The Right Prompts for the Job: Repair Code-Review Defects with Large Language Model
CoRR(2023)
摘要
Automatic program repair (APR) techniques have the potential to reduce manual
efforts in uncovering and repairing program defects during the code review (CR)
process. However, the limited accuracy and considerable time costs associated
with existing APR approaches hinder their adoption in industrial practice. One
key factor is the under-utilization of review comments, which provide valuable
insights into defects and potential fixes. Recent advancements in Large
Language Models (LLMs) have enhanced their ability to comprehend natural and
programming languages, enabling them to generate patches based on review
comments. This paper conducts a comprehensive investigation into the effective
utilization of LLMs for repairing CR defects. In this study, various prompts
are designed and compared across mainstream LLMs using two distinct datasets
from human reviewers and automated checkers. Experimental results demonstrate a
remarkable repair rate of 72.97
substantial improvement in the effectiveness and practicality of automatic
repair techniques.
更多查看译文
AI 理解论文
溯源树
样例
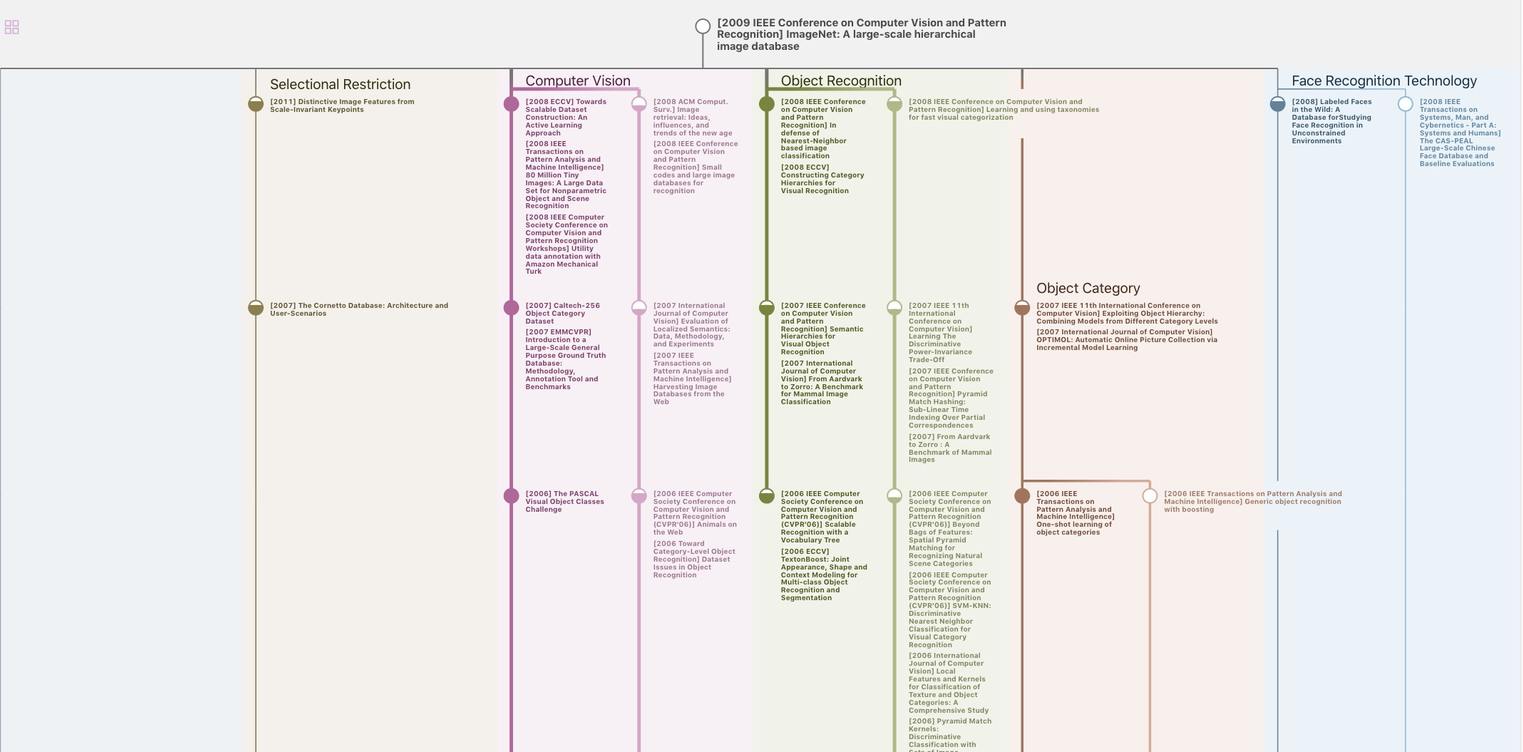
生成溯源树,研究论文发展脉络
Chat Paper
正在生成论文摘要