6D-Diff: A Keypoint Diffusion Framework for 6D Object Pose Estimation
CoRR(2023)
摘要
Estimating the 6D object pose from a single RGB image often involves noise
and indeterminacy due to challenges such as occlusions and cluttered
backgrounds. Meanwhile, diffusion models have shown appealing performance in
generating high-quality images from random noise with high indeterminacy
through step-by-step denoising. Inspired by their denoising capability, we
propose a novel diffusion-based framework (6D-Diff) to handle the noise and
indeterminacy in object pose estimation for better performance. In our
framework, to establish accurate 2D-3D correspondence, we formulate 2D
keypoints detection as a reverse diffusion (denoising) process. To facilitate
such a denoising process, we design a Mixture-of-Cauchy-based forward diffusion
process and condition the reverse process on the object features. Extensive
experiments on the LM-O and YCB-V datasets demonstrate the effectiveness of our
framework.
更多查看译文
AI 理解论文
溯源树
样例
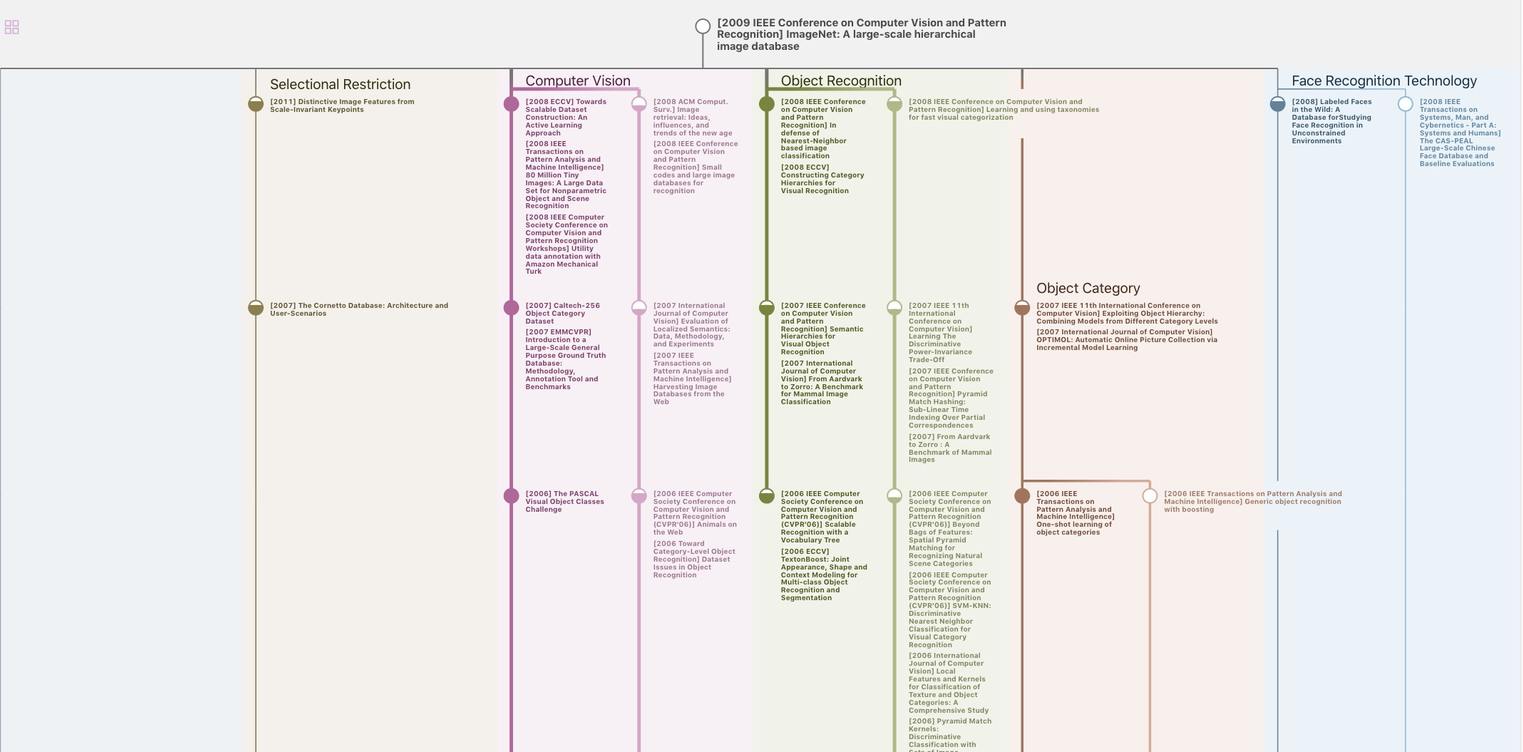
生成溯源树,研究论文发展脉络
Chat Paper
正在生成论文摘要