Enhancing Deep Neural Network Corruption Robustness using Evolutionary Algorithm.
2023 IEEE International Conference on Cybernetics and Intelligent Systems (CIS) and IEEE Conference on Robotics, Automation and Mechatronics (RAM)(2023)
摘要
A robust image classifier can model a classification function well and has subliminal impacts on classification performance when the input data is corrupted in some ways. Many popular classifiers based on deep neural networks (DNN) are trained using back-propagation (BP) that explicitly computes the gradient of a loss function. However, the BP-trained DNN shows poor performance on diverse types of image corruption. In this work, we propose the use of an evolutionary algorithm, differential evolution (DE), to evolve a population of BP pre-trained DNNs and examine whether the evolution improves DNNs’ classification robustness against various types of corruption. Specifically, pre-trained MobileNet and ResNet on CIFAR-100 and ImageNet are evolved using DE. Despite no change in network architecture and loss function, DE empirically enhances DNNs’ corruption robustness, in terms of mean Corruption Error (mCE), in almost all types of corruptions in CIFAR-100-C (18 out of 18 corruptions with ResNet-18) and Imagenet-C (18 out of 19 corruptions with MobileNetV2, except for impulse noise). The preliminary results and findings have implications for refining the robustness of BP-pre-trained DNNs.
更多查看译文
关键词
Differential evolution,corruption robustness,deep neural networks
AI 理解论文
溯源树
样例
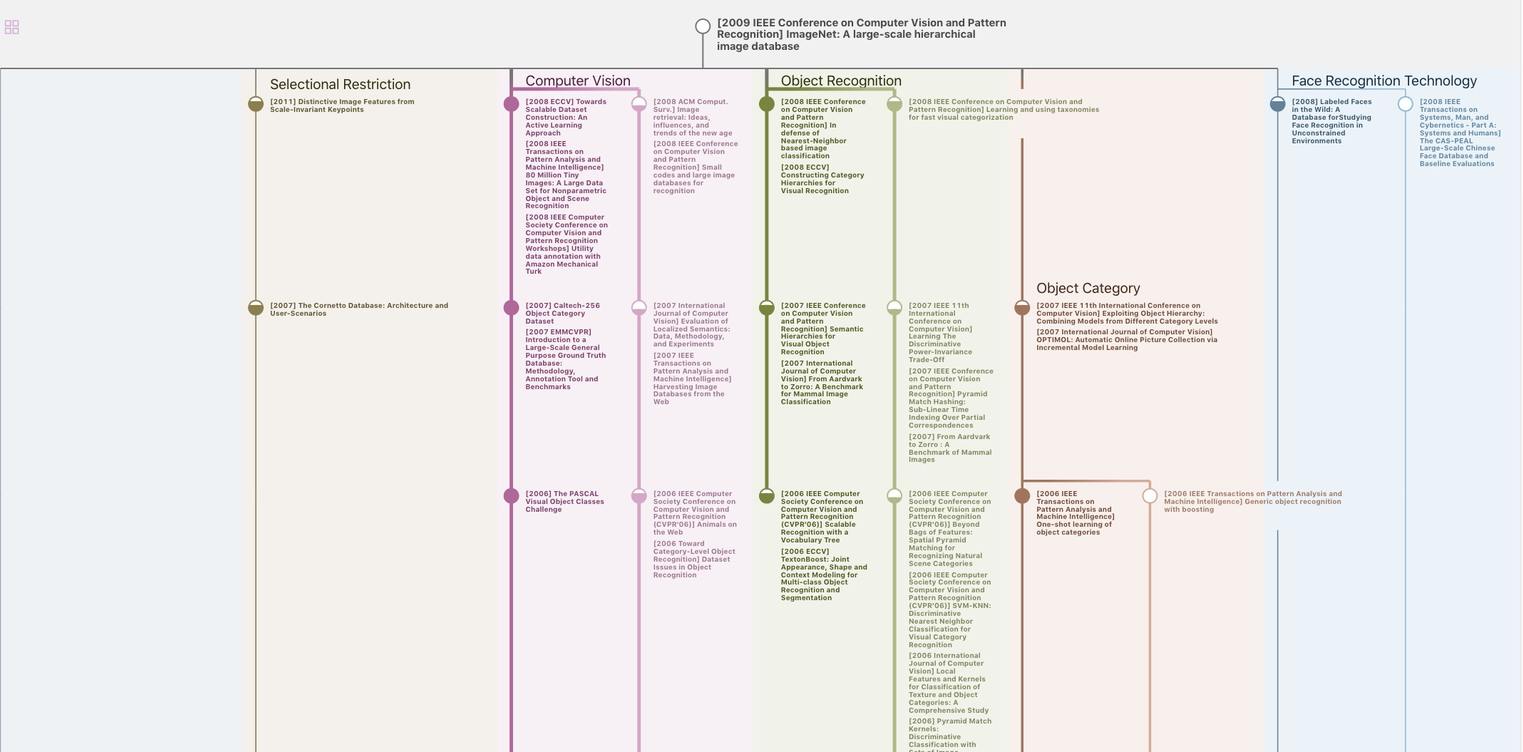
生成溯源树,研究论文发展脉络
Chat Paper
正在生成论文摘要