AutoML with Focal Loss for Defect Diagnosis and Prognosis in Smart Manufacturing.
2023 IEEE International Conference on Cybernetics and Intelligent Systems (CIS) and IEEE Conference on Robotics, Automation and Mechatronics (RAM)(2023)
摘要
Reliable defect diagnosis and prognosis for production lines are of great benefit in a range of advanced solutions towards smart manufacturing and yield optimization. However, inherent challenges exist, especially imbalanced data, missing value, and knowledge-related feature engineering. This study proposes a domain-specific automated machine learning (AutoML) method to self-learn the optimal models for defect diagnosis and prognosis. Firstly, a tree-based AutoML with focal loss (termed AutoGBM) is proposed based on XGBoost and Bayesian Optimization to improve imbalanced learning performance. Secondly, the AutoGBM system includes a hybrid feature learning mechanism based on fast feature filtering and recursive elimination, by which the key factors for defect diagnosis can be unveiled. Meanwhile, the proposed feature learning can identify the optimal interpolation preprocess to tackle missing data. Thirdly, based on Bayesian optimization, hyperparameter autotuning is conducted to generate the best prognostic results. Herein, SECOM data is used for test-bedding. Experimental results have demonstrated that AutoGBM is reliable and promising to diagnose key factors and predict defects in an automated way, as well as achieve adaptive model updates according to changes in manufacturing lines.
更多查看译文
关键词
Smart Manufacturing,Automated Machine Learning,XGBoost,Focal Loss
AI 理解论文
溯源树
样例
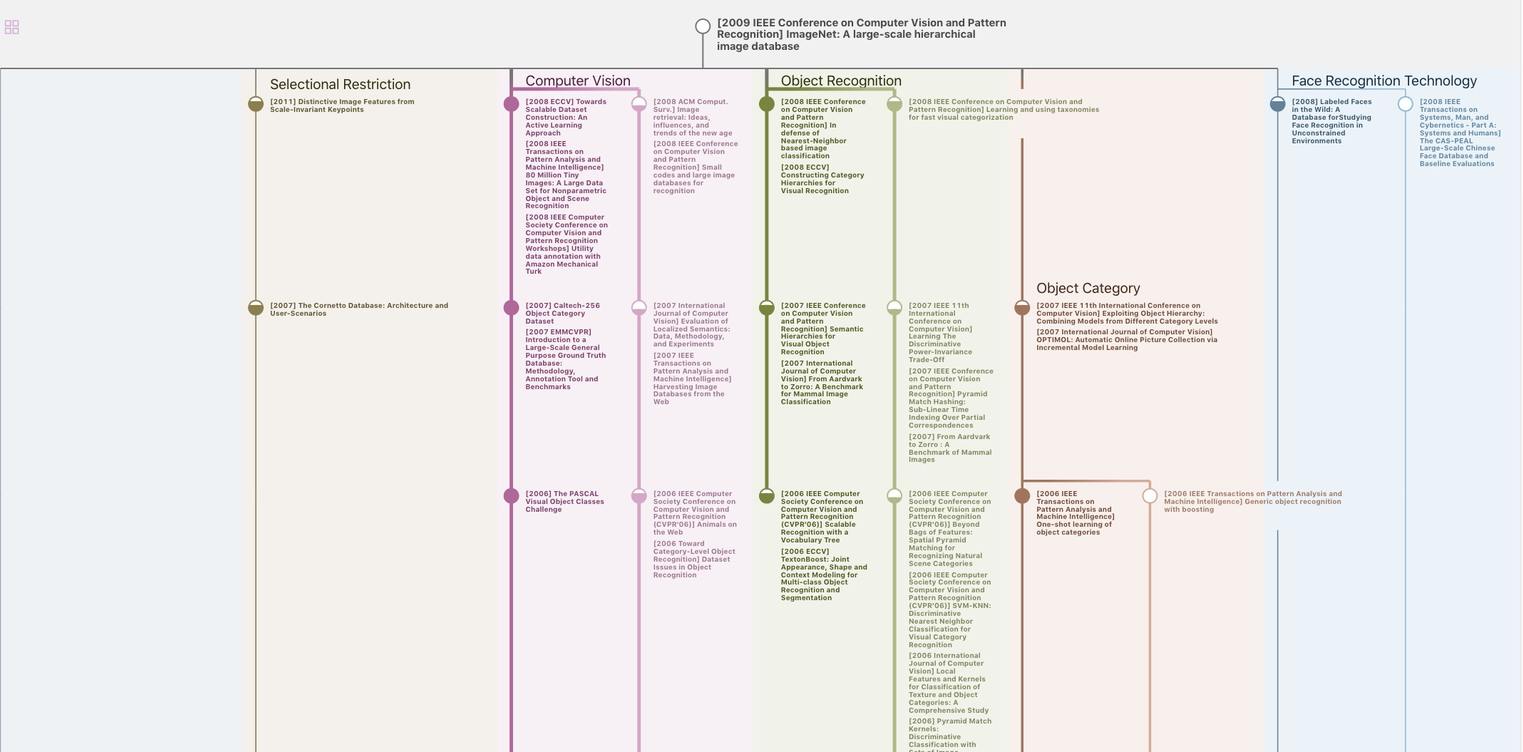
生成溯源树,研究论文发展脉络
Chat Paper
正在生成论文摘要