Enhancing Semiconductor Chip Image Defect Classification Using Deep Learning and Segmented Defect Region Information.
2023 IEEE International Conference on Cybernetics and Intelligent Systems (CIS) and IEEE Conference on Robotics, Automation and Mechatronics (RAM)(2023)
摘要
Due to the rapid development of deep learning networks in recent years, significant progress has been made in the field of semiconductor image processing. As semiconductor chips are the foundation of modern electronic technology and play an irreplaceable role in information storage, communication transmission, control systems, and other aspects, they are even widely used in fields such as medical care, energy, and environmental protection, making them one of the important engines driving social progress and technological development. However, there are many reasons why semiconductor chip image defects may occur, not only during the complex manufacturing process but also due to changes in temperature, humidity, and lighting. Therefore, it is necessary to control various factors during the manufacturing, transportation, and usage of semiconductor chips to minimize the possibility of defects. In order to promptly identify and solve problems, optimize the manufacturing process, avoid wasting materials and human resources due to manufacturing defects, reduce manufacturing costs, ensure the quality and yield of semiconductor chips, and ultimately reduce manufacturing costs, it is crucial to classify semiconductor chip image defects. However, traditional methods for semiconductor defect image classification have poor generalization ability and are not easy to apply to defect classification. To address this issue in complex scenarios, we propose a deep learning method that aggregates semiconductor image information with the segmented defect region information. By merging the information of the segmented image defect region, the model’s learning process is focused on the image area where defects occur, which can more effectively and accurately learn the classification and recognition of the semiconductor image condition, further improving the accuracy of image defect classification. Overall, our method significantly reduces the waste of human resources in defect classification and achieves more accurate and efficient judgment.
更多查看译文
关键词
Deep learning,Image classification,Segmentation-guided classification,Semiconductor imaging
AI 理解论文
溯源树
样例
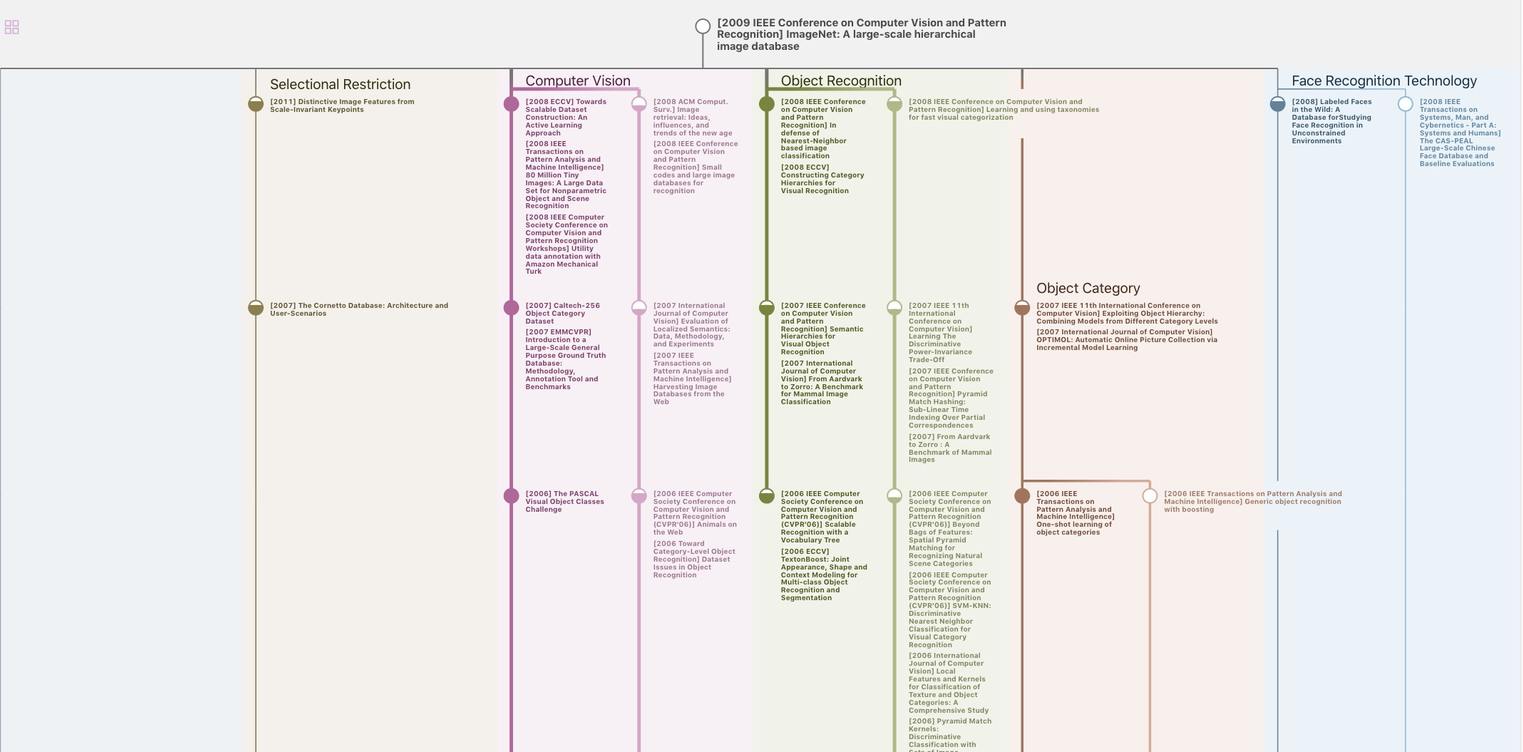
生成溯源树,研究论文发展脉络
Chat Paper
正在生成论文摘要