Deep HarDec: Deep Neural Network Applied to Estimate Harmonic Decomposition
ENERGY INFORMATICS, EI.A 2023, PT I(2024)
摘要
A Deep Harmonic Decomposition (Deep HarDec) approach is proposed in this paper, being developed by means of a deep neural network, allowing to obtain estimations of the amplitude and phase quantities of a given periodic signal. Consequently, harmonic characterization of periodic signals are explored in this paper, assessing the suitability of the Deep HarDec. Such a method can be potentially applied to the real-time management of electric power systems as well as other control applications, supporting the monitoring of harmonic distortions and providing means to active filtering interventions targeting power quality improvement. In order to build the Deep HarDec model, a dataset comprising diverse combinations of the fifth, seventh, eleventh, and thirteenth harmonic orders was considered, covering a wide range of operational perspectives. A grid search technique was used to find the best configuration for the multi-layer perceptron adopted for the approach, and the deep neural network was subjected to a training procedure targeting the harmonic estimation. A study case focusing on a selective active filtering application demonstrates that the Deep HarDec can effectively decompose harmonics, supporting the synthesis of real-time compensation references to tackle harmonic distortions in an electric grid.
更多查看译文
关键词
artificial neural networks,active power filter,deep learning,harmonic decomposition,harmonic estimation,multi-layer perceptron,power quality
AI 理解论文
溯源树
样例
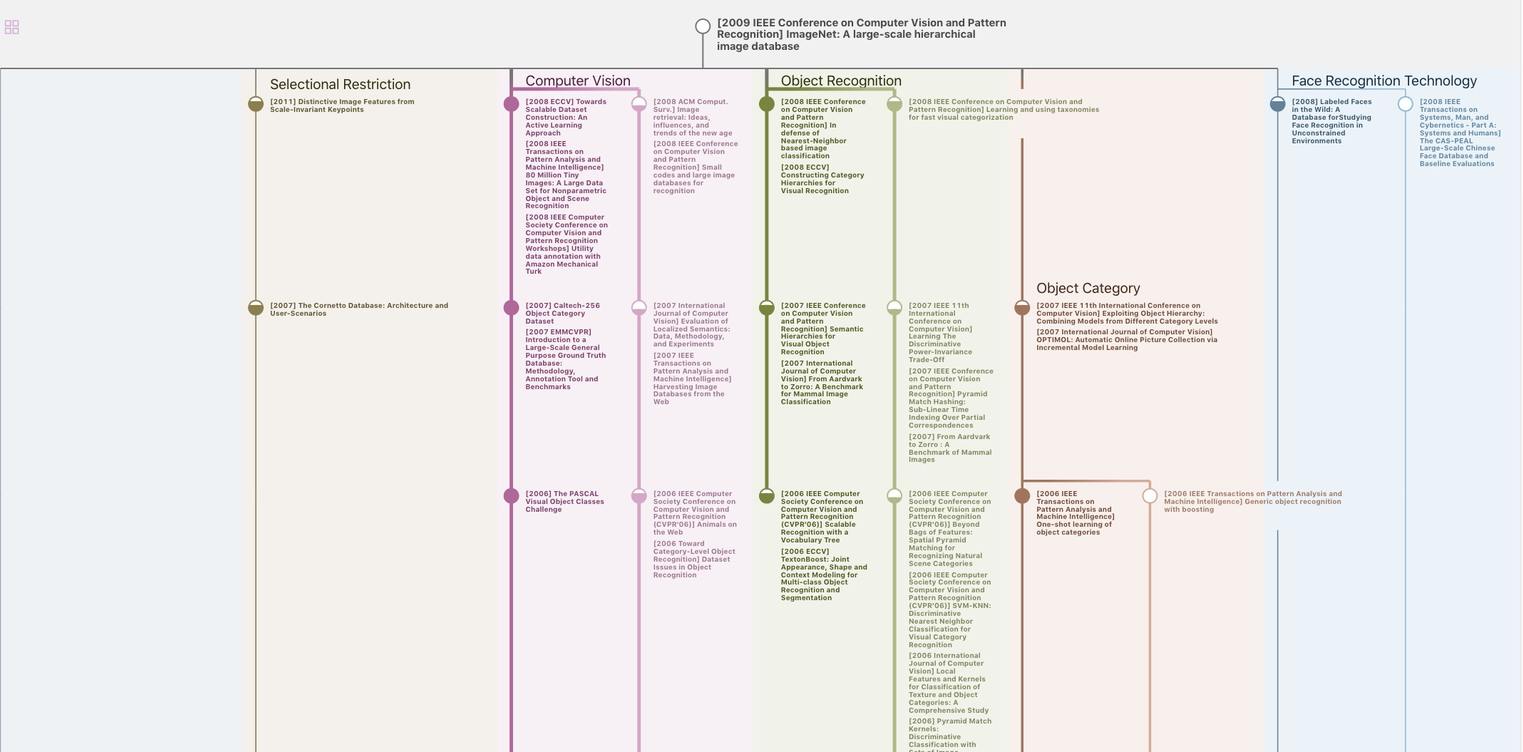
生成溯源树,研究论文发展脉络
Chat Paper
正在生成论文摘要