DeepSecDrive: An explainable deep learning framework for real-time detection of cyberattack in in-vehicle networks
INFORMATION SCIENCES(2024)
摘要
Autonomous driving (AD) technologies are becoming increasingly popular, promising significant enhancements in road safety and efficiency. However, the susceptibility of autonomous vehicles to cyberattacks highlights the critical need for protecting the security and dependability of invehicular networks (IVNs). This study proposes a lightweight, efficient, and explainable deep learning framework, DeepSecDrive, for early detection of security threats against IVN. DeepSecDrive presents robust feature extractor units designed with deformable convolutions and a lightweight non-local network (LNLN). The former promotes dynamic change of receptive field to enhance extraction of complex features from the traffics of in-vehicle networks, while the latter enables learning contextual representations and non-local relationships in vehicular data. DeepSecDrive integrates Shapley Additive exPlanations to construct multi-level interpretability units, providing local and global explanations for model decisions in terms of feature contributions to the overall performance. Proof-of-concept experiments are conducted to evaluate the efficacy and durability of DeepSecDrive on real-world IVN attacks from Car-Hacking dataset, and the experimental results demonstrate the ability of our model to outperform state-of-the-art detection methods.
更多查看译文
关键词
Explainable AI (XAI),In-Vehicular Network,Security,Deep Learning,Autonomous Driving
AI 理解论文
溯源树
样例
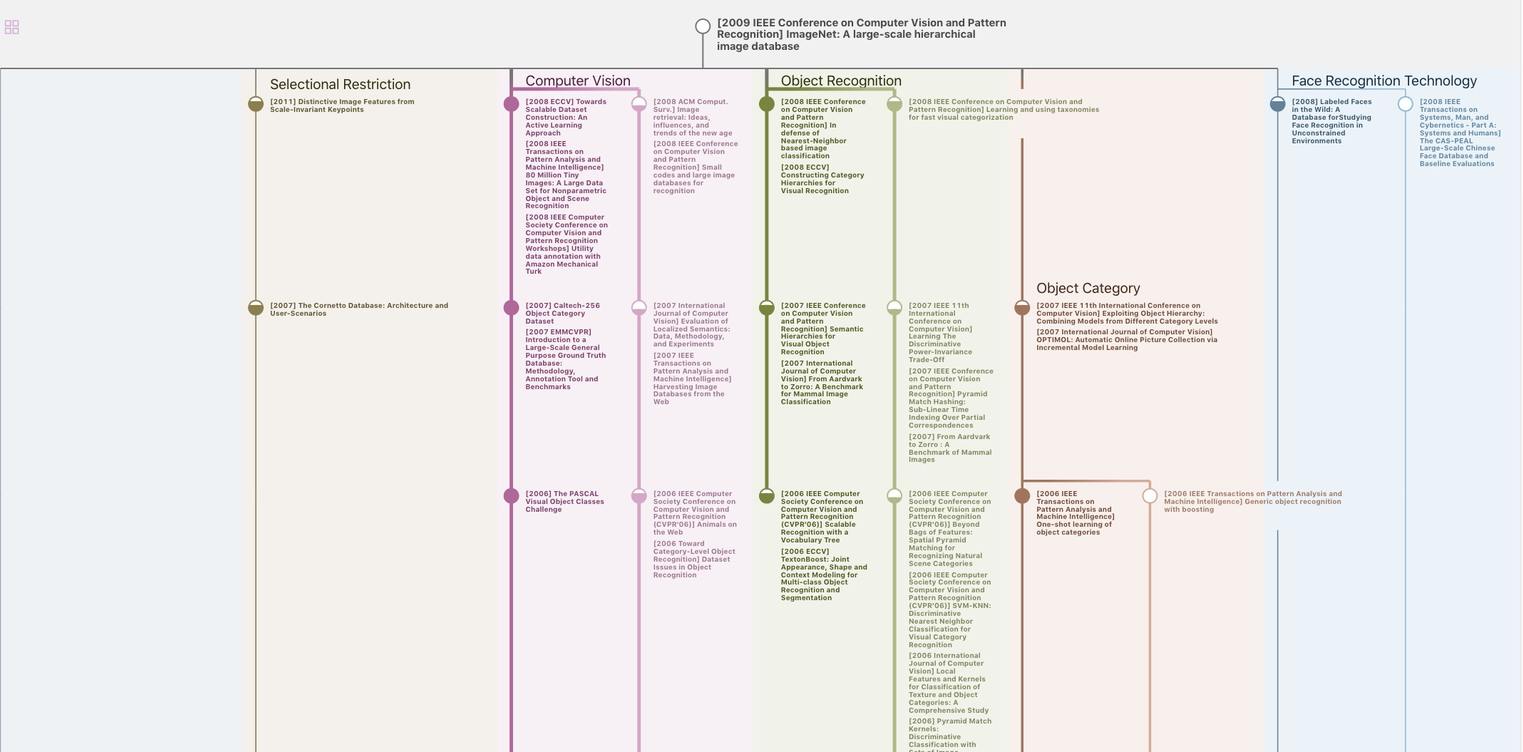
生成溯源树,研究论文发展脉络
Chat Paper
正在生成论文摘要