Ensemble based feature extraction and deep learning classification model with depth vision
COMPUTING AND INFORMATICS(2023)
摘要
It remains a challenging task to identify human activities from a video sequence or still image due to factors such as backdrop clutter, fractional occlu-sion, and changes in scale, point of view, appearance, and lighting. Different ap-pliances, as well as video surveillance systems, human-computer interfaces, and robots used to study human behavior, require different activity classification sys-tems. A four-stage framework for recognizing human activities is proposed in the paper. As part of the initial stages of pre-processing, video-to-frame con-version and adaptive histogram equalization (AHE) are performed. Additionally, watershed segmentation is performed and, from the segmented images, local tex-ton XOR patterns (LTXOR), motion boundary scale-invariant feature transforms (MoBSIFT) and bag of visual words (BoW) based features are extracted. The Bidirectional gated recurrent unit (Bi-GRU) and the Bidirectional long short-term memory (Bi-LSTM) classifiers are used to detect human activity. In addition, the combined decisions of the Bi-GRU and Bi-LSTM classifiers are further fused, and their accuracy levels are determined. With this Dempster-Shafer theory (DST) technique, it is more likely that the results obtained from the analysis are ac-curate. Various metrics are used to assess the effectiveness of the deployed ap-proach.
更多查看译文
关键词
Human activities,improved LTXOR,BoW,Bi-LSTM,Bi-GRU classi-fier
AI 理解论文
溯源树
样例
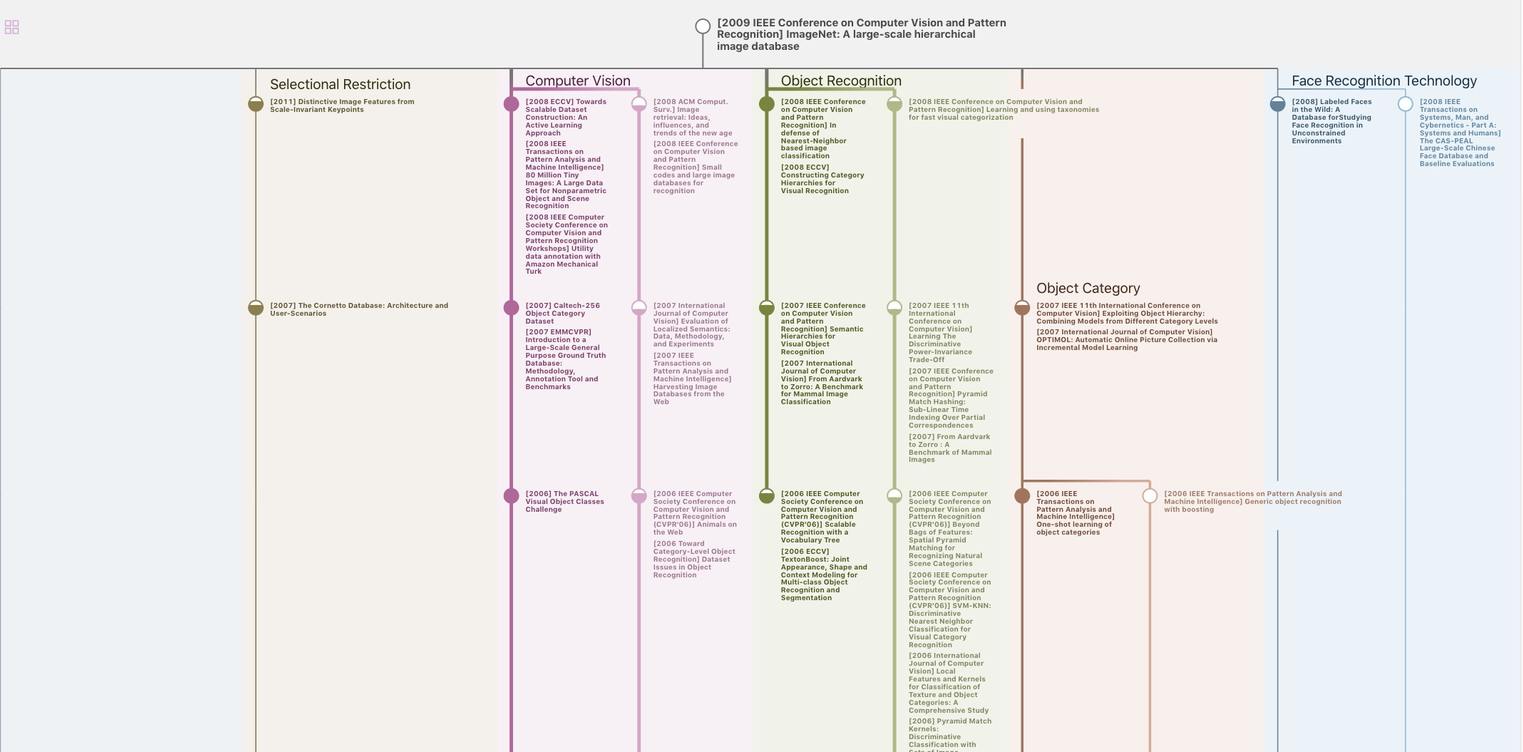
生成溯源树,研究论文发展脉络
Chat Paper
正在生成论文摘要