Reinforcement Learning-based Response Shaping Control of Dynamical Systems
2023 11TH INTERNATIONAL CONFERENCE ON CONTROL, MECHATRONICS AND AUTOMATION, ICCMA(2023)
摘要
The control system design specifications for dynamical systems are typically provided in terms of the desired transient response and steady-state response. Meeting such requirements for dynamical systems whose mathematical models are unavailable is a challenging task. In this paper, we propose a learning-based controller to achieve the desired control system design specifications for unknown dynamical systems. We consider a SoTA model-free reinforcement learning agent (TD3) in the continuous state and action space setting where the agent has no knowledge of system dynamics. The selection of an appropriate reward function is a key factor that shapes the response of the dynamical system when the learning-based controller is implemented. The resulting controller is trained and tested for first-order and second-order linear systems, as well as a nonlinear system. The resulting trajectories of the closed-loop systems indicate that the transient and steady-state response can be altered by choosing the appropriate reward function while adhering to the constraints imposed on the control input.
更多查看译文
关键词
model-free reinforcement learning,dynamical systems,error dynamics,reward shaping,trajectory tracking control
AI 理解论文
溯源树
样例
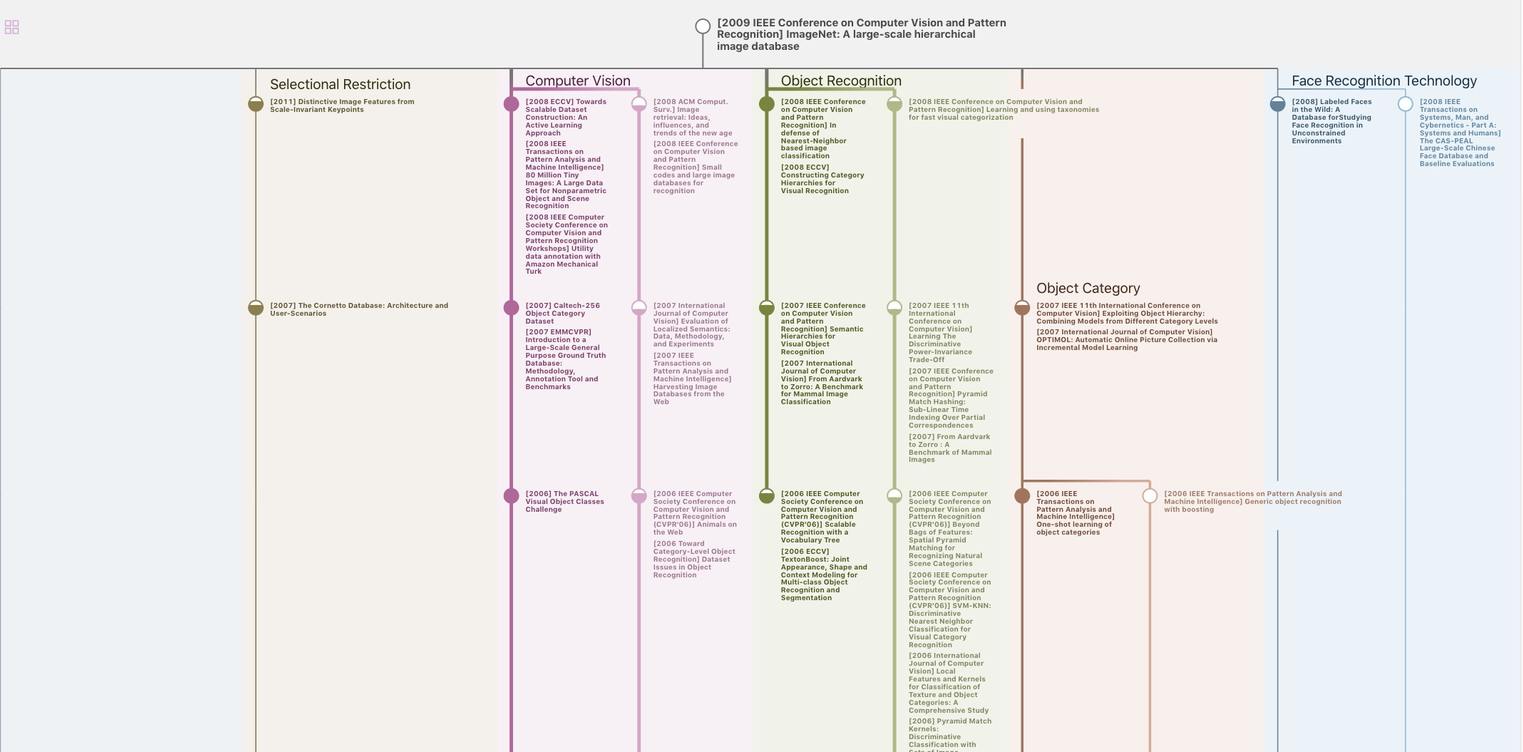
生成溯源树,研究论文发展脉络
Chat Paper
正在生成论文摘要