Algorithm Package of AI-Driven SDN Controller-Switch Load Balancing Strategies.
2023 IEEE Symposium Series on Computational Intelligence (SSCI)(2023)
摘要
Recently, Software-Defined Networking (SDN) is receiving much research attention due to its ability to decouple the data plane from the control architecture by associating the network switches to one (centralized) or more (distributed) controller(s). Traditionally, switches are assigned to the controllers in a static manner which results in under-utilization of the resources of the controllers and increased response delays to user requests. In this paper, we consider a practical load-balancing and agile scenario by formulating the dynamic associations of switches and controllers as an NP-hard optimization problem to minimize the maximum resource utilization of the controllers. Therefore, we propose an Ant Colony Optimization (ACO)-based algorithm to deal with the aforementioned request satisfiability issue in large SDN systems in polynomial-time. Furthermore, we envision a hybrid deep learning model consisting of Convolutional Neural Network (CNN) and Gated Recurrent Unit (GRU) structures to achieve near-optimal resource utilization for real-time SDN applications. Experimental results demonstrate that our customized CNN-GRU model outperforms the other techniques in terms of resource utilization (15% - 45% optimality gap) within a sianlficantly reduced computational running time (≤ 0.1s).
更多查看译文
关键词
Software-Defined Networking,Resource utilization,Convolutional Neural Network,Gated Recurrent Unit
AI 理解论文
溯源树
样例
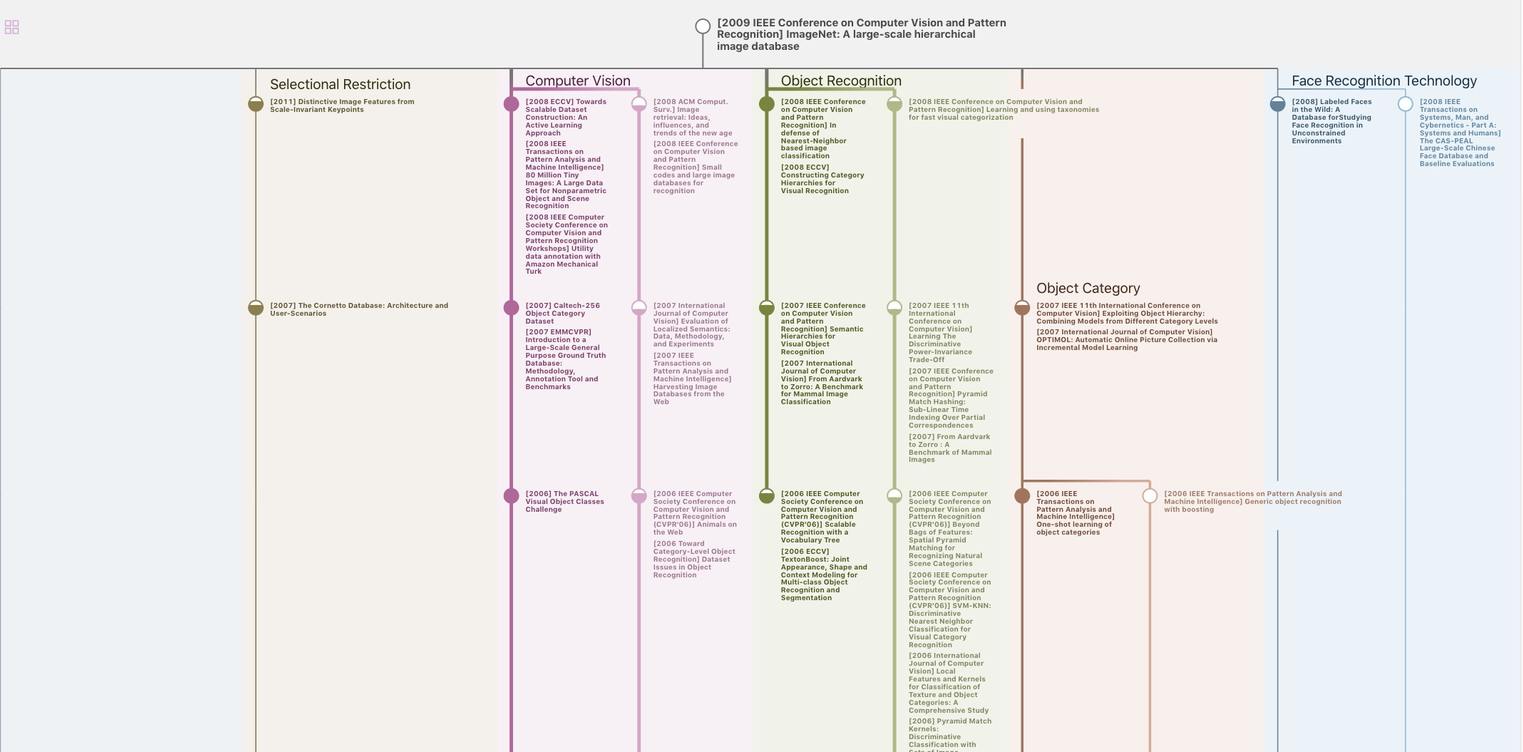
生成溯源树,研究论文发展脉络
Chat Paper
正在生成论文摘要