Comparing Manual vs Automatic Tuning of Differential Evolution Strategies for Energy Resource Management Optimization
ENERGY INFORMATICS, EI.A 2023, PT I(2024)
摘要
The energy resource management problem in energy systems is hard to optimize, mainly due to non-linear restrictions and a large number of variables involved. This is partly because of the increased integration of distributed energy resources. Computational intelligence optimization techniques, namely evolutionary algorithms, are regarded as efficient techniques for identifying optimal and near-optimal solutions. However, these algorithms usually have in their design several parameters that need to be set and, in most cases, tuned for a given problem to find good solutions. This work proposes an automatic configuration approach of different differential evolution strategies using the irace package to solve a centralized day-ahead energy resource management problem. The problem considers an aggregator managing multiple resources, such as renewable generation, battery energy systems, electric vehicles, and loads with demand response capabilities. The aggregator aims to minimize operational costs and maximize revenues to obtain a profit. We compare the results of a "manual" tuning of parameters with the results obtained with the auto-tuned parameters using irace. Results show that the automatic configuration improves the profits of the aggregator in almost all strategies (except for DE/either-or-algorithm/1), getting the best results, an improvement of around 7%, with the automatically tuned DE/target-to-best/1 mutation strategy.
更多查看译文
关键词
Automatic tuning,Differential evolution,Energy resource management,Evolutionary algorithms,Iterated racing,Optimization
AI 理解论文
溯源树
样例
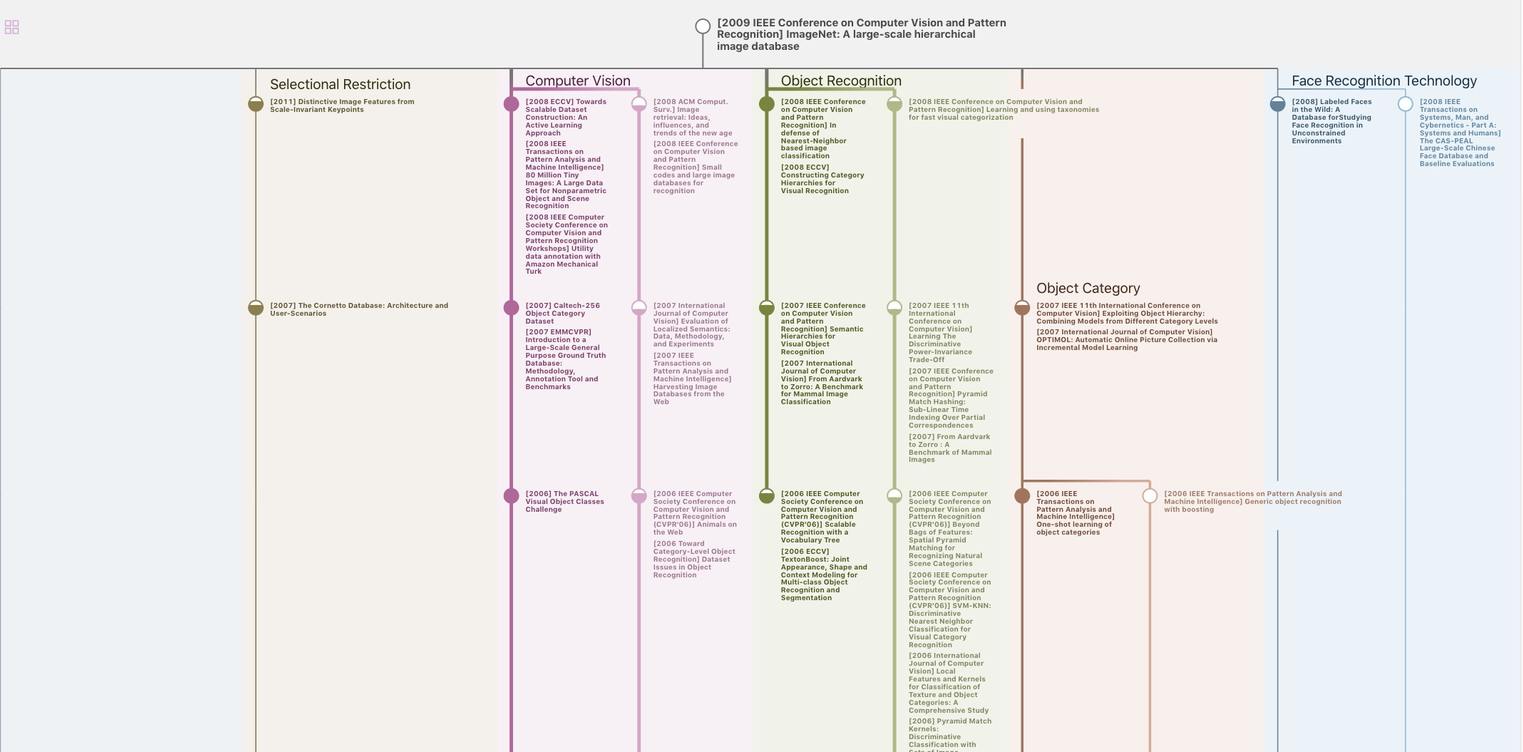
生成溯源树,研究论文发展脉络
Chat Paper
正在生成论文摘要