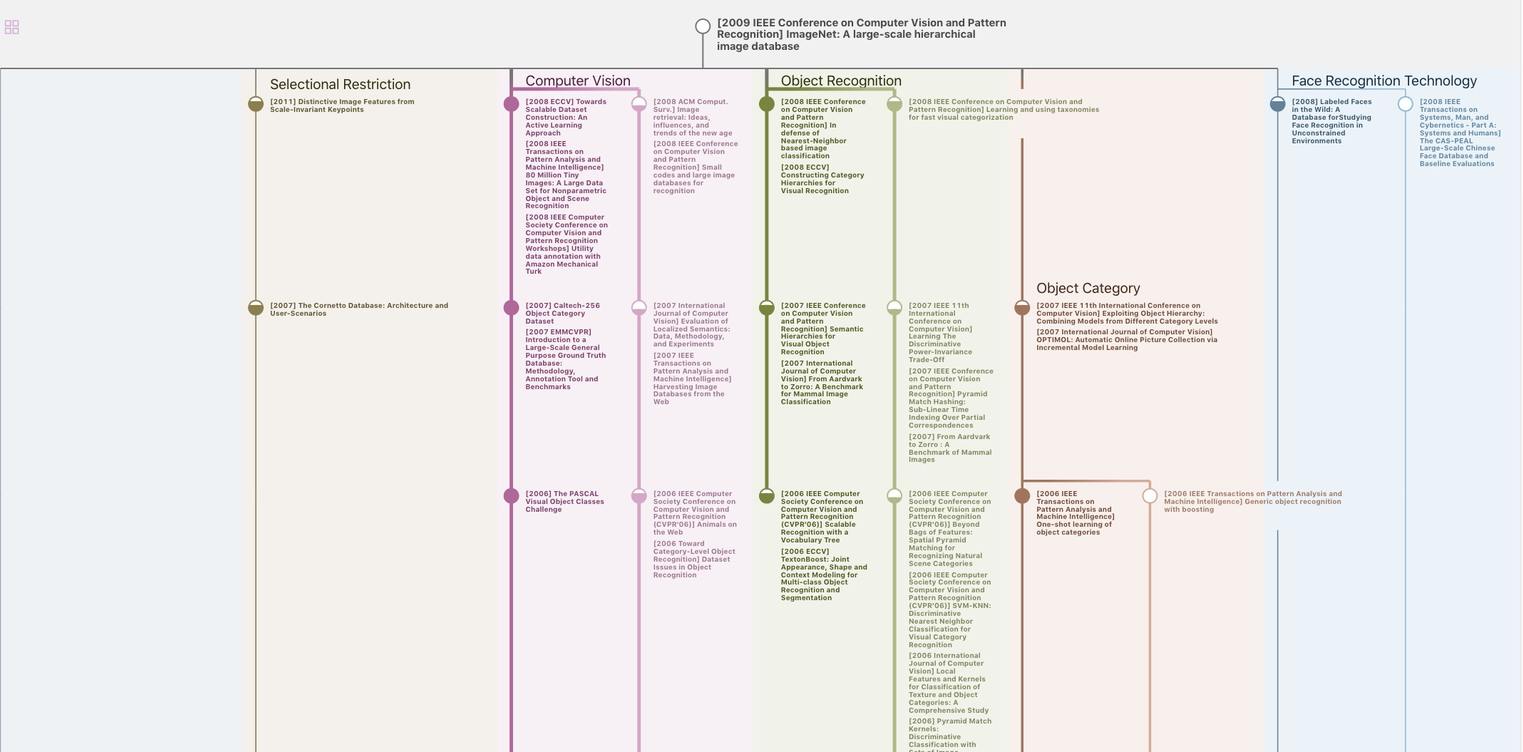
生成溯源树,研究论文发展脉络
Chat Paper
正在生成论文摘要