Competitive Reinforcement Learning Agents with Adaptive Networks
2023 11TH INTERNATIONAL CONFERENCE ON CONTROL, MECHATRONICS AND AUTOMATION, ICCMA(2023)
摘要
The depth of a neural network's architecture is a crucial decision that must balance network performance and the computational resources required during training and inference. In the context of Reinforcement Learning (RL), this architectural choice can profoundly impact the policy (i.e. agent's behavior) learned by the network. Depending on the state of the agent, different policies learned by the network may improve the agent's performance, particularly for time-sensitive applications (e.g. real-time, low-latency scenarios) when considering the additional computational time needed to access the output of deeper networks. Therefore, this paper proposes Greater Use of Time (GUT), a method that involves training multiple networks of different lengths and allowing them to make decisions collaboratively. If the shorter network is not confident enough, the longer network is relied on. For each network, the policy is learned through deep Q-learning, and the method's performance is evaluated in a competitive multi-agent environment. The results demonstrate that using multiple networks with different lengths not only reduces computational cost at inference time, but also yields significantly better performance than either the short or long network alone (p < 0.05). Importantly, the proposed use of confidence-based decision-making also significantly outperforms random decision-making (p < 0.05).
更多查看译文
关键词
Deep Reinforcement Learning,Adaptive Agents,Early-Exit Neural Networks,Network Selection
AI 理解论文
溯源树
样例
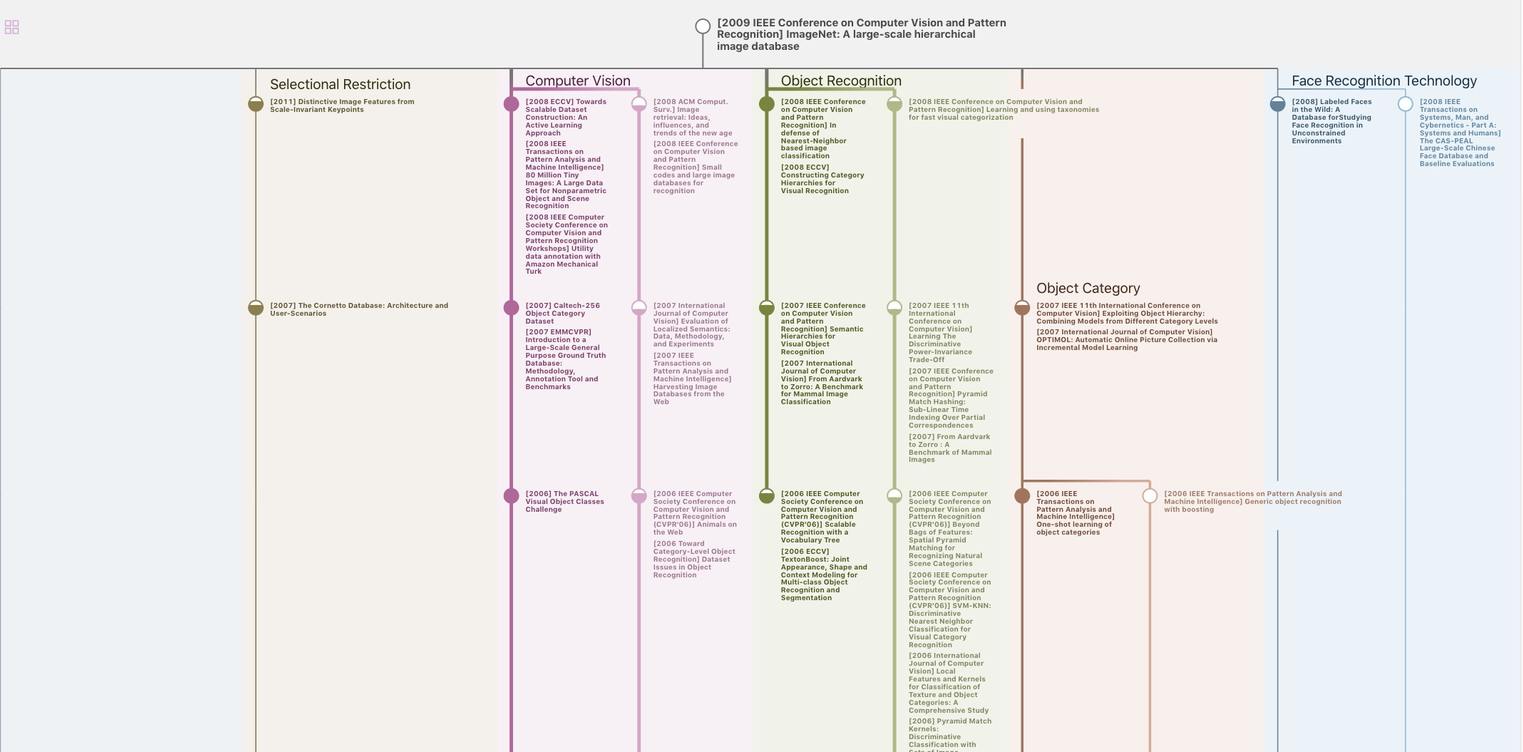
生成溯源树,研究论文发展脉络
Chat Paper
正在生成论文摘要