Predicting Interaction Quality Aspects Using Level-Based Scores for Conversational Agents
PROCEEDINGS OF THE 23RD ACM INTERNATIONAL CONFERENCE ON INTELLIGENT VIRTUAL AGENTS, IVA 2023(2023)
摘要
In order to improve human-agent interaction, it is essential to have good measures of interaction quality. We define interaction quality based on multiple aspects, including usability, likability and perceived conversation quality as subjective measures, and interaction length, completion rate and frequency of unrecognized utterances as objective measures. Determining necessary improvements to a conversational agent is a non-trivial task, because it is difficult to infer from an evaluation of the agent as a whole, which aspects of the agent need to be improved to raise the interaction quality. In this paper, we propose a scoring system for task-oriented conversational agents to predict aspects of interaction quality and to guide an iterative improvement process. Our scoring system does not provide a single score, but leverages structural features of the dialogue management approach and assigns a score on three levels: the utterance, dialogue move, and genre level. Using the agent's scores on separate levels to predict the interaction quality allows making targeted improvements to the conversational agent. In order to evaluate our scoring system, we apply it over the course of multiple crowdsourcing pilot studies, using a recipe recommendation agent. We evaluate the obtained scores in regard to their ability to predict selected objective and subjective interaction quality aspects, as well as their suitability for making informed decisions about necessary improvements.
更多查看译文
AI 理解论文
溯源树
样例
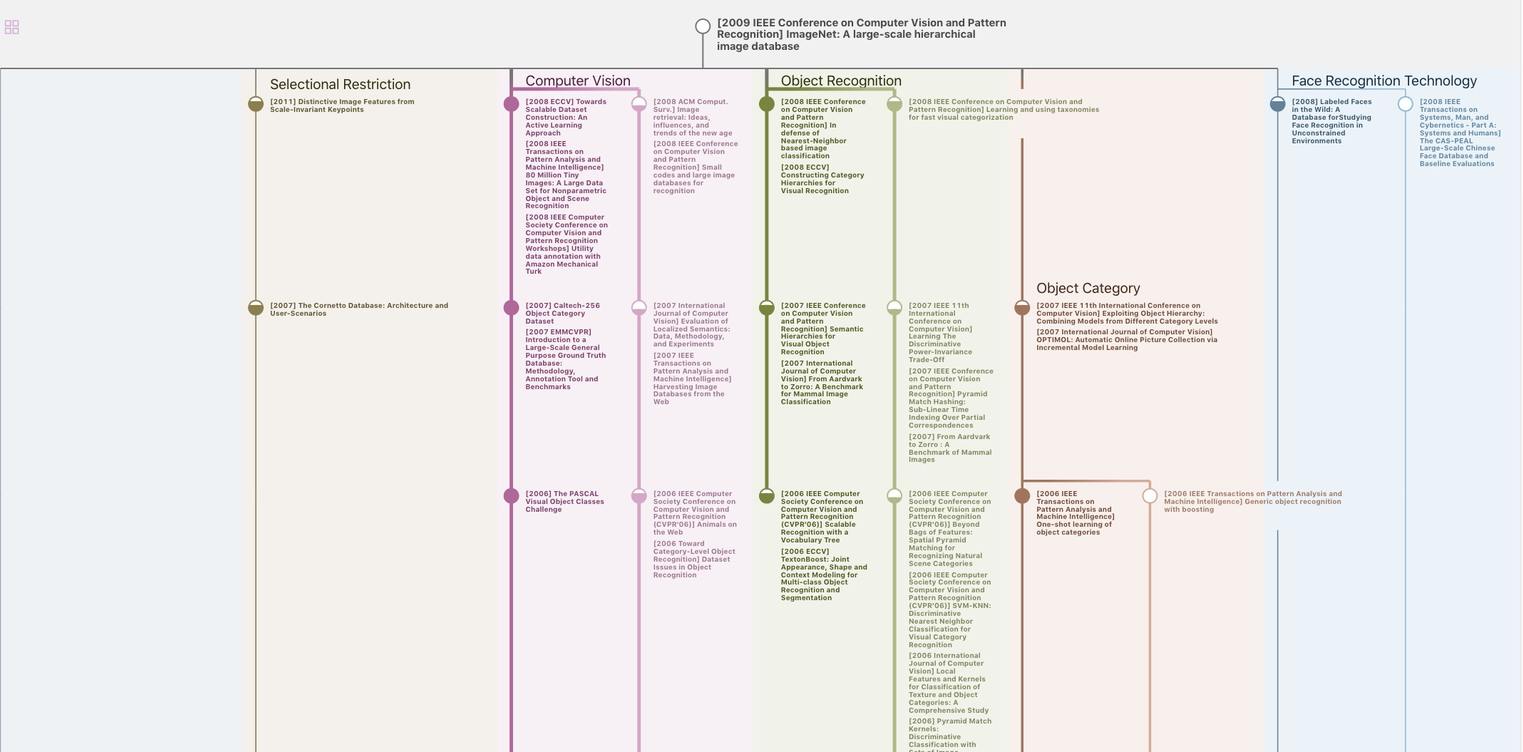
生成溯源树,研究论文发展脉络
Chat Paper
正在生成论文摘要